Full Publication List
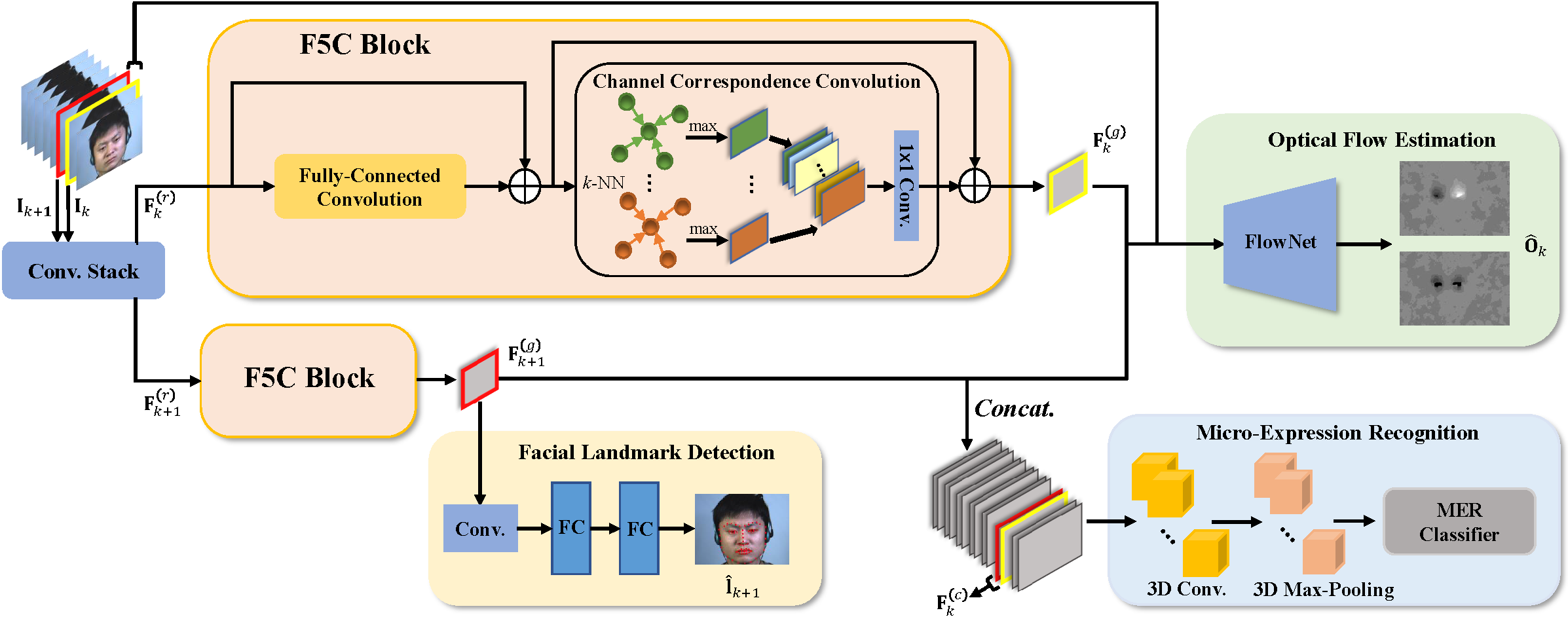
We propose an end-to-end micro-action-aware deep learning framework with advantages from transformer, graph convolution, and vanilla convolution. In particular, we propose a novel F5C block composed of fully-connected convolution and channel correspondence convolution to directly extract local-global features from a sequence of raw frames, without the prior knowledge of key frames. The transformer-style fully-connected convolution is proposed to extract local features while maintaining global receptive fields, and the graph-style channel correspondence convolution is introduced to model the correlations among feature patterns. Moreover, MER, optical flow estimation, and facial landmark detection are jointly trained by sharing the local-global features. The two latter tasks contribute to capturing facial subtle action information for MER, which can alleviate the impact of insufficient training data.
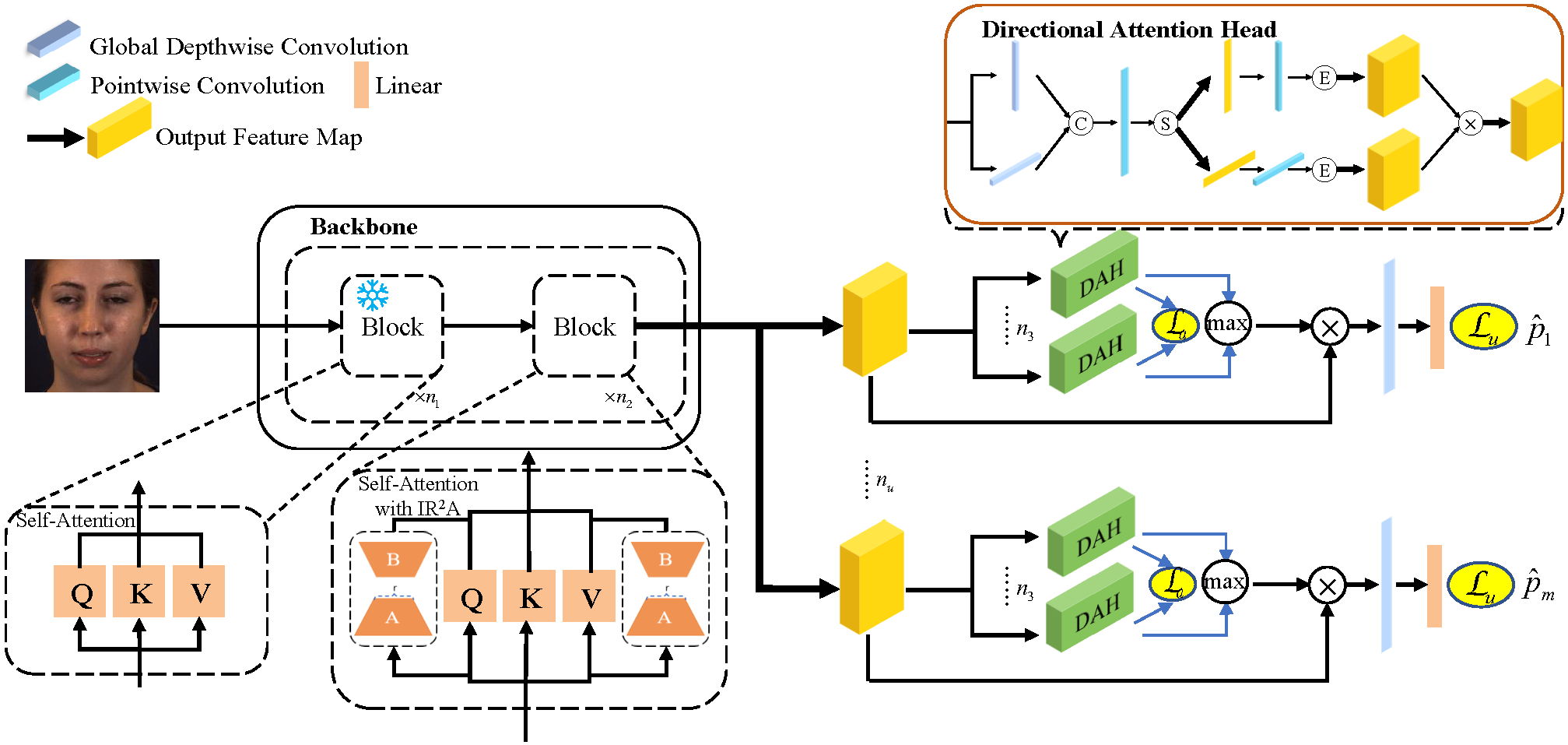
We propose a new iterative rank reduction adapter (IR2A) to fine-tune a VFM for AU detection. In particular, we freeze the pre-trained model parameters and introduce trainable rank decomposition matrices to top self-attention blocks. We set an initial rank for rank decomposition matrices, and then iteratively reduce the rank via principal component analysis. Moreover, we propose a directional attention to learn relevant features to each AU, in which important information in different directions are captured.
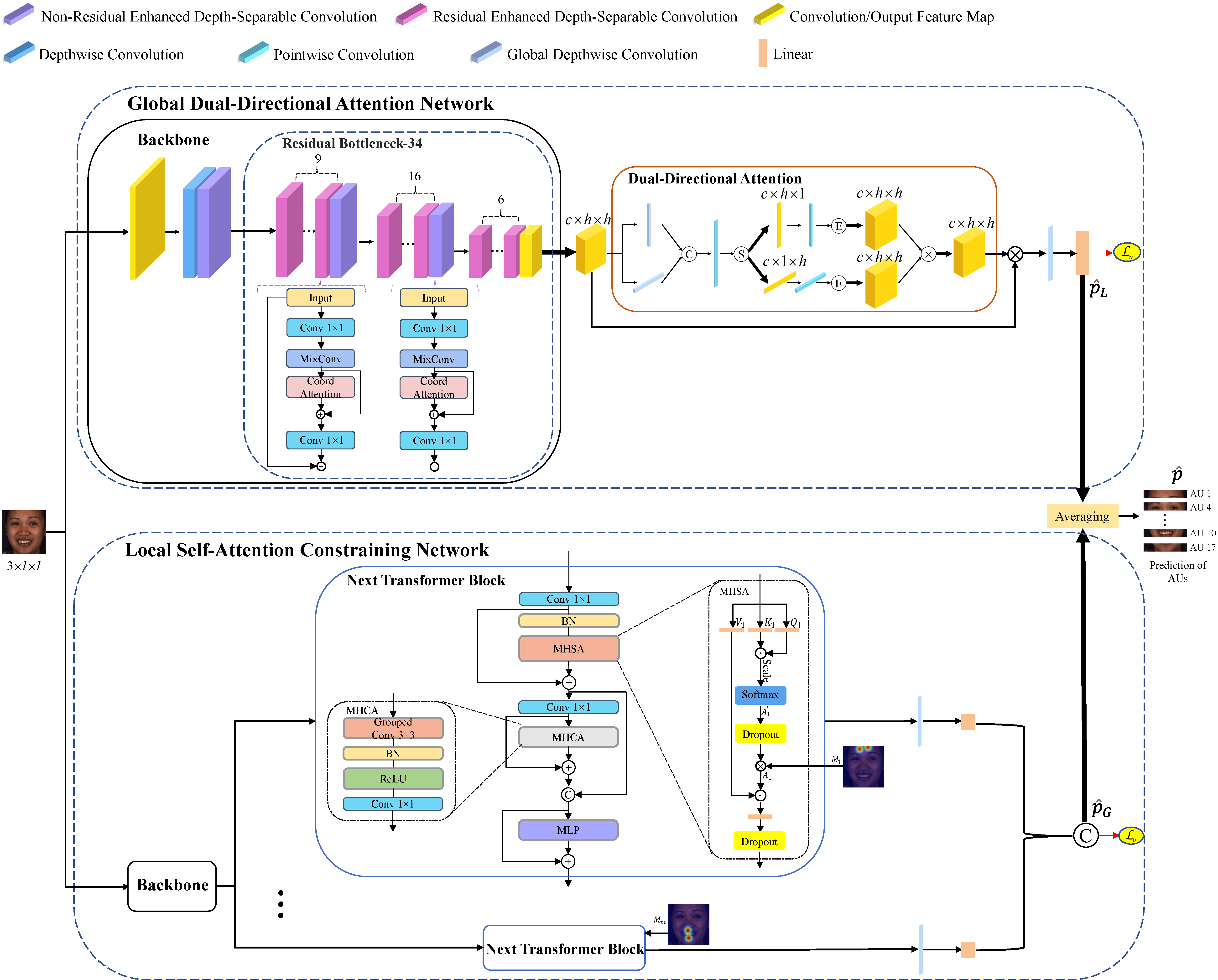
We propose a local self-attention constraining (LSC) network, by regarding the self-attention distribution of each AU as a spatial distribution, and constraining it based on prior knowledge so as to capture AU-related local information. Moreover, to learn correlations among different AU regions, we propose a global dual-directional attention (GDA) network, which adaptively learns global attention map from both vertical and horizontal directions. Last but not least, the two networks from different views of capturing patterns are assembled to integrate both advantages.
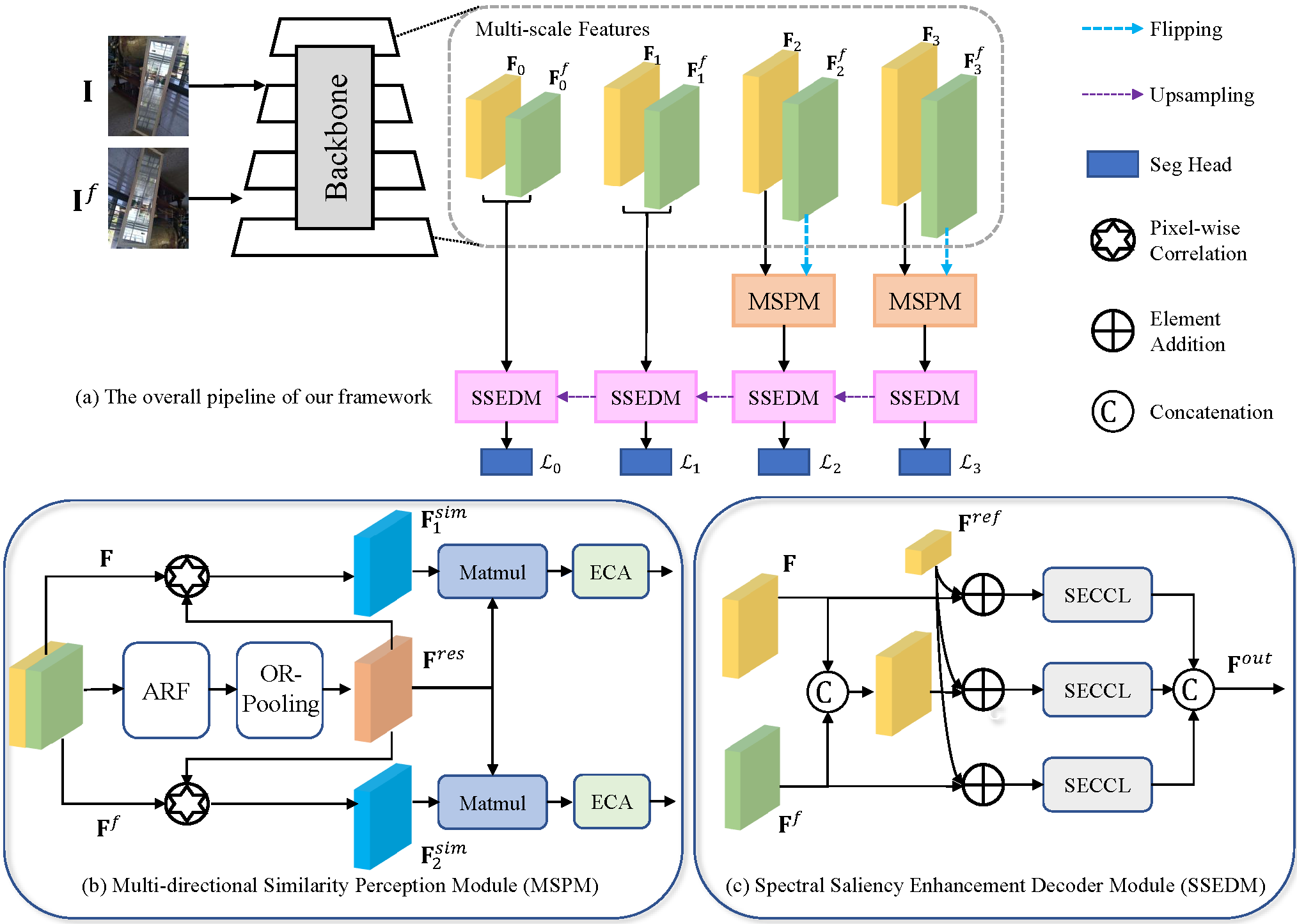
We propose a novel mirror detection framework called S2MD including two main modules, multi-directional similarity perception module (MSPM) and spectral saliency enhancement decoder module (SSEDM). Specifically, we employ a backbone network to extract multi-scale global information from images using a dual-path approach. Then, we feed these high-level dual-path features into MSPMs to generate direction-sensitive similarity-consistent features. MSPM utilizes active rotating filters and oriented response pooling to model the similarity relations in different orientations. Moreover, the SSEDM is utilized to enhance the spatial contextual contrasted features using feature spectral residuals and fuse the dual-path features to obtain the final predicted mirror mask.
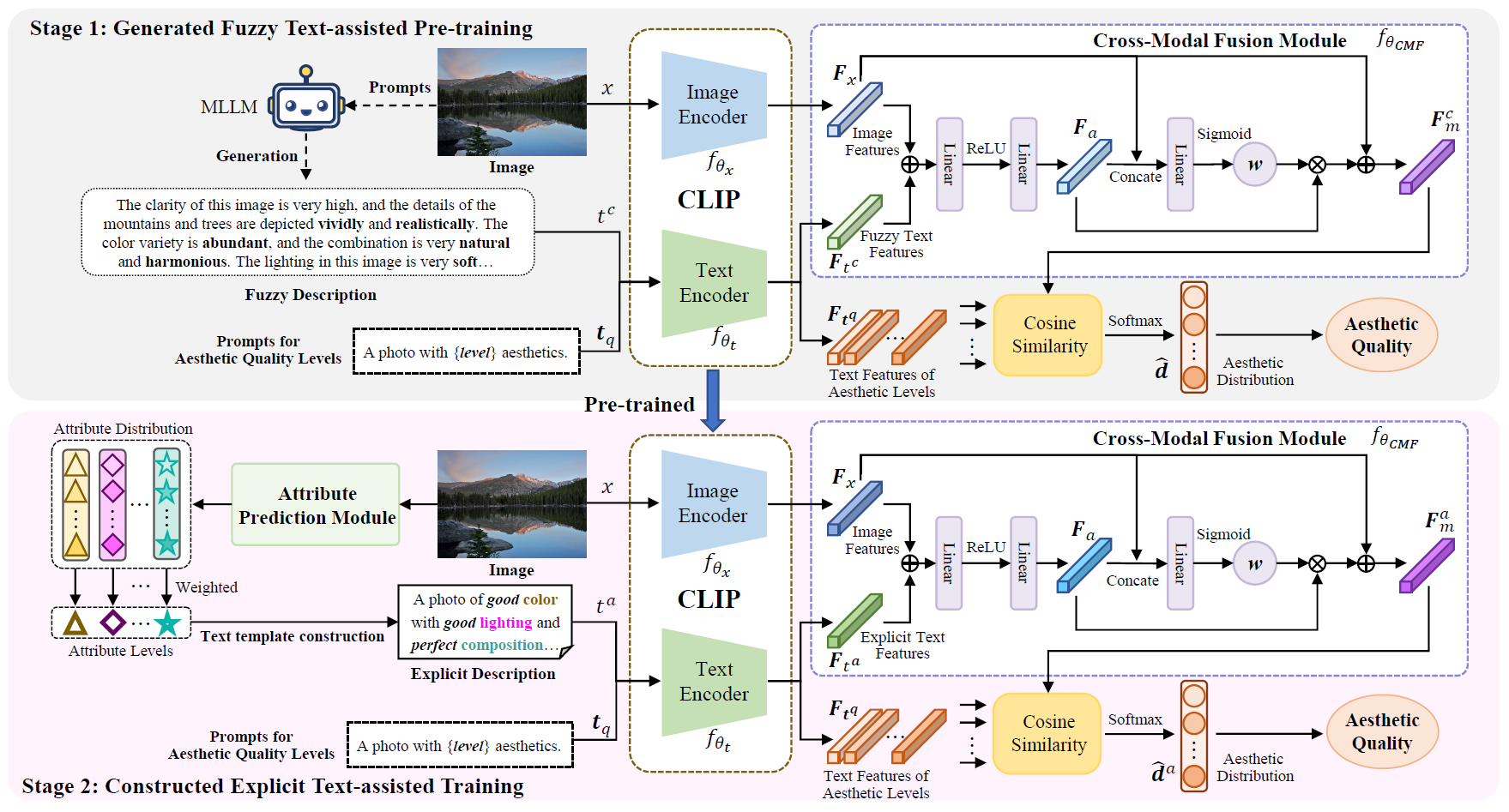
This paper proposes a progressively generated text-assisted image aesthetic quality assessment method, aiming to address the lack of aesthetic comments and the fuzziness of aesthetic judgments in these comments. Specifically, we first adopt a Multimodal Large Language Model (MLLM) to generate aesthetic comments on images by simulating user perceptions and utilize the generated comments to characterize their aesthetic perception to assist in the pretraining of our multimodal-based IAQA model. Then, we design an attribute prediction module to determine the attribute levels of aesthetic judgments and utilize text template construction to further generate explicit descriptions of image aesthetics. Finally, we leverage the generated attribute descriptions to further assist in training our IAQA model. By progressively generating textual auxiliary descriptions of aesthetics for images, the proposed model can gradually determine the aesthetic quality of the images.
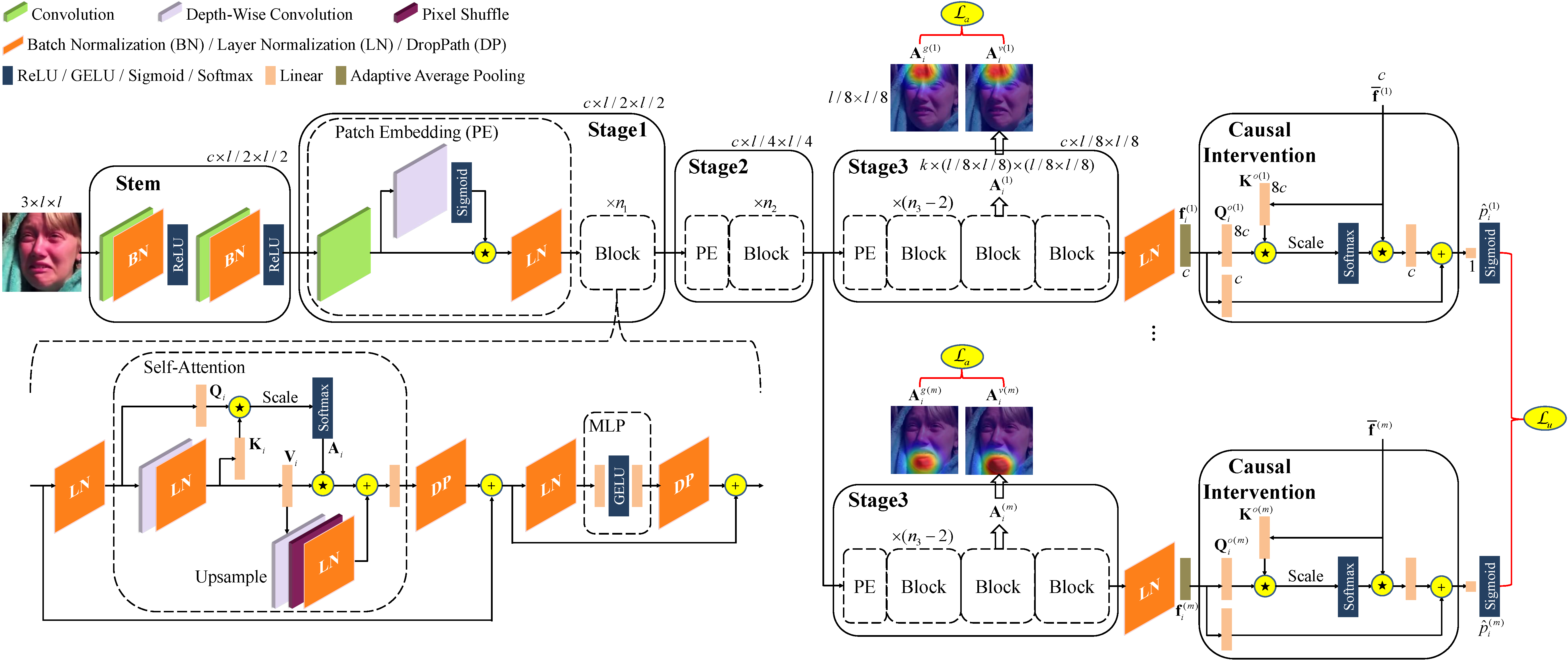
We propose a novel AU detection framework called AC2D by adaptively constraining self-attention weight distribution and causally deconfounding the sample confounder. Specifically, we explore the mechanism of self-attention weight distribution, in which the self-attention weight distribution of each AU is regarded as spatial distribution and is adaptively learned under the constraint of location-predefined attention and the guidance of AU detection. Moreover, we propose a causal intervention module for each AU, in which the bias caused by training samples and the interference from irrelevant AUs are both suppressed.
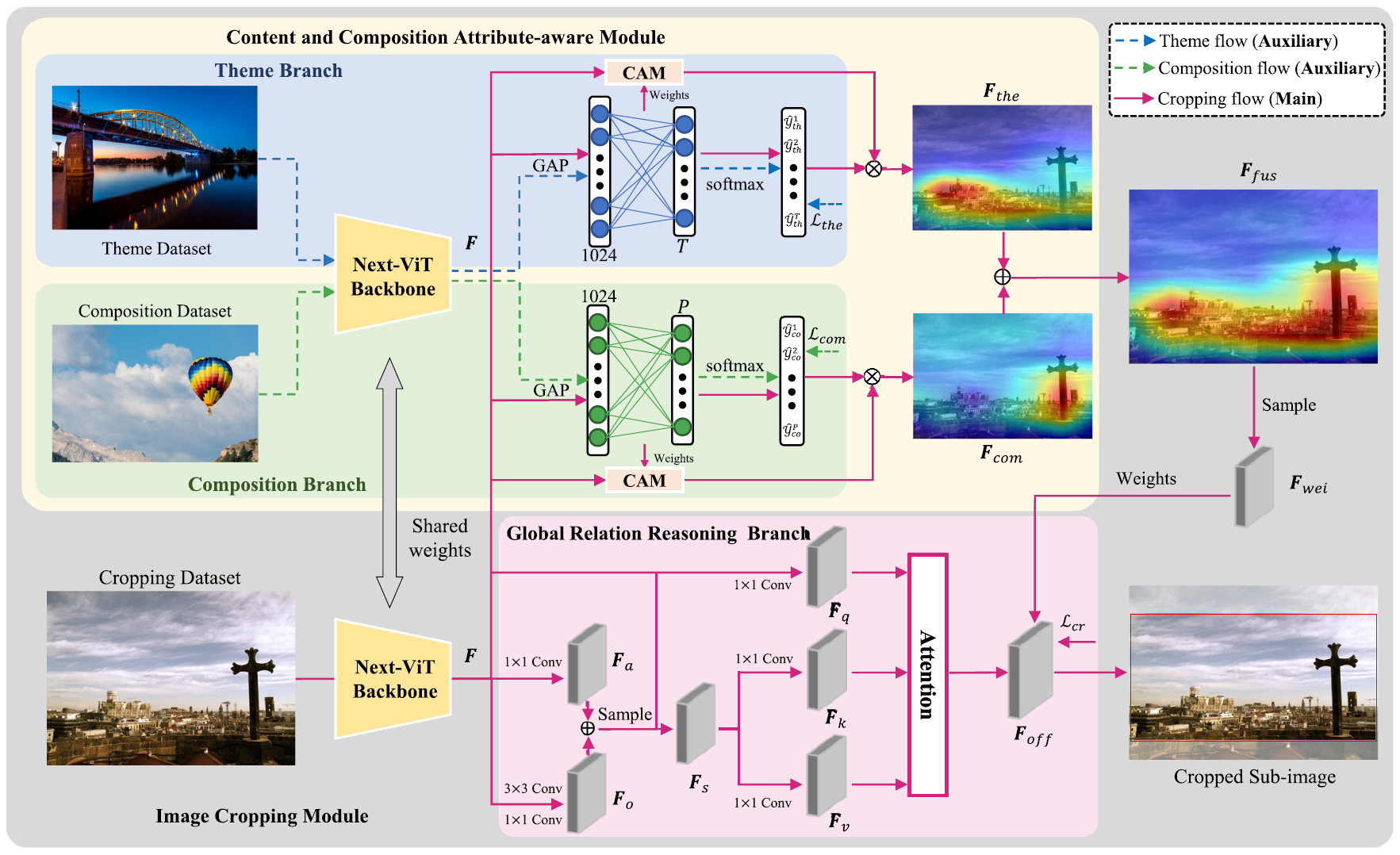
To comprehensively introduce aesthetic information into image cropping, we capture feature representations reinforced by content and composition attributes simultaneously. The feature representations can strengthen the visual aesthetics of cropped sub-images. To make the cropped sub-images amply contain more global information, we introduce a global relation reasoning branch in the proposed cropping module, which can fully exploit the dependency relationship between the foreground and background in images.
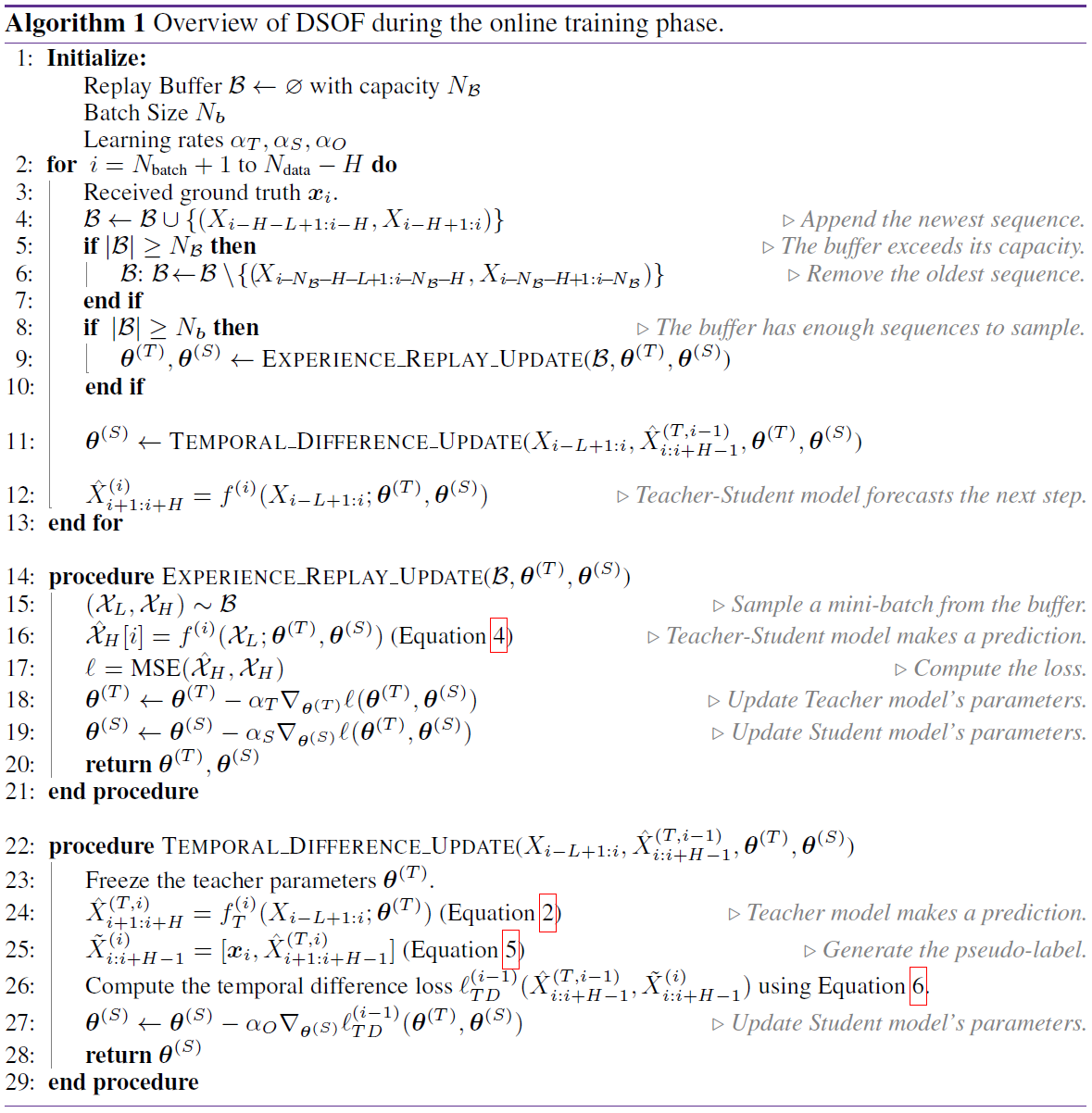
This paper redefines online time series forecasting to focus on predicting unknown future steps and evaluates performance solely based on these predictions. Following this new setting, challenges arise in leveraging incomplete pairs of ground truth and prediction for backpropagation, as well as generalizing accurate information without overfitting to noises from recent data streams. To address these challenges, we propose a novel dual-stream framework for online forecasting (DSOF): a slow stream that updates with complete data using experience replay, and a fast stream that adapts to recent data through temporal difference learning. This dual-stream approach updates a teacher-student model learned through a residual learning strategy, generating predictions in a coarse-to-fine manner.
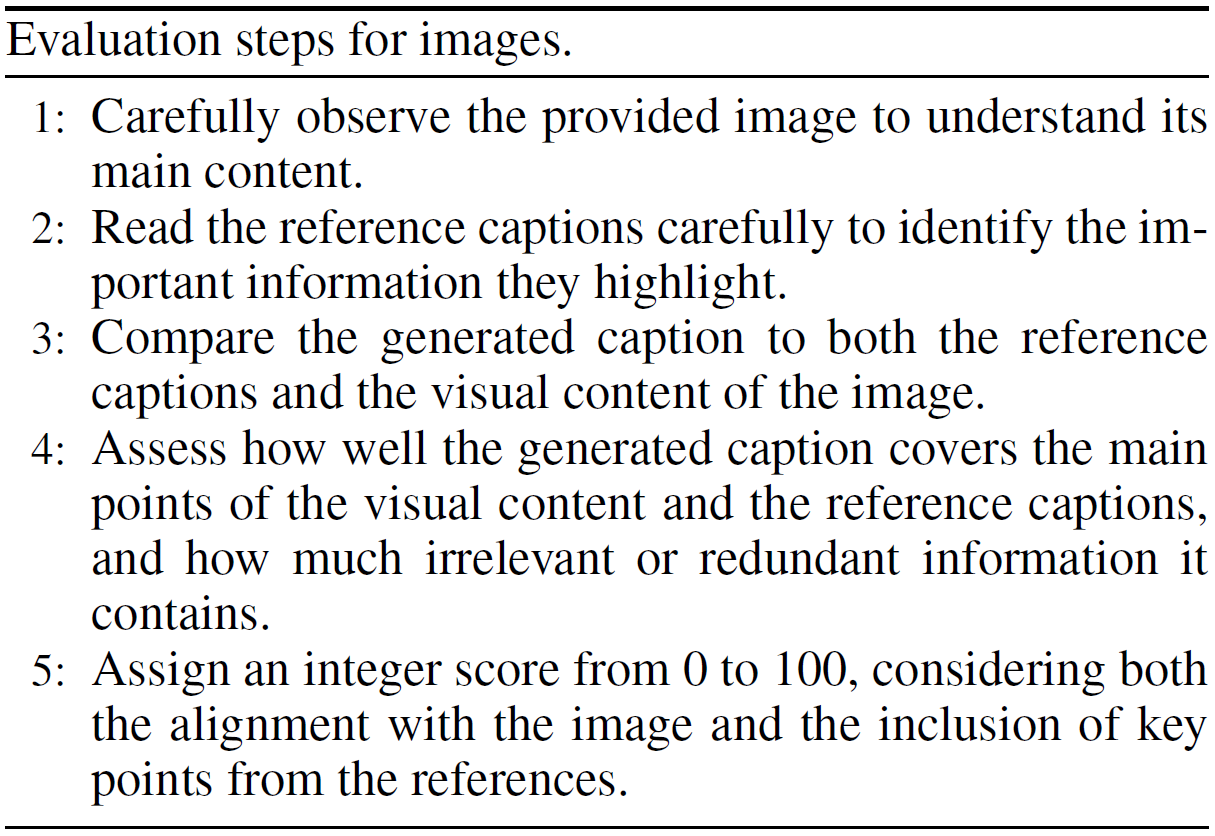
We introduce G-VEval, a novel metric inspired by G-Eval and powered by the new GPT-4o. G-VEval uses chain-of-thought reasoning in large multimodal models and supports three modes: reference-free, reference-only, and combined, accommodating both video and image inputs. We also propose MSVD-Eval, a new dataset for video captioning evaluation, to establish a more transparent and consistent framework for both human experts and evaluation metrics. It is designed to address the lack of clear criteria in existing datasets by introducing distinct dimensions of Accuracy, Completeness, Conciseness, and Relevance (ACCR).
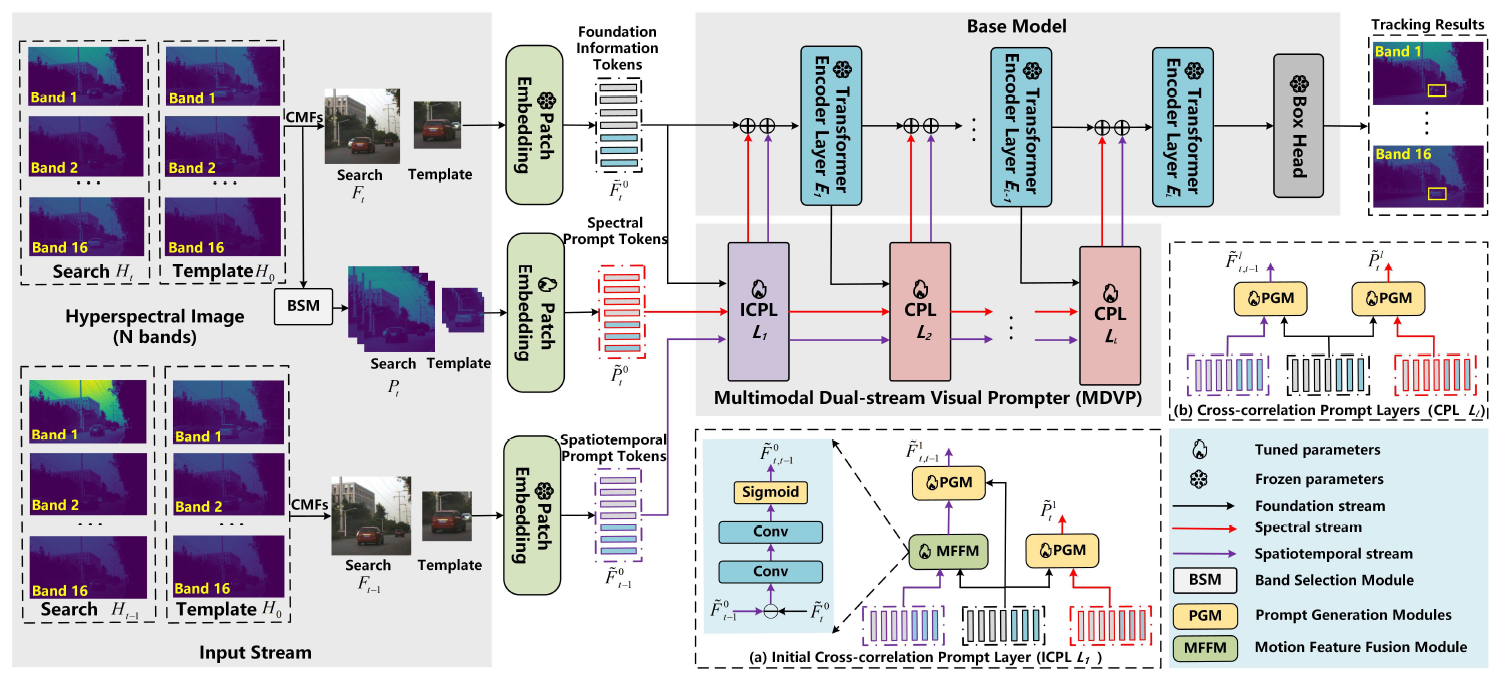
We propose a unified spectral-spatiotemporal multimodal dual-stream prompt hyperspectral object tracking, named HDSP. We design a density clustering-based band selection module to preserve spectral prompt information efficiently. Using the generated bands and temporal data as multimodal prompts, a dual-stream visual prompter is proposed. Designed Multimodal Dual-stream Visual Prompter (MDVP) transform the multimodal input into a single modality, enhancing the foundational modality’s representation capabilities for hyperspectral tracking.
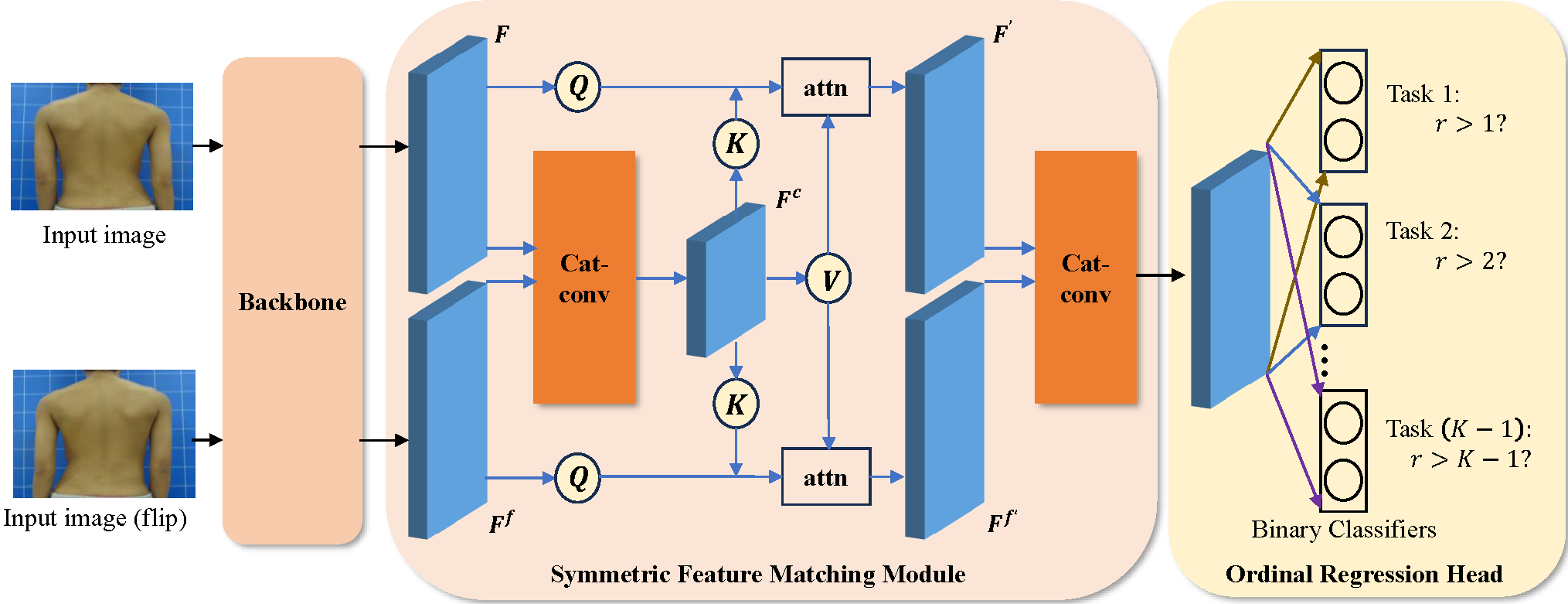
We notice that the human back has a certain degree of symmetry, and asymmetrical human backs are usually caused by spinal lesions. Besides, scoliosis severity levels have ordinal relationships. Taking inspiration from this, we propose a dual-path scoliosis detection network with two main modules: symmetric feature matching module (SFMM) and ordinal regression head (ORH). Specifically, we first adopt a backbone to extract features from both the input image and its horizontally flipped image. Then, we feed the two extracted features into the SFMM to capture symmetric relationships. Finally, we use the ORH to transform the ordinal regression problem into a series of binary classification sub-problems.
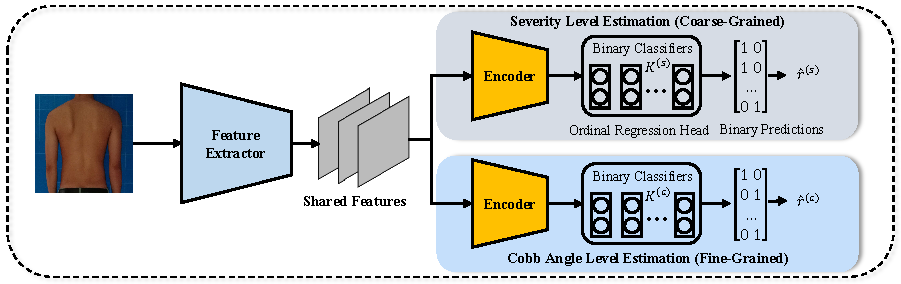
We propose a multi-grained scoliosis detection framework by jointly estimating severity level and Cobb angle level of scoliosis from a natural image instead of a radiographic image, which has not been explored before. Specifically, we regard scoliosis estimation as an ordinal regression problem, and transform it into a series of binary classification sub-problems. Besides, we adopt the visual attention network with large kernel attention as the backbone for feature learning, which can model local and global correlations with efficient computations. The feature learning and the ordinal regression is put into an end-to-end framework, in which the two tasks of scoliosis severity level estimation and scoliosis angle level estimation are jointly learned and can contribute to each other.
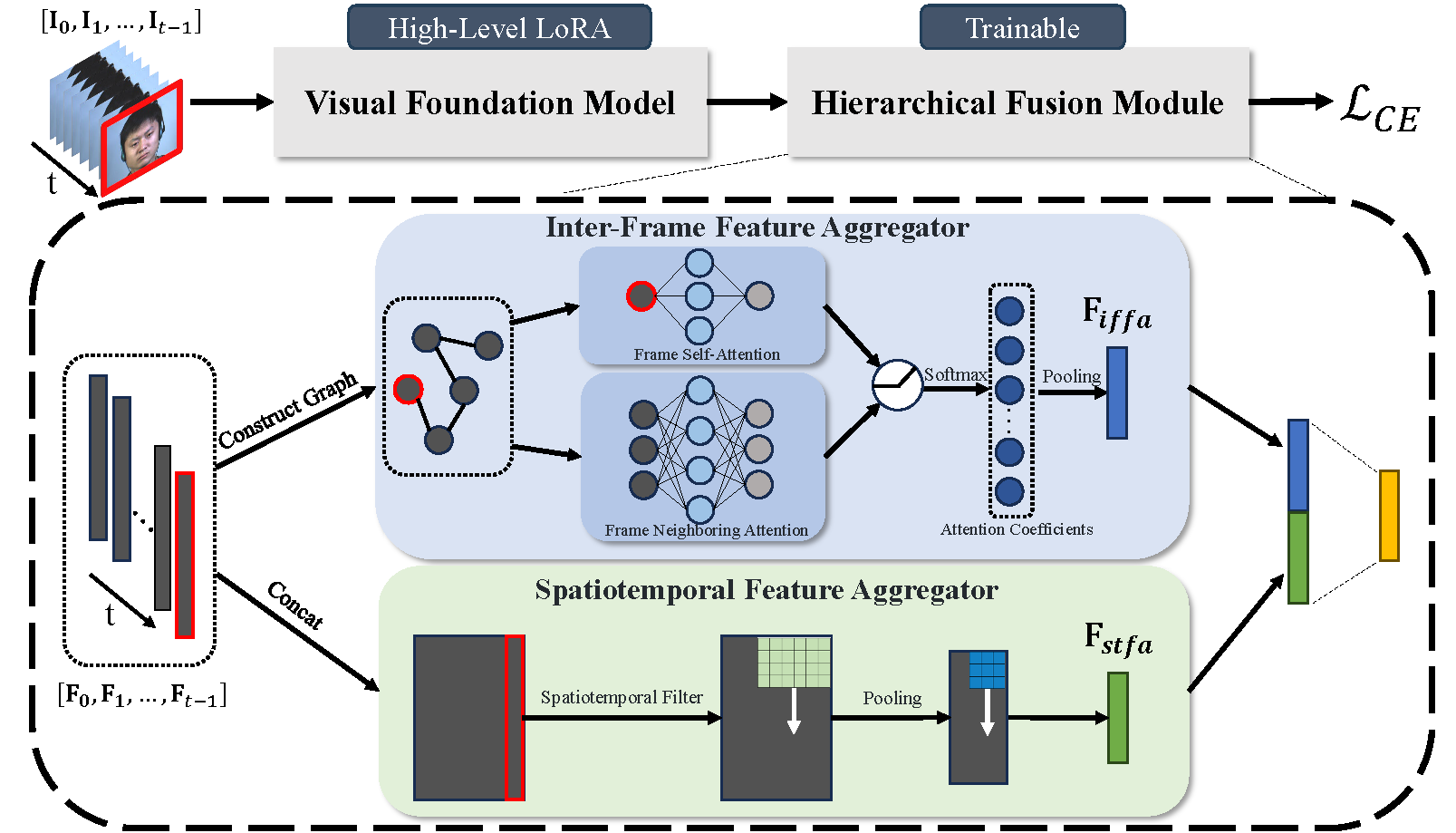
We propose HLoRA-MER, a novel framework that combines high-level low-rank adaptation (HLoRA) and a hierarchical fusion module (HFM). HLoRA fine-tunes the high-level layers of a VFM to capture facial muscle movement information, while HFM aggregates inter-frame and spatio-temporal features.
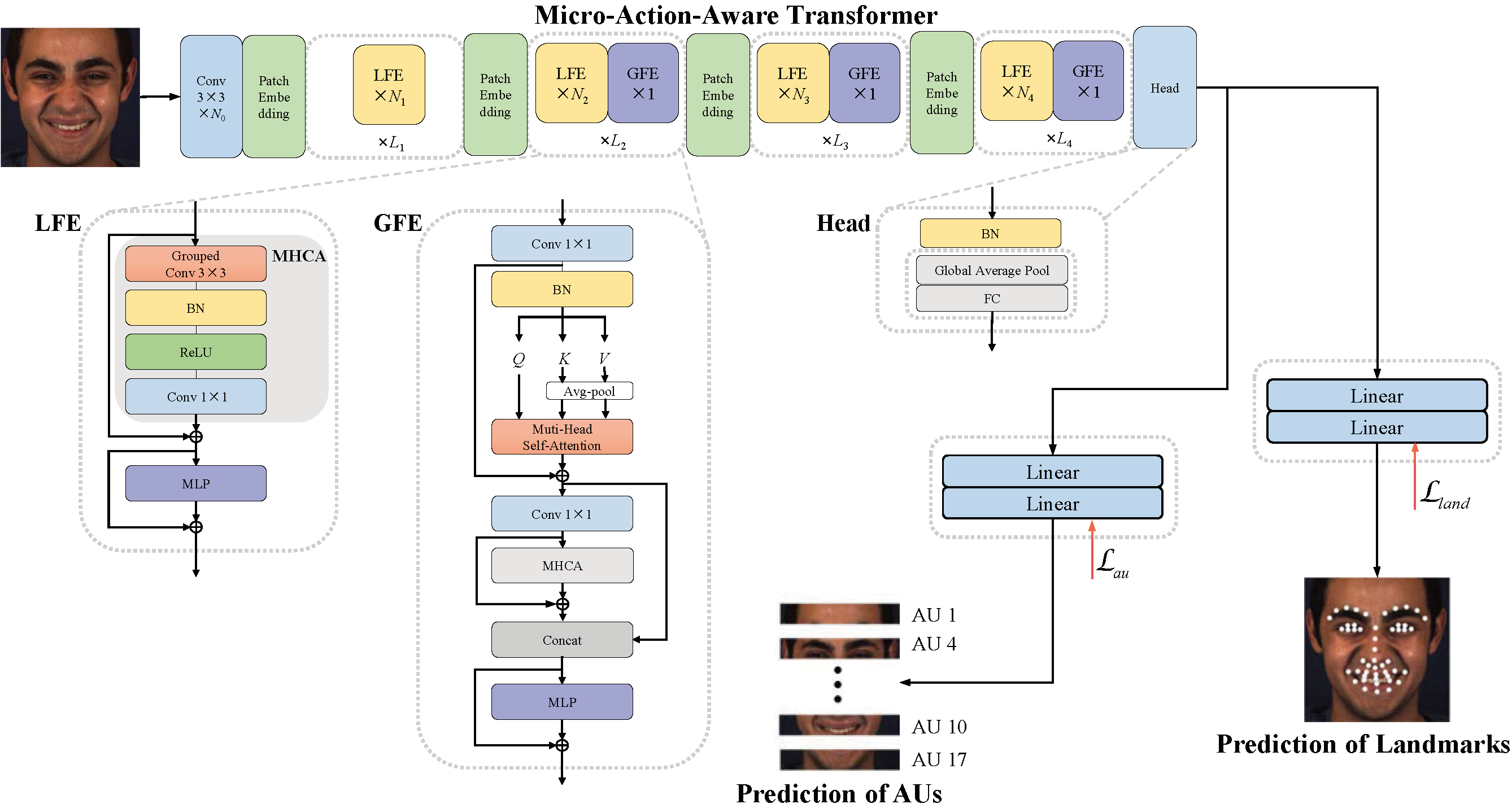
We propose a novel micro-action-aware transformer to integrate local and global feature extractions, which effectively captures subtle AU details while maintaining the global relational modeling capacity of transformers. Besides, we jointly train facial AU recognition and facial landmark detection, in which the two correlated tasks contribute to each other and further facilitate the learning of local-global AU-related feature.
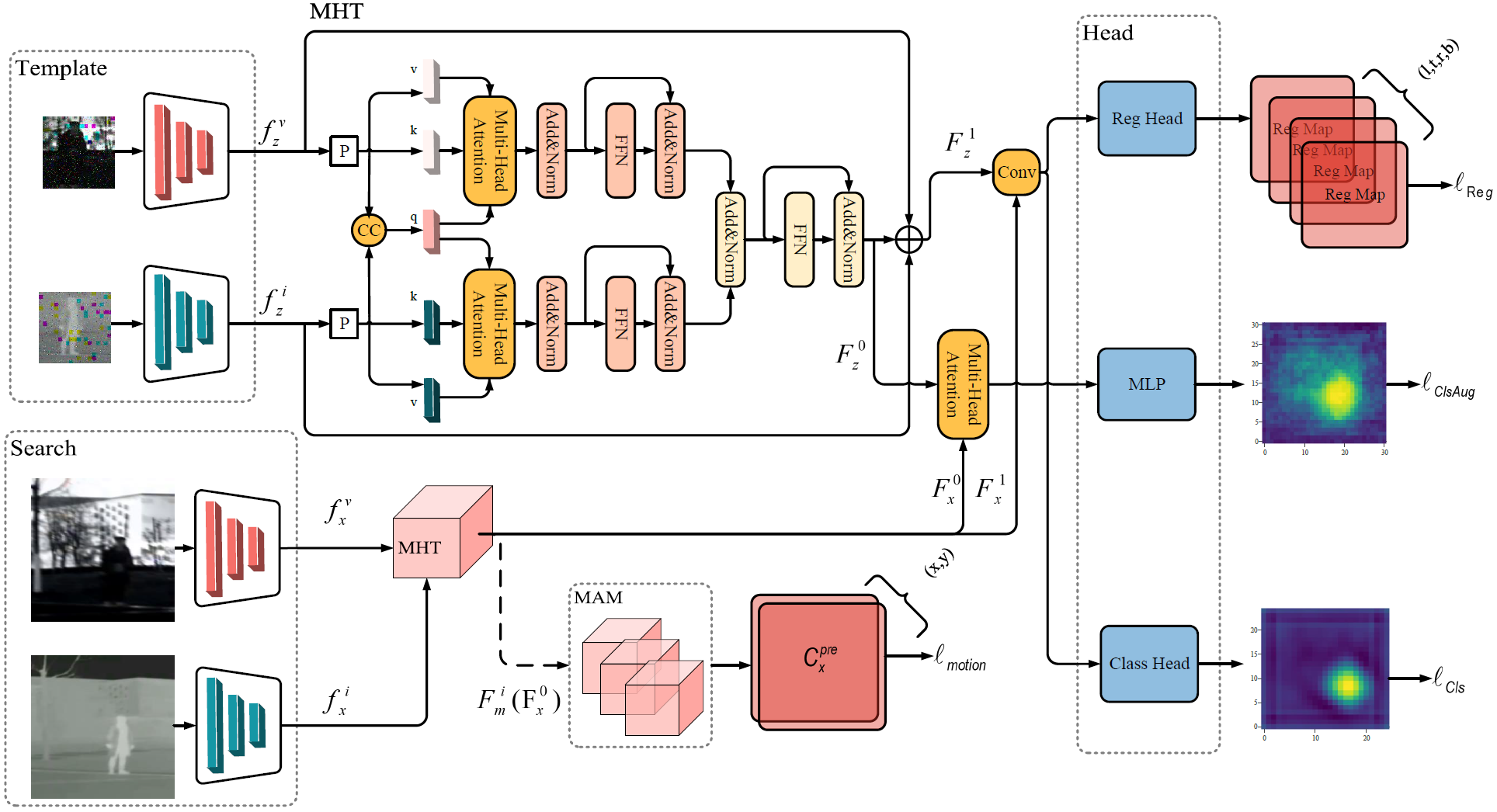
We propose a self-supervised RGBT object tracking method (S2OTFormer) to bridge the gap between tracking methods supervised under pseudo-labels and ground truth labels. Firstly, to provide more robust appearance features for motion cues, we introduce a Multi-Modal Hierarchical Transformer module (MHT) for feature fusion. This module allocates weights to both modalities and strengthens the expressive capability of the MHT module through multiple nonlinear layers to fully utilize the complementary information of the two modalities. Secondly, in order to solve the problems of motion blur caused by camera motion and inaccurate appearance information caused by pseudo-labels, we introduce a Motion-Aware Mechanism (MAM). The MAM extracts the average motion vectors from the previous multi-frame search frame features and constructs the consistency loss with the motion vectors of the current search frame features. The motion vectors of inter-frame objects are obtained by reusing the inter-frame attention map to predict coordinate positions. Finally, to further reduce the effect of inaccurate pseudo-labels, we propose an Attention-Based Multi-Scale Enhancement Module. By introducing cross-attention to achieve more precise and accurate object tracking, this module overcomes the receptive field limitations of traditional CNN tracking heads.
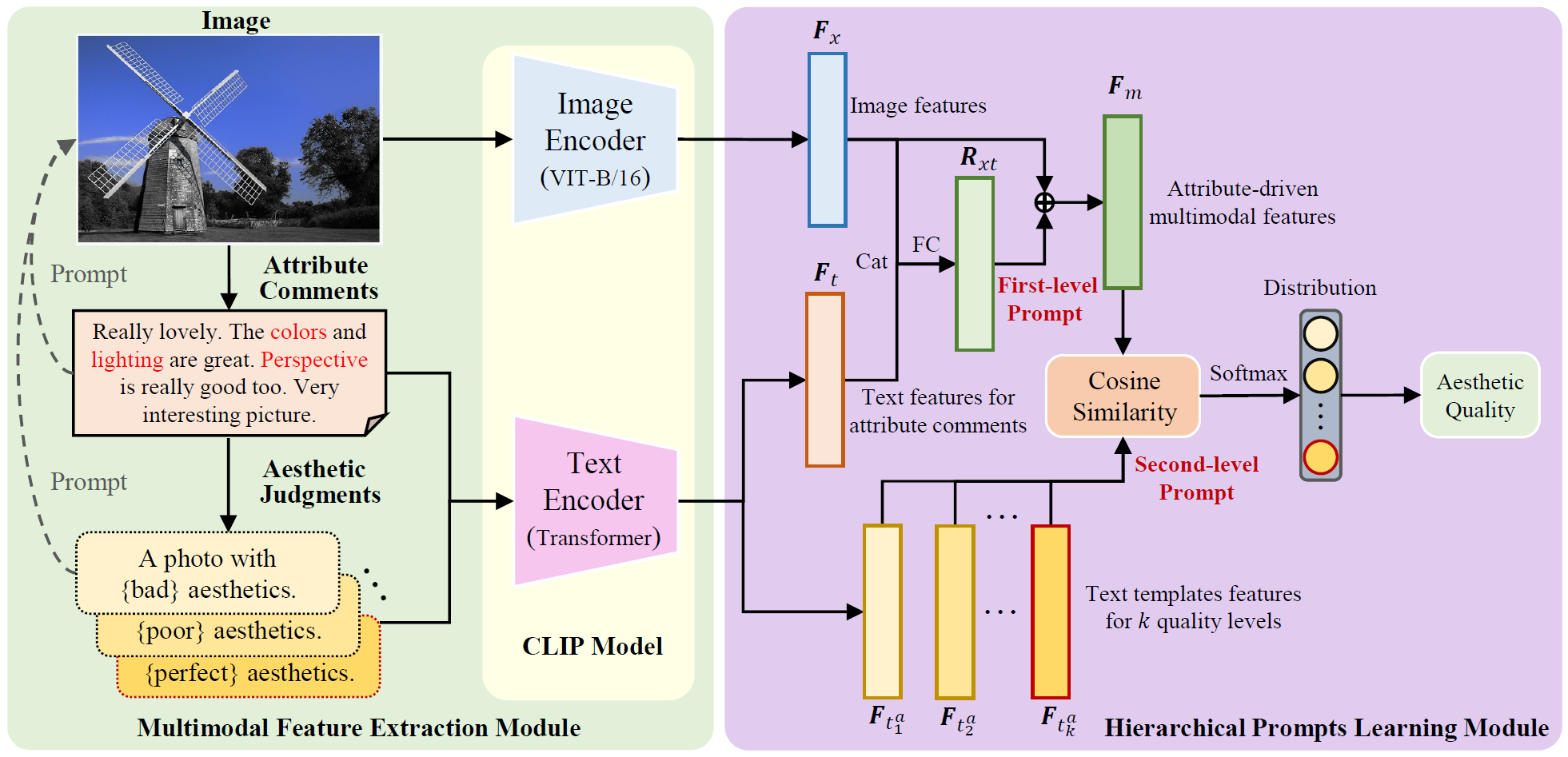
This paper proposes an image aesthetic quality assessment method based on attribute-driven multimodal hierarchical prompts. Unlike existing IAQA methods that utilize multimodal pre-training or straightforward prompts for model learning, the proposed method leverages attribute comments and quality-level text templates to hierarchically learn the aesthetic attributes and quality of images. Specifically, we first leverage aesthetic attribute comments to perform prompt learning on images. The learned attribute-driven multimodal features can comprehensively capture the semantic information of image aesthetic attributes perceived by users. Then, we construct text templates for different aesthetic quality levels to further facilitate prompt learning through semantic information related to the aesthetic quality of images. The proposed method can explicitly simulate aesthetic judgment of images to obtain more precise aesthetic quality.
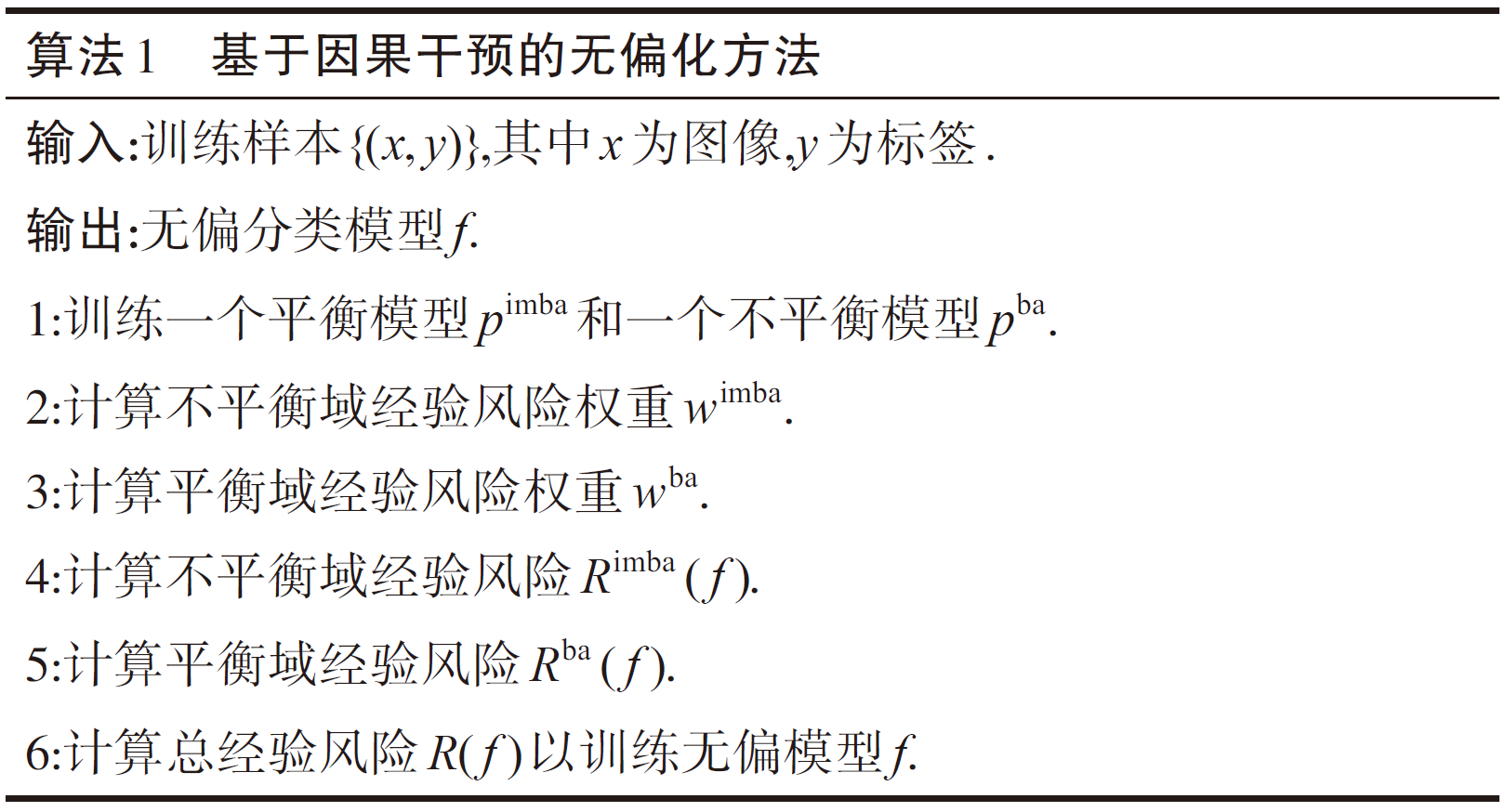
By introducing causal inference theory, we propose an unbiased AU recognition method CIU (Causal Intervention for Unbiased facial action unit recognition), which adjusts the empirical risks in both the imbalanced and balanced but invisible domains to achieve model unbiasedness.
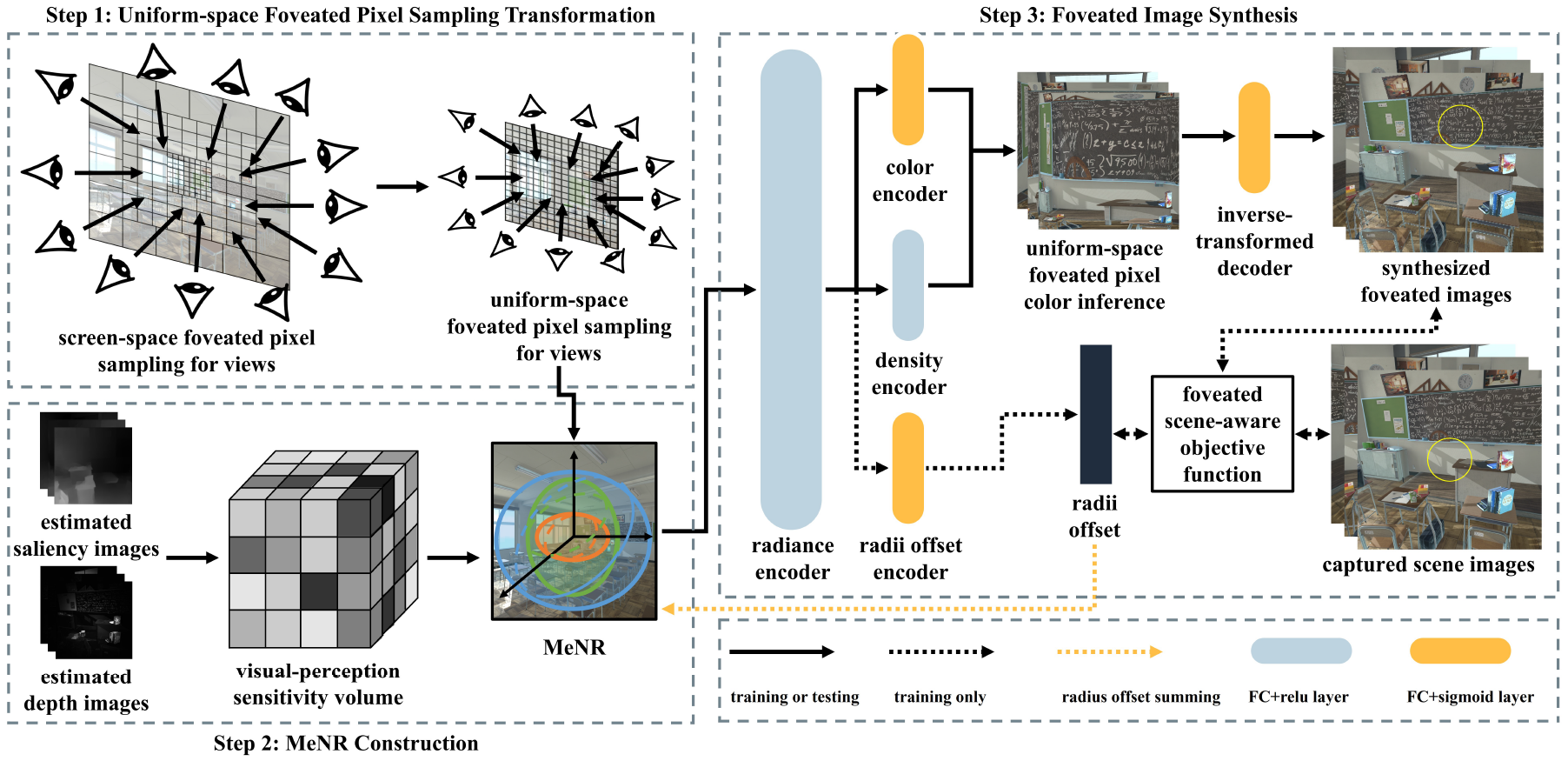
In this paper, we propose a scene-aware foveated neural radiance fields method to synthesize high-quality foveated images in complex VR scenes at high frame rates. Firstly, we construct a multi-ellipsoidal neural representation to enhance the neural radiance fields representation capability in salient regions of complex VR scenes based on the scene content. Then, we introduce a uniform sampling based foveated neural radiance fields framework to improve the foveated image synthesis performance with one-pass color inference, and improve the synthesis quality by leveraging the foveated scene-aware objective function.
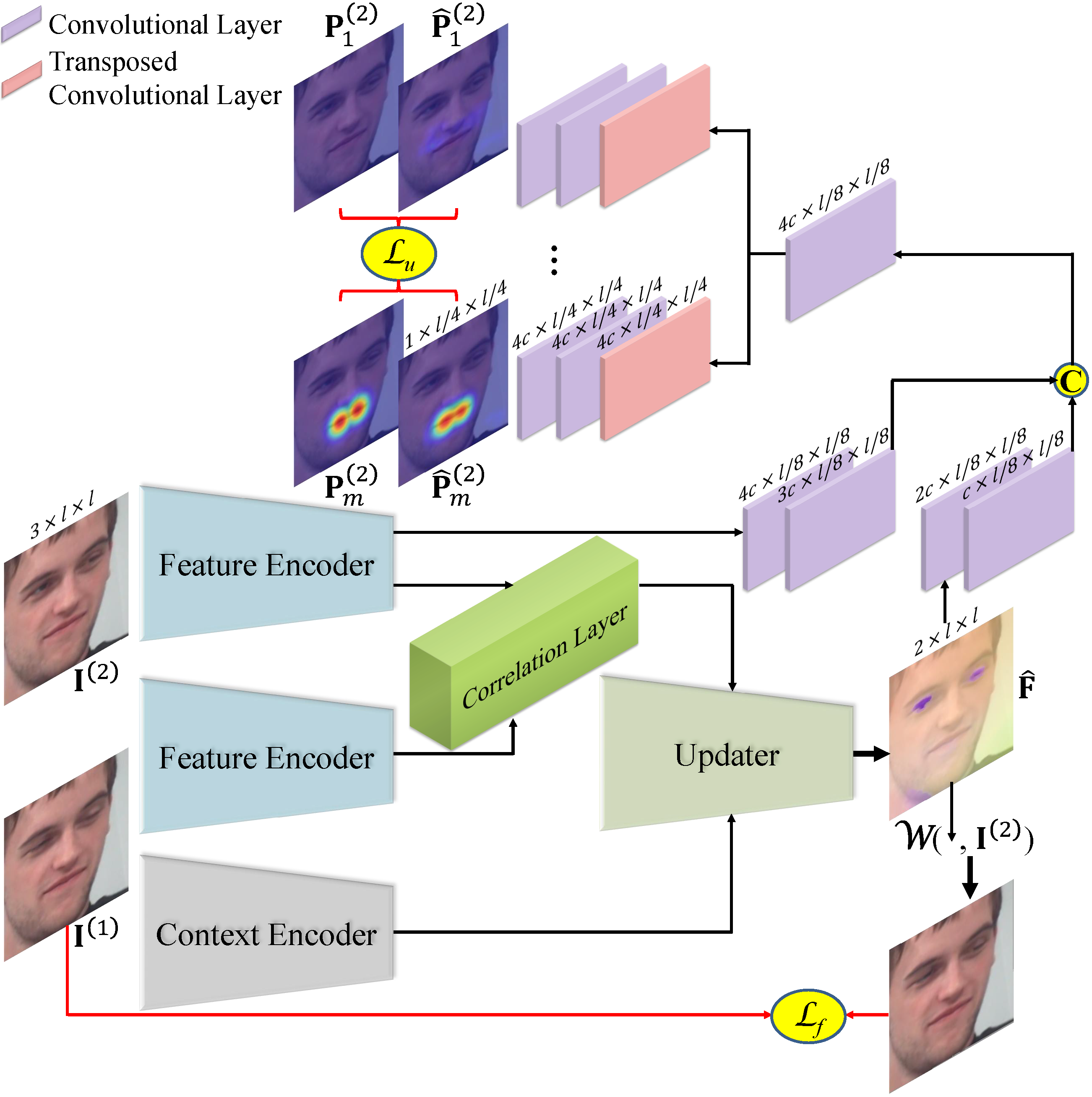
We propose a novel end-to-end joint framework of AU recognition and optical flow estimation, in which the two tasks contribute to each other. Moreover, due to the lack of optical flow annotations in AU datasets, we propose to estimate optical flow in a self-supervised manner. To regularize the self-supervised estimation of optical flow, we propose an identical mapping constraint for the optical flow guided image warping process, in which the estimated optical flow between two same images is required to not change the image during warping.
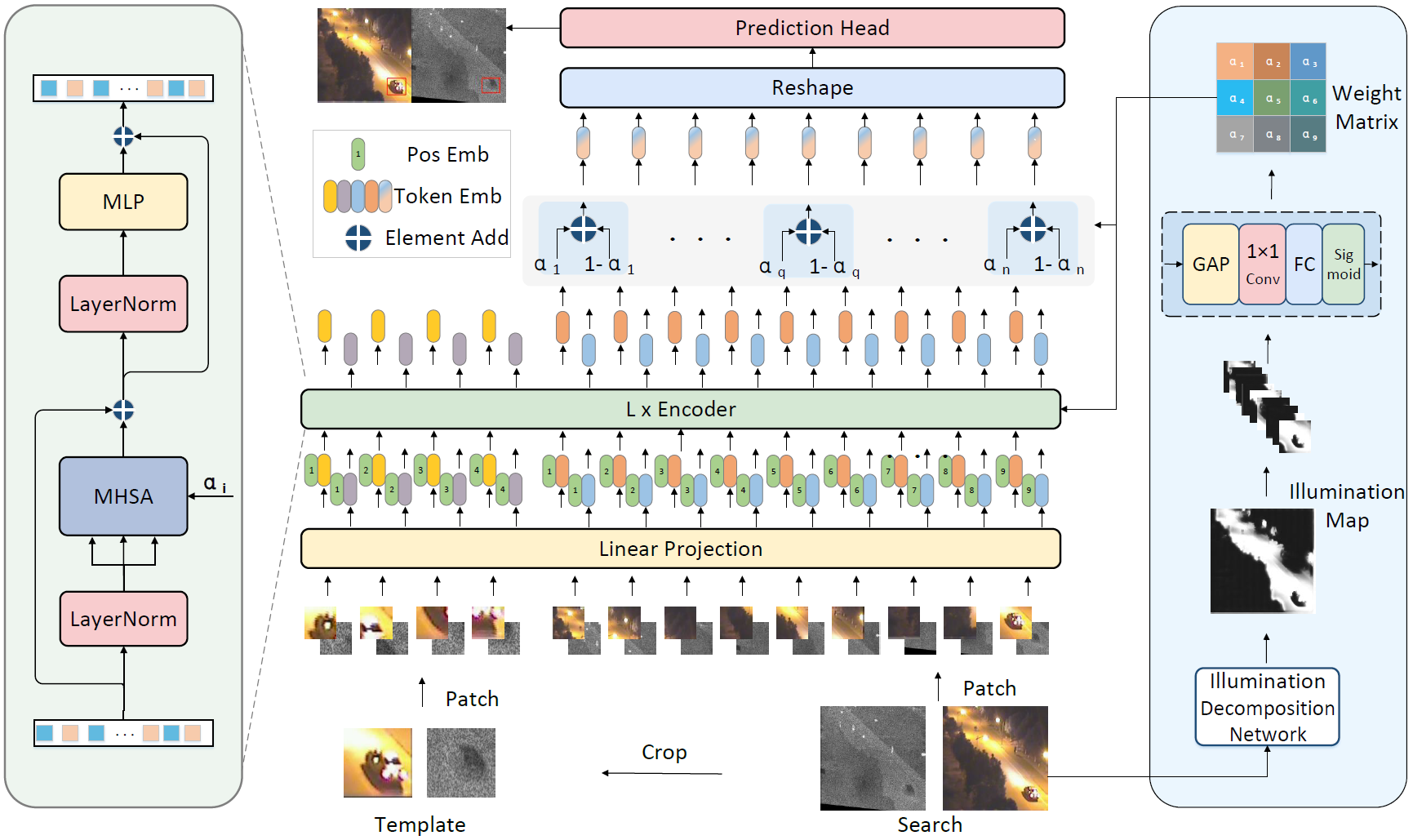
We address these two critical issues by introducing a novel RGBT tracking framework centered on multimodal hierarchical relationship modeling. Through the incorporation of multiple Transformer encoders and the deployment of self-attention mechanisms, we progressively aggregate and fuse multimodal image features at various stages of image feature learning. Throughout the process of multimodal interaction within the network, we employ a dynamic component feature fusion module at the patch-level to dynamically assess the relevance of visible information within each region of the tracking scene.
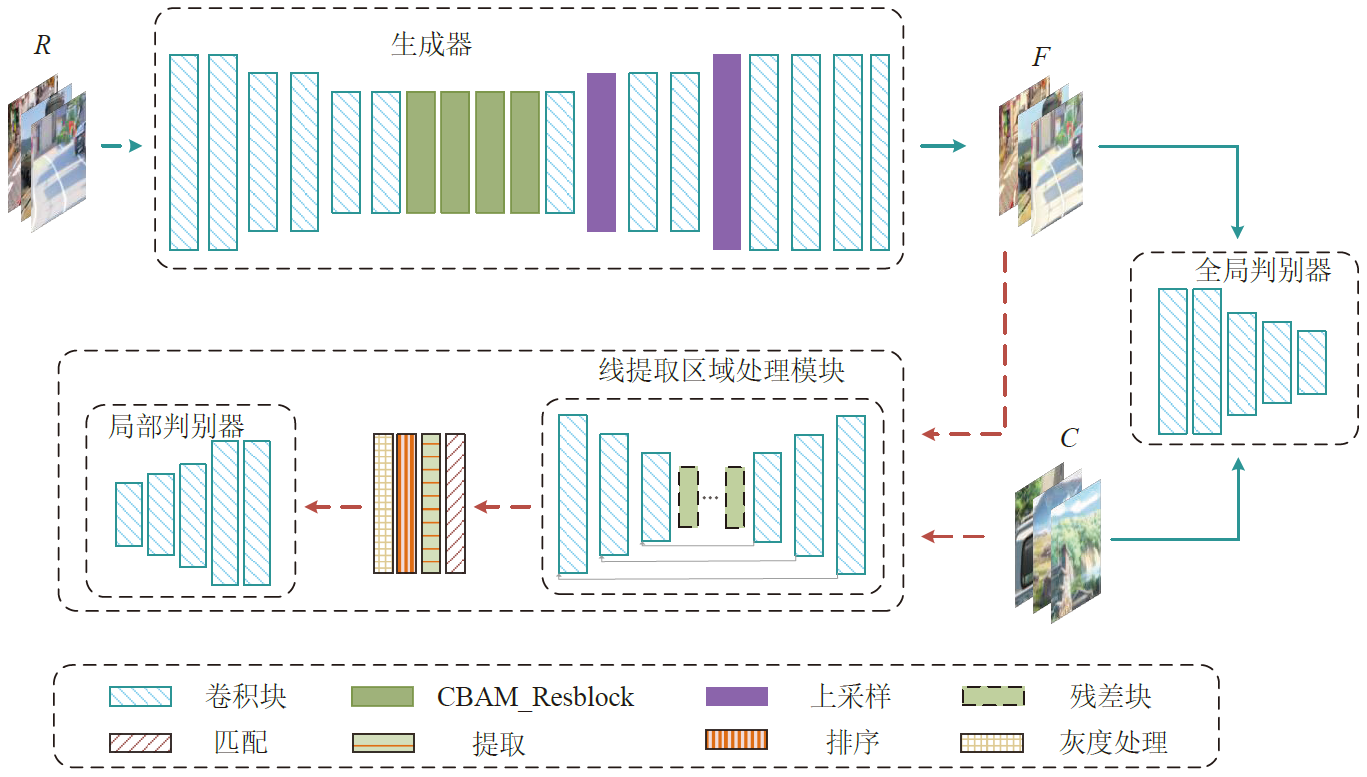
An image cartoonization method that incorporated attention mechanism and structural line extraction was proposed in order to address the problem that image cartoonization does not highlight important feature information in the image and insufficient edge processing. The generator network with fused attention mechanism was constructed, which extracted more important and richer image information from different features by fusing the connections between features in space and channels. A line extraction region processing module (LERM) in parallel with the global one was designed to perform adversarial training on the edge regions of cartoon textures in order to better learn cartoon textures.
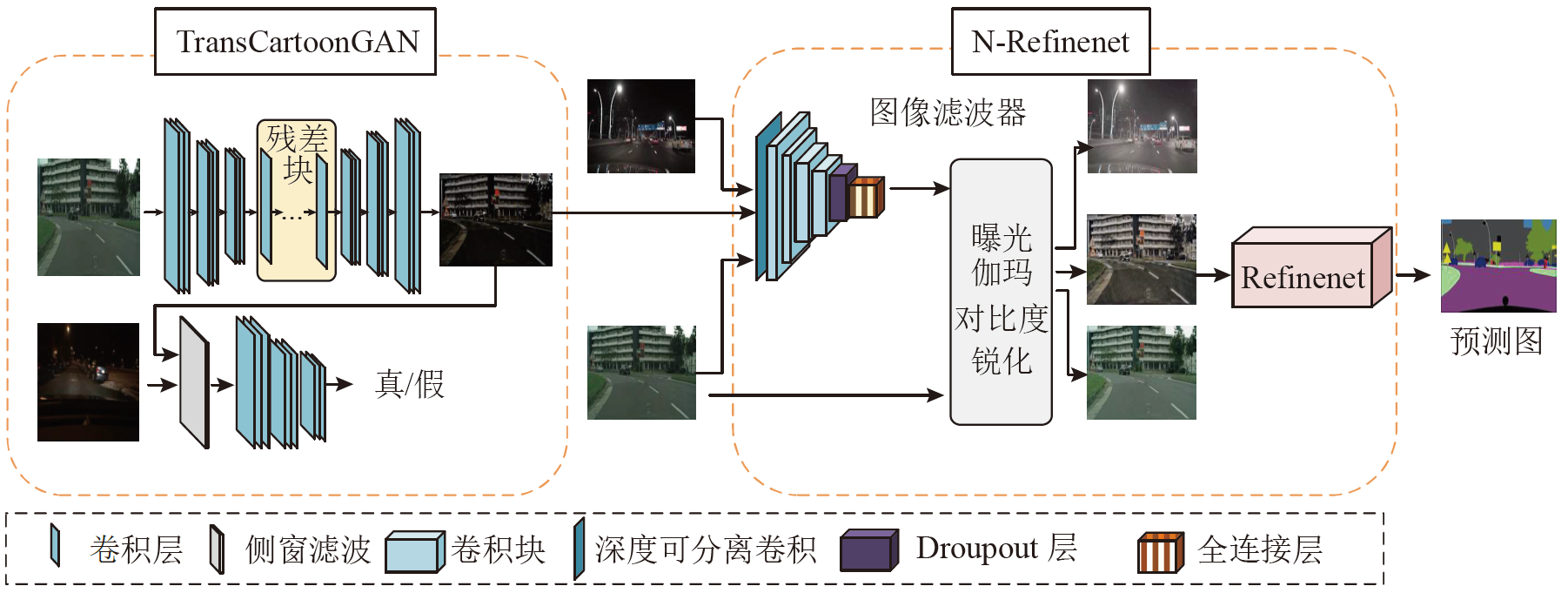
The semantic segmentation method Trans-nightSeg was proposed aiming at the issues of low brightness and lack of annotated semantic segmentation dataset in nighttime road scenes. The annotated daytime road scene semantic segmentation dataset Cityscapes was converted into low-light road scene images by TransCartoonGAN, which shared the same semantic segmentation annotation, thereby enriching the nighttime road scene dataset. The result together with the real road scene dataset was used as input of N-Refinenet. The N-Refinenet network introduced a low-light image adaptive enhancement network to improve the semantic segmentation performance of the nighttime road scene. Depth-separable convolution was used instead of normal convolution in order to reduce the computational complexity.
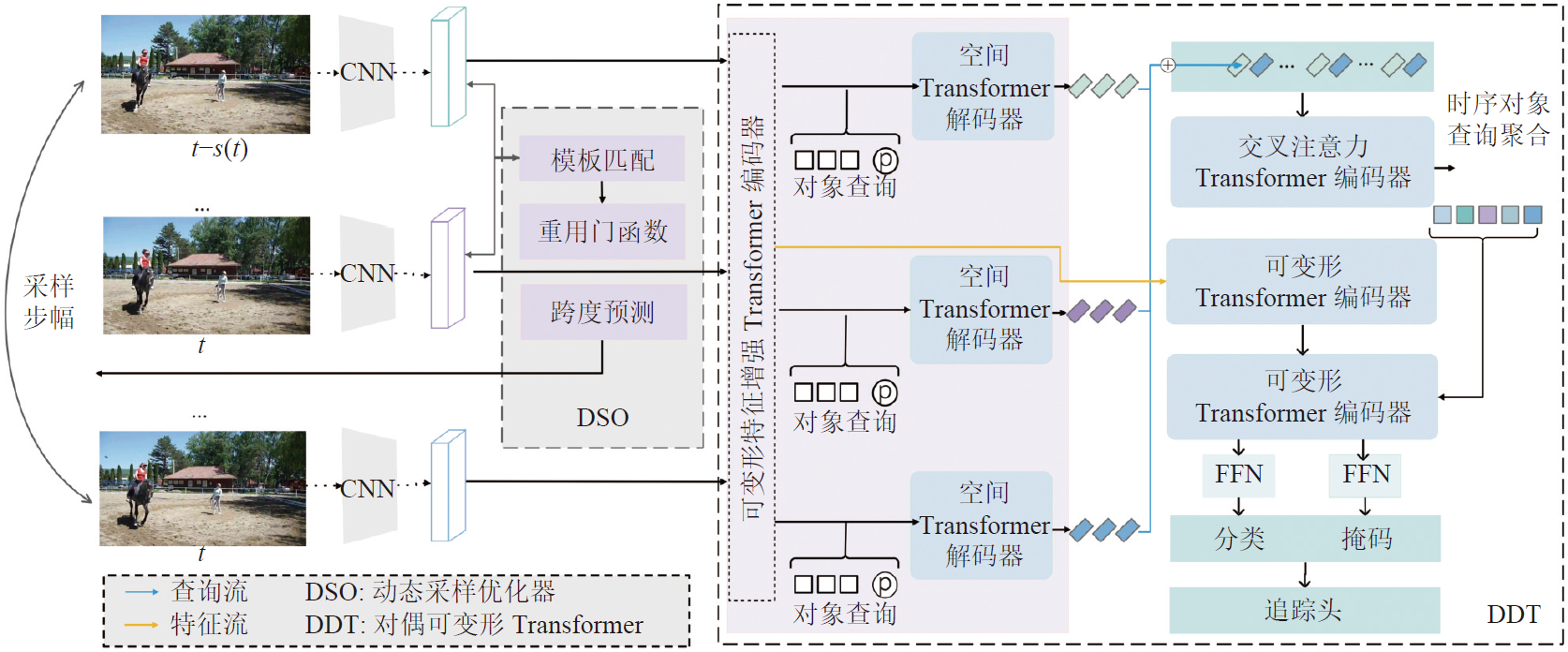
The dynamic sampling dual deformable network (DSDDN) was proposed in order to enhance the inference speed of video instance segmentation by better using temporal information within video frames. A dynamic sampling strategy was employed, which adjusted the sampling policy based on the similarity between consecutive frames. The inference process for the current frame was skipped for frames with high similarity by utilizing only segmentation results from the preceding frame for straightforward transfer computation. Frames with a larger temporal span were dynamically aggregated for frames with low similarity in order to enhance information for the current frame. Two deformable operations were additionally incorporated within the Transformer structure to circumvent the exponential computational cost associated with attention-based methods. The complex network was optimized through carefully designed tracking heads and loss functions.
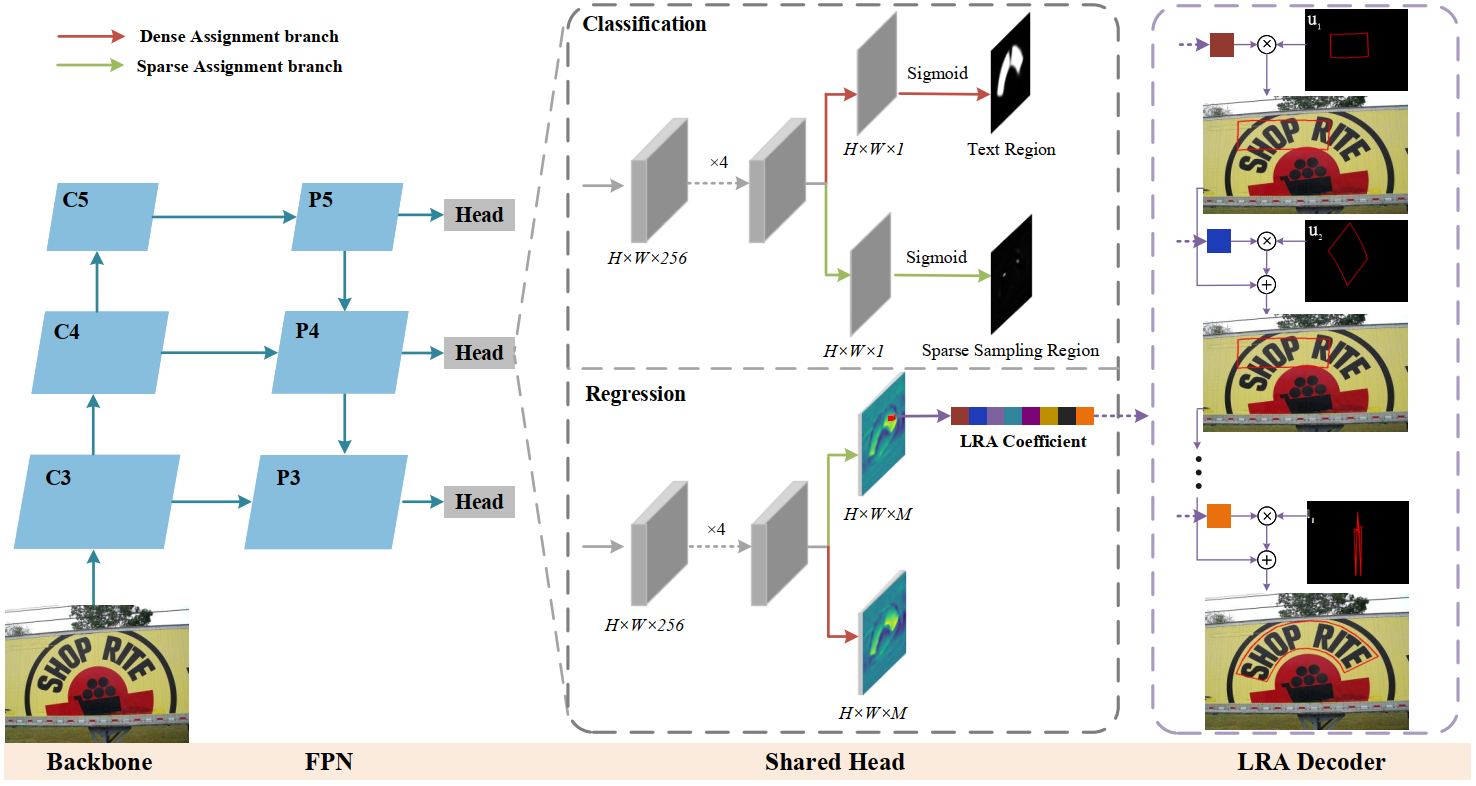
We first propose a novel parameterized text shape method based on low-rank approximation. Unlike other shape representation methods that employ data-irrelevant parameterization, our approach utilizes singular value decomposition and reconstructs the text shape using a few eigenvectors learned from labeled text contours. By exploring the shape correlation among different text contours, our method achieves consistency, compactness, simplicity, and robustness in shape representation. Next, we propose a dual assignment scheme for speed acceleration. It adopts a sparse assignment branch to accelerate the inference speed, and meanwhile, provides ample supervised signals for training through a dense assignment branch. Building upon these designs, we implement an accurate and efficient arbitrary-shaped text detector named LRANet.
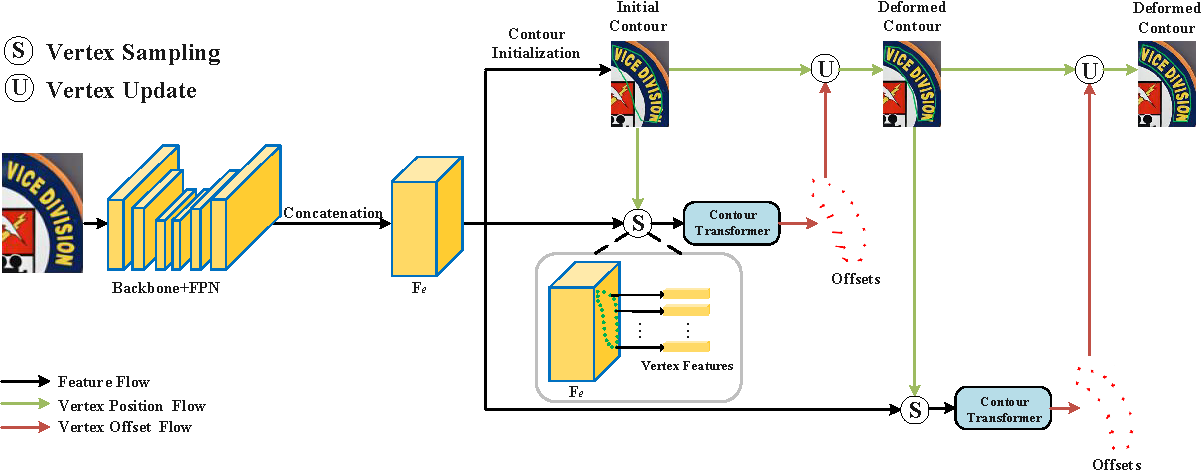
We propose a novel arbitrary-shaped scene text detection framework named CT-Net by progressive contour regression with contour transformers. Specifically, we first employ a contour initialization module that generates coarse text contours without any post-processing. Then, we adopt contour refinement modules to adaptively refine text contours in an iterative manner, which are beneficial for context information capturing and progressive global contour deformation. Besides, we propose an adaptive training strategy to enable the contour transformers to learn more potential deformation paths, and introduce a re-score mechanism that can effectively suppress false positives.
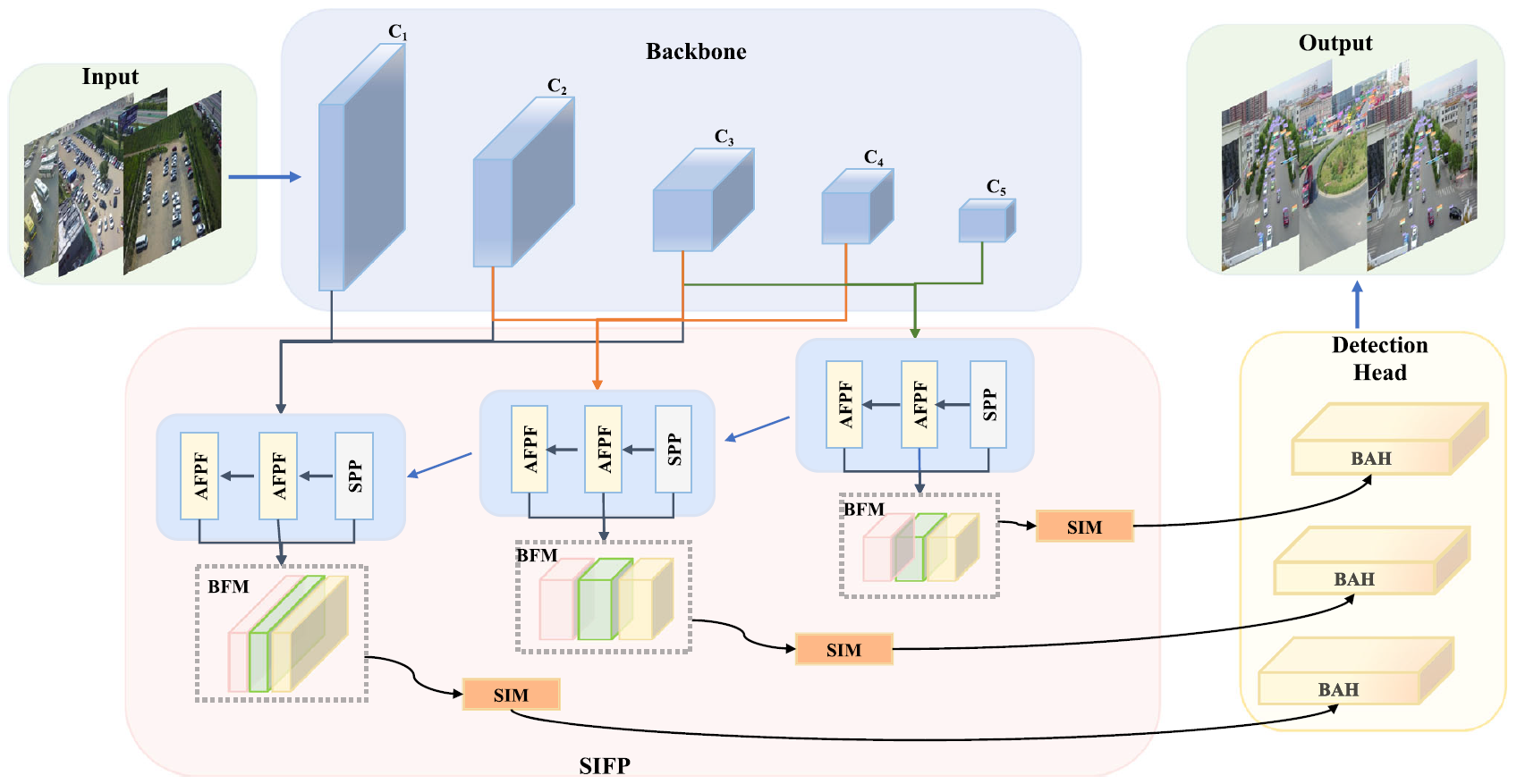
We propose an effective boundary-aware network with attention refinement and spatial interaction to tackle the above challenges. Specifically, we first present a highly effective yet simple boundary-aware detection head (BAH), which directly guides representation learning of object structure semantics in the prediction layer to preserve object-related boundary semantics. Additionally, the attentional feature parallel fusion (AFPF) module offers multi-scale feature encoding capability in a parallel triple fusion fashion and adaptively selects features appropriate for objects of certain scales. Furthermore, we design a spatial interactive module (SIM) to preserve fine spatial detail through cross-spatial feature association.
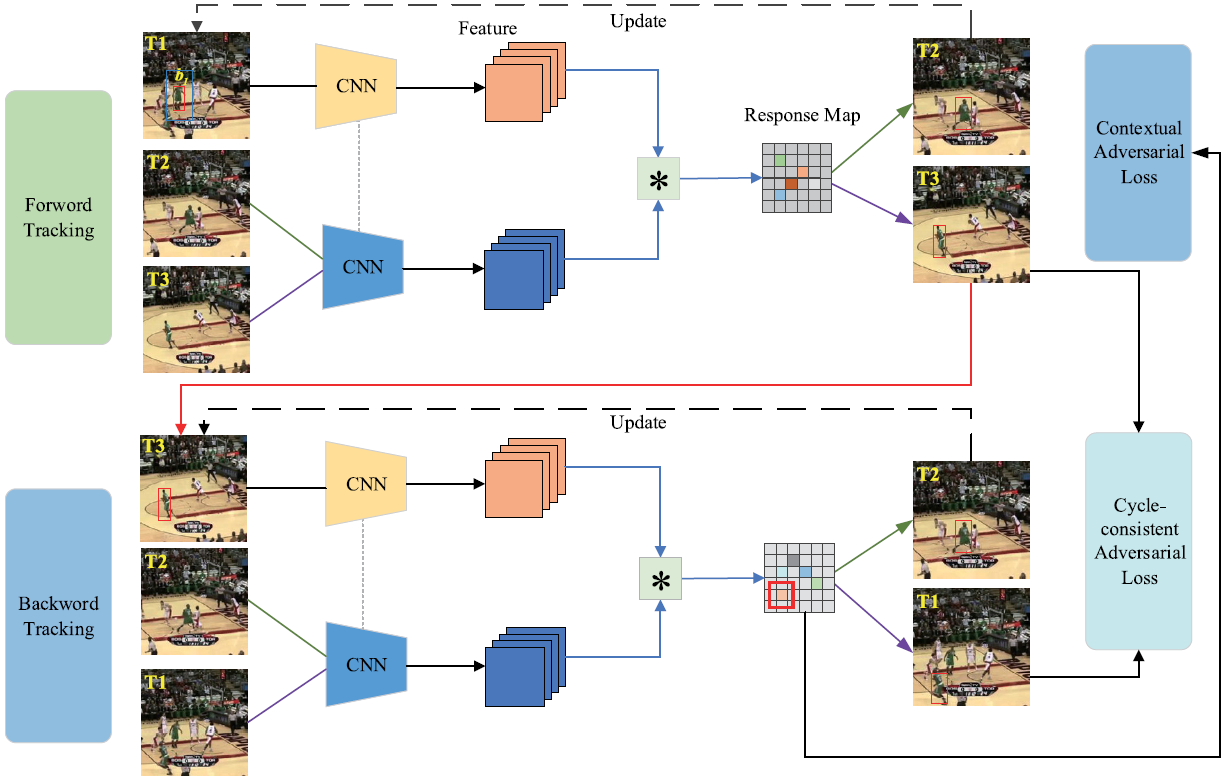
This paper presents an unsupervised attack methodology against visual object tracking models. The approach employs the cycle consistency principle of object tracking models to maximize the inconsistency between forward and backward tracking, thereby providing effective countermeasures. Additionally, this paper introduces a contextual attack method, leveraging the information from the attack object’s region and its surrounding contextual regions. This strategy attacks the object region and its surrounding context regions simultaneously, aiming to decrease its response score to the attack. The proposed attack method is assessed across various types of deep learning-based object trackers.
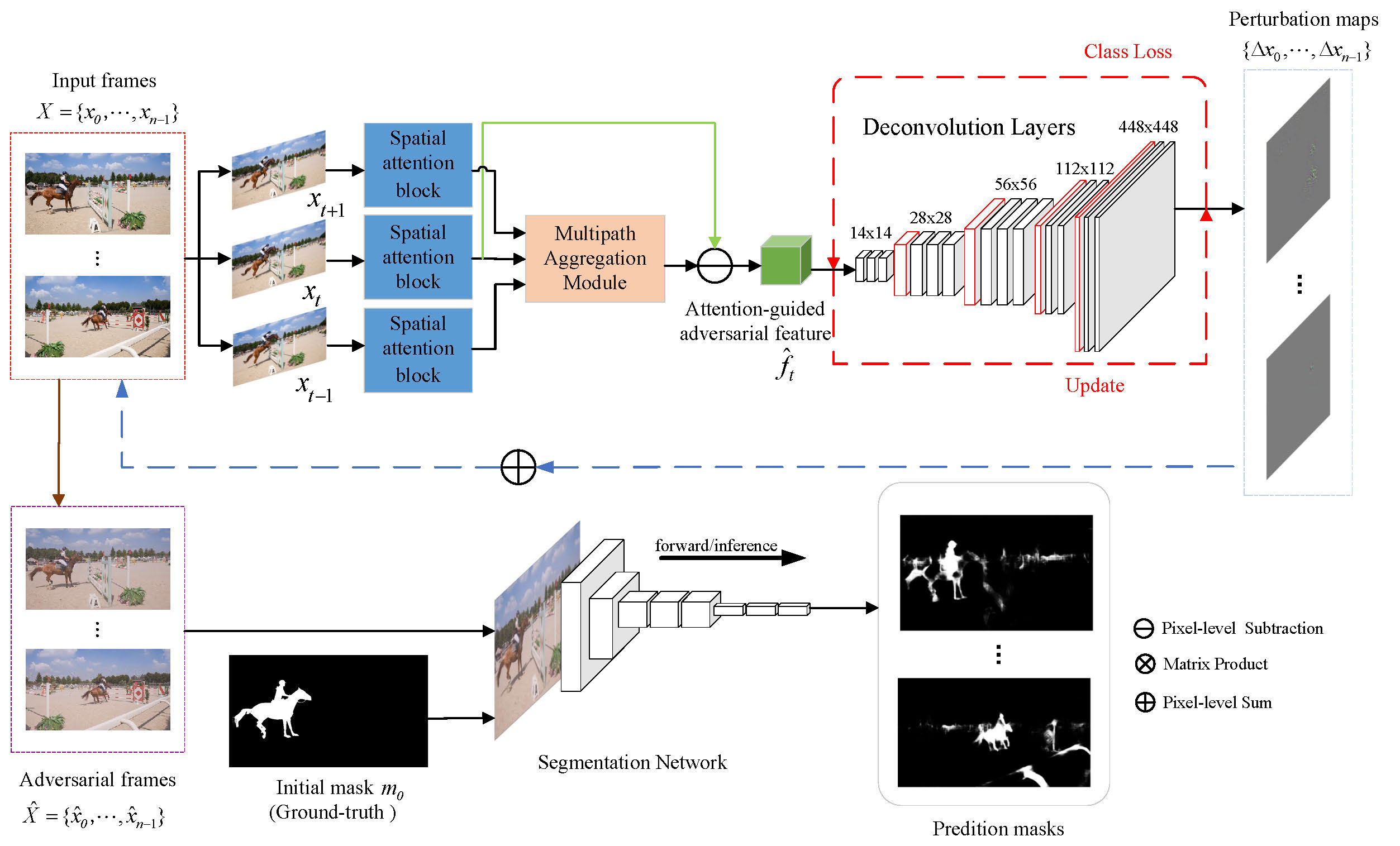
We propose an attention-guided adversarial attack method, which uses spatial attention blocks to capture features with global dependencies to construct correlations between consecutive video frames, and performs multipath aggregation to effectively integrate spatial-temporal perturbation, thereby guiding the deconvolution network to generate adversarial example with strong attack capability. Specifically, the class loss function is designed to enable the deconvolution network to better activate noise in other regions and suppress the activation related to the object class based on the enhanced feature map of the object class. At the same time, attentional feature loss is designed to enhance the transferability against attack.
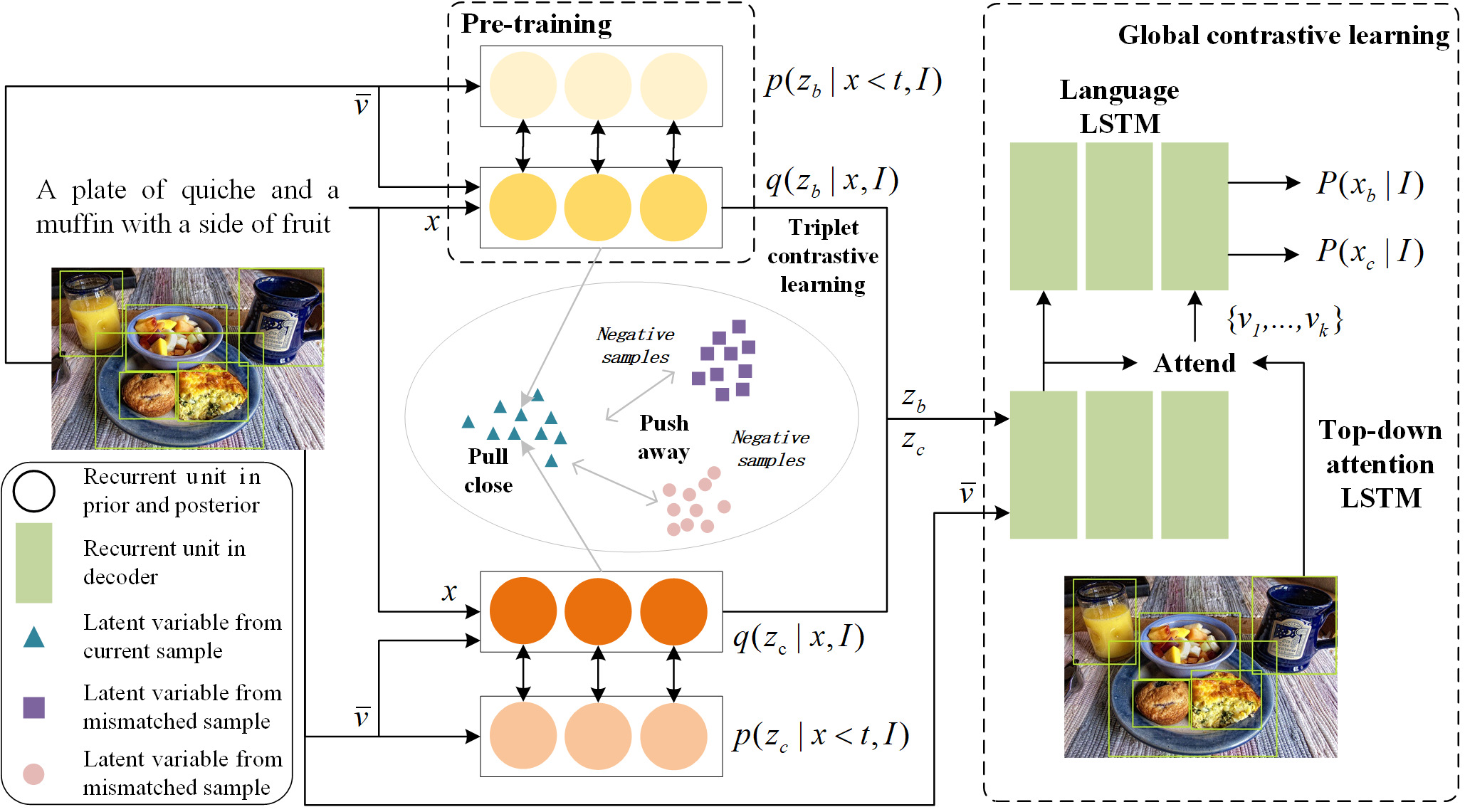
We propose a novel Conditional Variational Autoencoder (DCL-CVAE) framework for diverse image captioning by seamlessly integrating sequential variational autoencoder with contrastive learning. In the encoding stage, we first build conditional variational autoencoders to separately learn the sequential latent spaces for a pair of captions. Then, we introduce contrastive learning in the sequential latent spaces to enhance the discriminability of latent representations for both image-caption pairs and mismatched pairs. In the decoding stage, we leverage the captions sampled from the pre-trained Long Short-Term Memory (LSTM), LSTM decoder as the negative examples and perform contrastive learning with the greedily sampled positive examples, which can restrain the generation of common words and phrases induced by the cross entropy loss. By virtue of dual constrastive learning, DCL-CVAE is capable of encouraging the discriminability and facilitating the diversity, while promoting the accuracy of the generated captions.
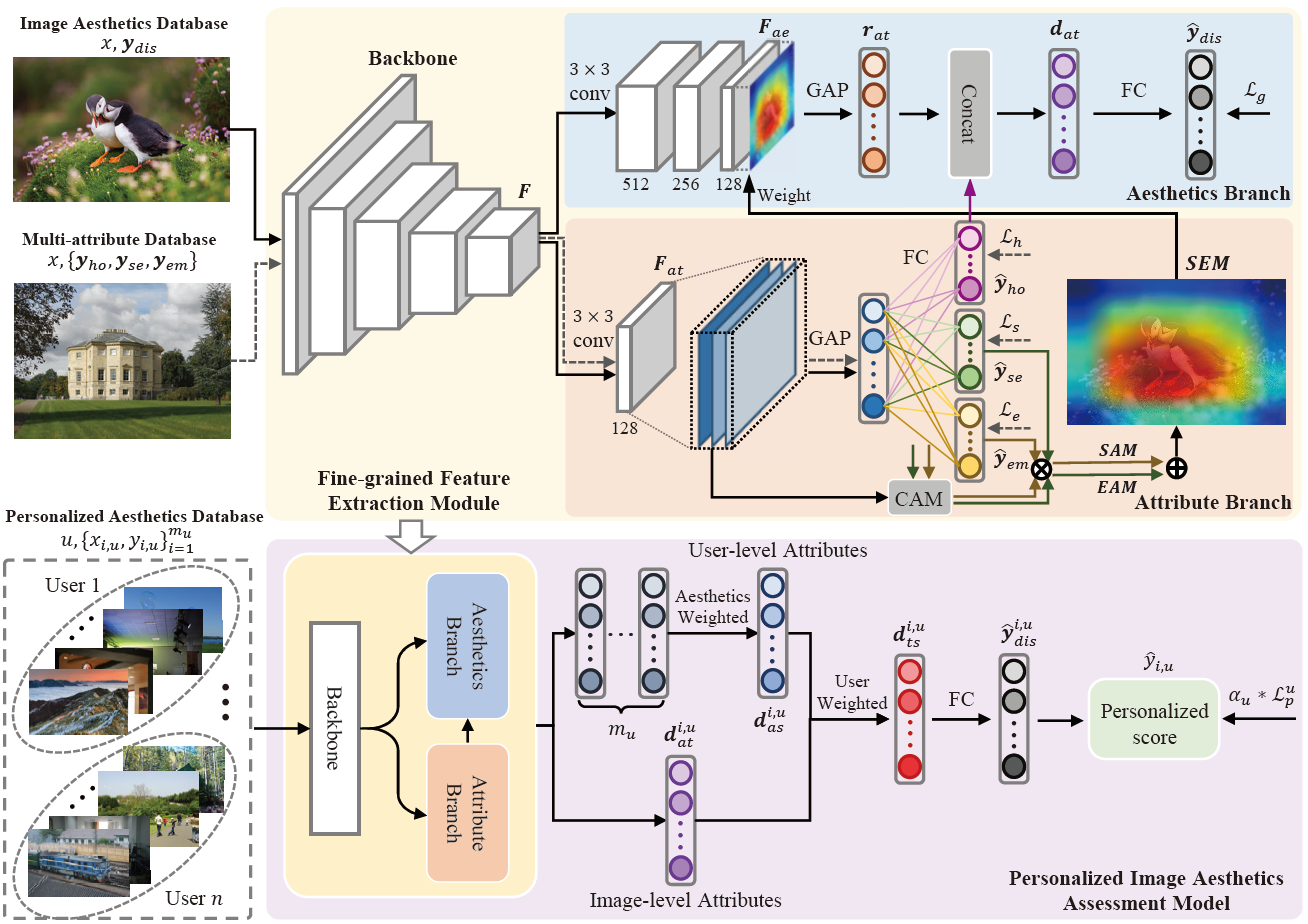
We first build a fine-grained feature extraction (FFE) module to obtain the refined local features of image attributes to compensate for holistic features. The FFE module is then used to generate user-level features, which are combined with the image-level features to obtain user-preferred fine-grained feature representations. By training extensive PIAA tasks, the aesthetic distribution of most users can be transferred to the personalized scores of individual users. To enable our proposed model to learn more generalizable aesthetics among individual users, we incorporate the degree of dispersion between personalized scores and image aesthetic distribution as a coefficient in the loss function during model training.
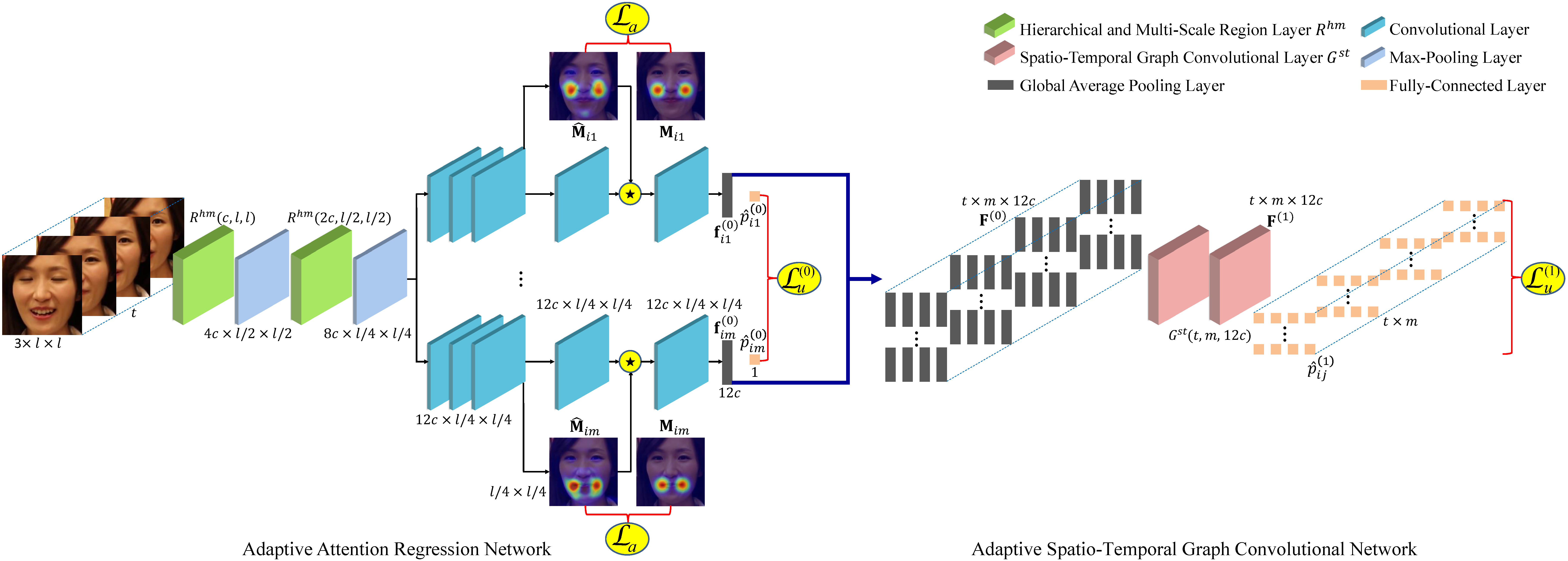
We propose a novel adaptive attention and relation (AAR) framework for facial AU detection. Specifically, we propose an adaptive attention regression network to regress the global attention map of each AU under the constraint of attention predefinition and the guidance of AU detection, which is beneficial for capturing both specified dependencies by landmarks in strongly correlated regions and facial globally distributed dependencies in weakly correlated regions. Moreover, considering the diversity and dynamics of AUs, we propose an adaptive spatio-temporal graph convolutional network to simultaneously reason the independent pattern of each AU, the inter-dependencies among AUs, as well as the temporal dependencies.
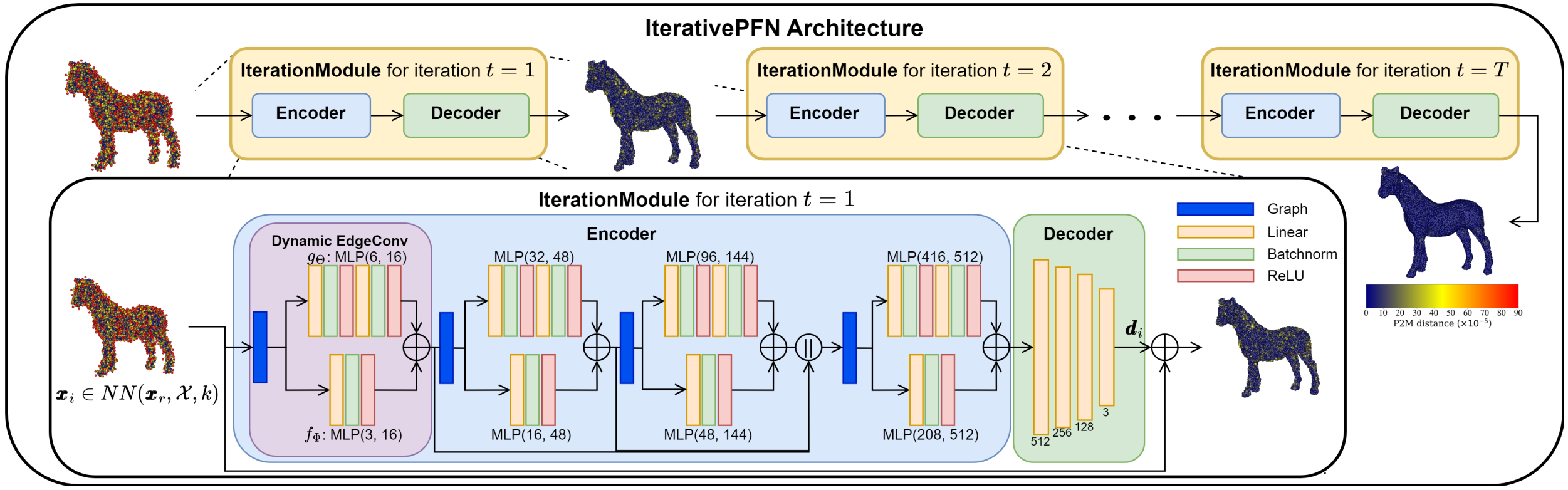
We propose IterativePFN (iterative point cloud filtering network), which consists of multiple IterationModules that model the true iterative filtering process internally, within a single network. We train our IterativePFN network using a novel loss function that utilizes an adaptive ground truth target at each iteration to capture the relationship between intermediate filtering results during training. This ensures filtered results converge faster to the clean surfaces.
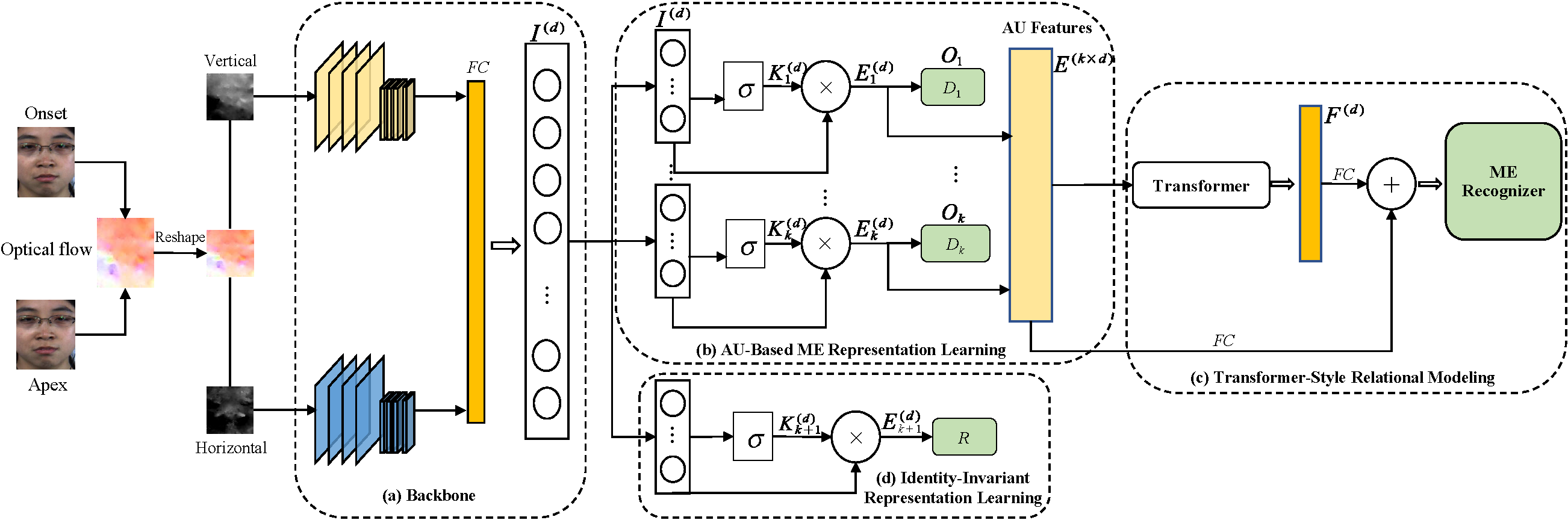
We propose a novel MER method by identity-invariant representation learning and transformer-style relational modeling. Specifically, we propose to disentangle the identity information from the input via an adversarial training strategy. Considering the coherent relationships between AUs and MEs, we further employ AU recognition as an auxiliary task to learn AU representations with ME information captured. Moreover, we introduce a transformer to achieve MER by modeling the correlations among AUs. MER and AU recognition are jointly trained, in which the two correlated tasks can contribute to each other.
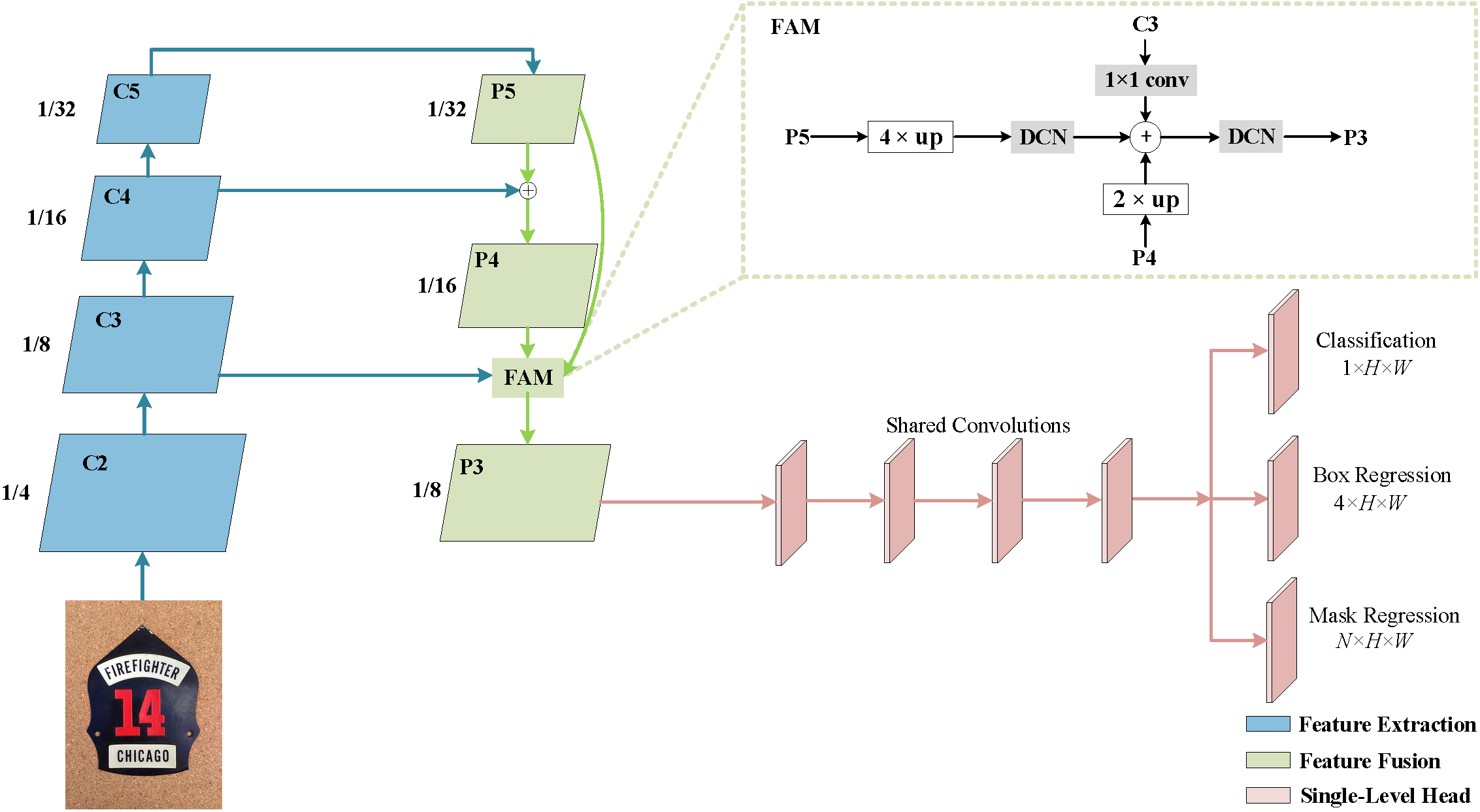
We propose a novel light-weight anchor-free text detection framework called TextDCT, which adopts the discrete cosine transform (DCT) to encode the text masks as compact vectors. Further, considering the imbalanced number of training samples among pyramid layers, we only employ a single-level head for top-down prediction. To model the multi-scale texts in a single-level head, we introduce a novel positive sampling strategy by treating the shrunk text region as positive samples, and design a feature awareness module (FAM) for spatial-awareness and scale-awareness by fusing rich contextual information and focusing on more significant features. Moreover, we propose a segmented non-maximum suppression (S-NMS) method that can filter low-quality mask regressions.
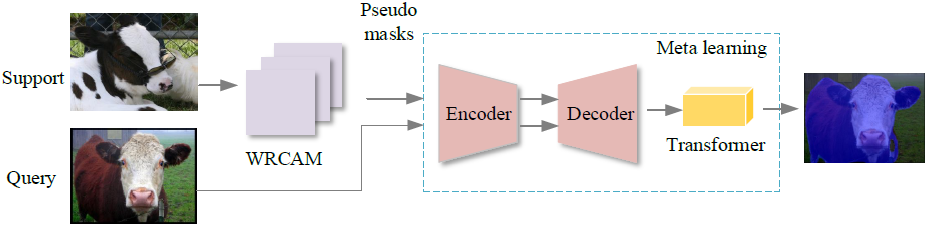
We propose a weakly supervised few-shot semantic segmentation model based on the meta learning framework, which utilizes prior knowledge and adjusts itself according to new tasks. Thereupon then, the proposed network is capable of both high efficiency and generalization ability to new tasks. In the pseudo mask generation stage, we develop a WRCAM method with the channel-spatial attention mechanism to refine the coverage size of targets in pseudo masks. In the few-shot semantic segmentation stage, the optimization based meta learning method is used to realize few-shot semantic segmentation by virtue of the refined pseudo masks.
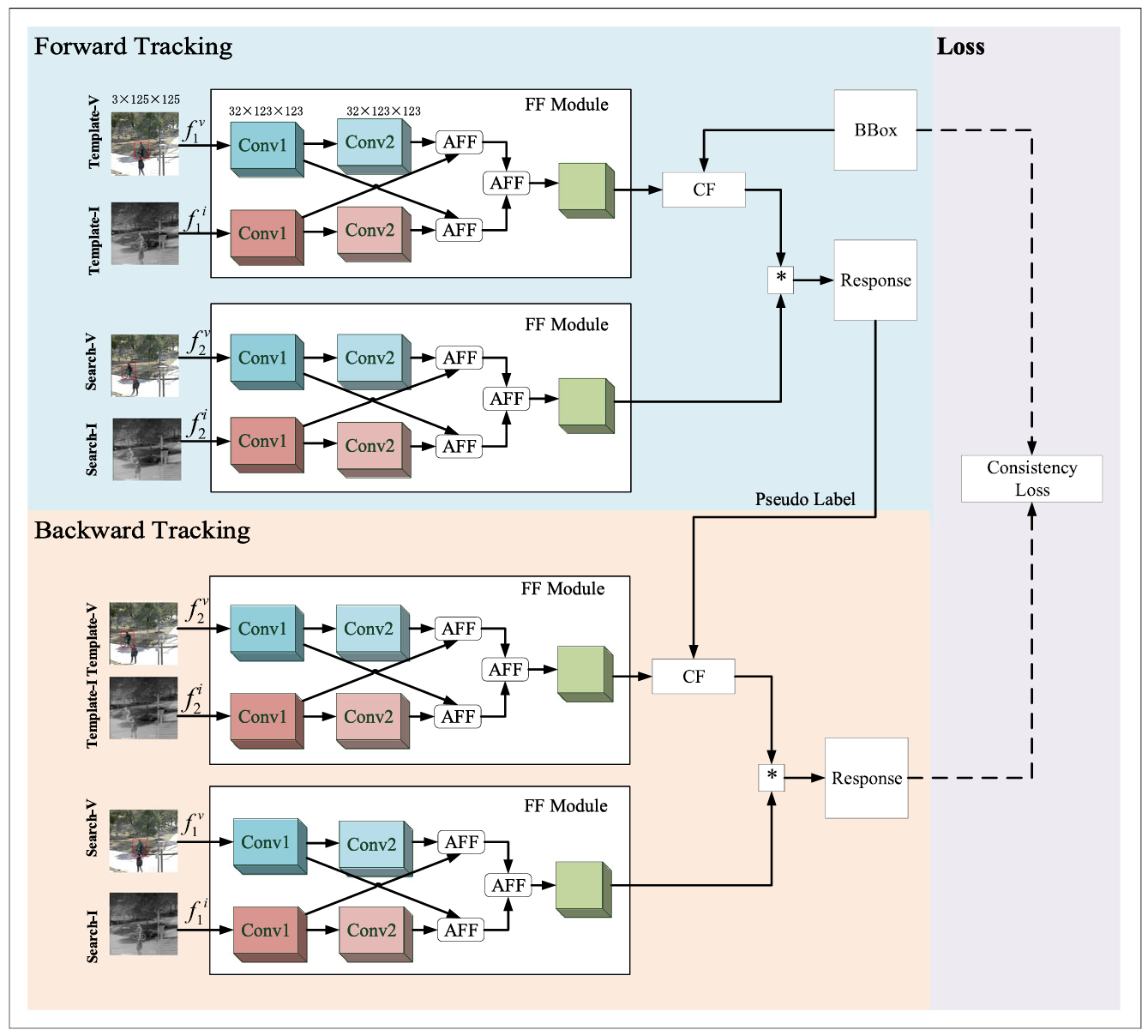
We propose a framework for visual tracking based on the attention mechanism fusion of multi-modal and multi-level features. This fusion method can give full play to the advantages of multi-level and multi-modal information. Specificly, we use a feature fusion module to fuse these features from different levels and different modalities at the same time. We use cycle consistency based on a correlation filter to implement unsupervised training of the model to reduce the cost of annotated data.
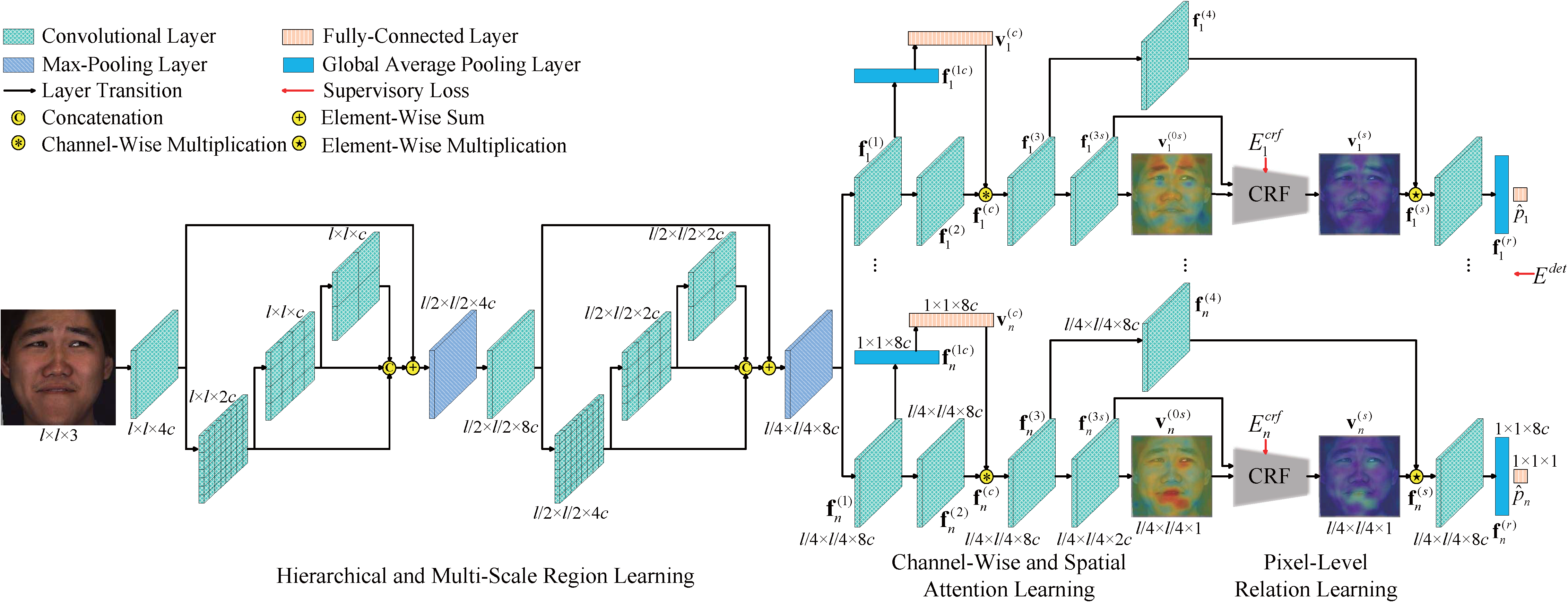
We propose an end-to-end deep learning based attention and relation learning framework for AU detection with only AU labels, which has not been explored before. In particular, multi-scale features shared by each AU are learned firstly, and then both channel-wise and spatial attentions are adaptively learned to select and extract AU-related local features. Moreover, pixel-level relations for AUs are further captured to refine spatial attentions so as to extract more relevant local features. Without changing the network architecture, our framework can be easily extended for AU intensity estimation.
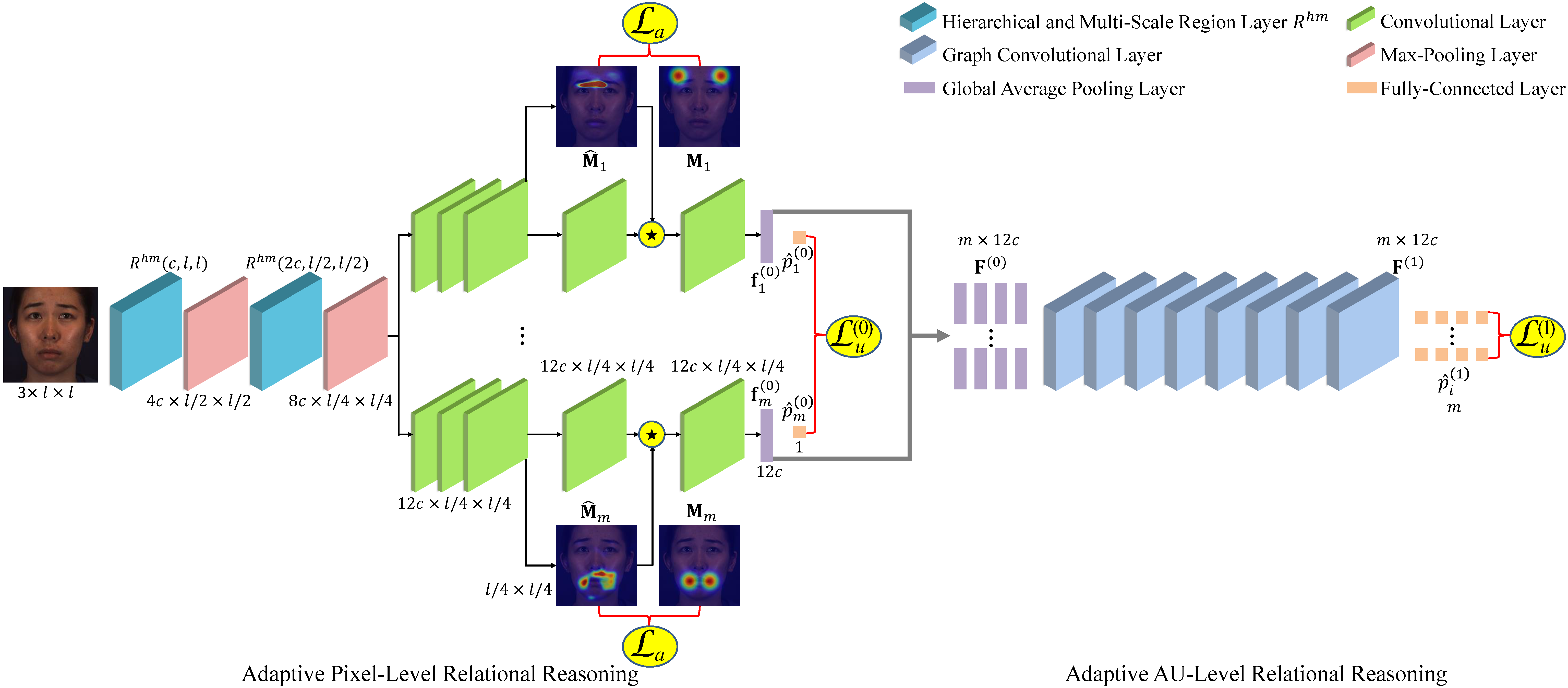
We propose a novel hybrid relational reasoning (HRR) framework for AU detection. In particular, we propose to adaptively reason pixel-level correlations of each AU, under the constraint of predefined regional correlations by facial landmarks, as well as the supervision of AU detection. Moreover, we propose to adaptively reason AU-level correlations using a graph convolutional network, by considering both predefined AU relationships and learnable relationship weights. Our framework is beneficial for integrating the advantages of correlation predefinition and correlation learning.
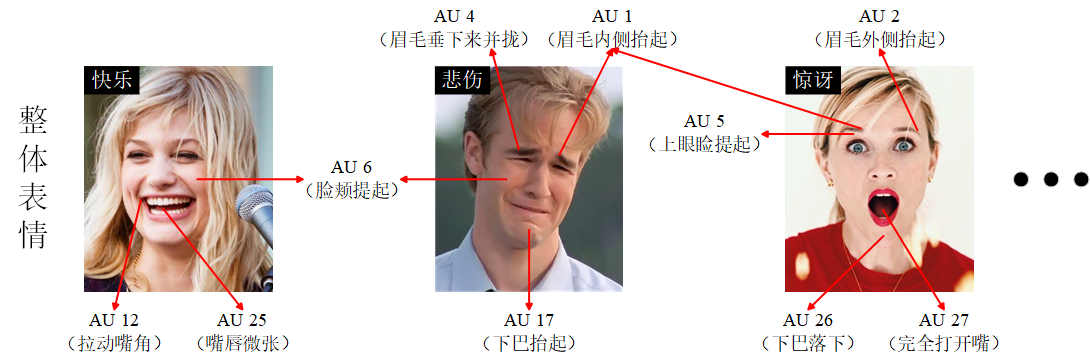
Expression action unit (AU) recognition based on deep learning is a hot topic in the fields of computer vision and affective computing. Each AU describes a facial local expression action, and the combinations of AUs can quantitatively represent any expression. Current AU recognition mainly faces three challenging factors: scarcity of labels, difficulty of feature capture, and imbalance of labels. On the basis of this, this paper categorizes the existing researches into transfer learning based, region learning based, and relation learning based methods, and comments and summarizes each category of representative methods. Finally, this paper compares and analyzes different methods, and further discusses the future research directions of AU recognition.
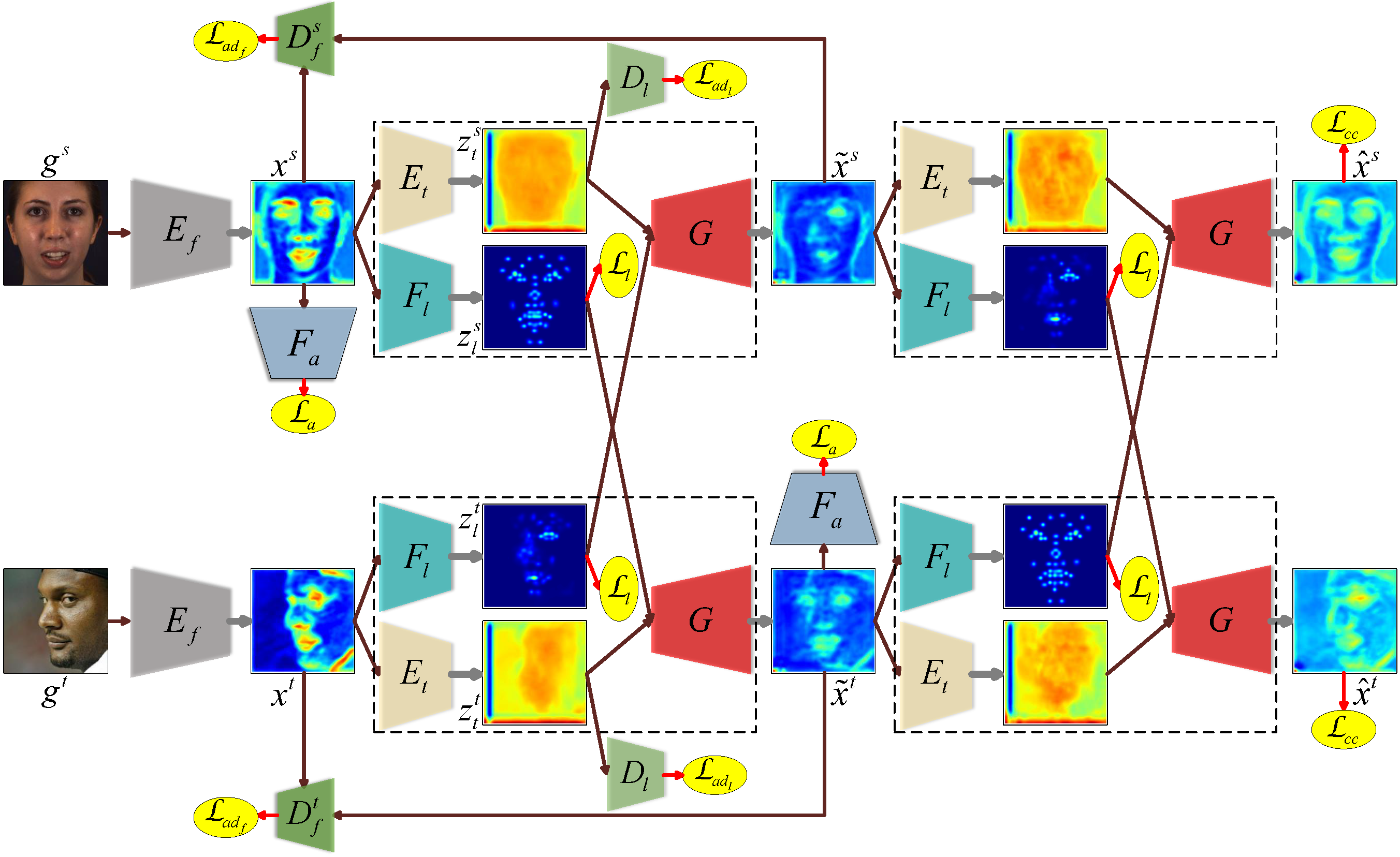
We propose an end-to-end unconstrained facial AU detection framework based on domain adaptation, which transfers accurate AU labels from a constrained source domain to an unconstrained target domain by exploiting labels of AU-related facial landmarks. Specifically, we map a source image with label and a target image without label into a latent feature domain by combining source landmark-related feature with target landmark-free feature. Due to the combination of source AU-related information and target AU-free information, the latent feature domain with transferred source label can be learned by maximizing the target-domain AU detection performance. Moreover, we introduce a novel landmark adversarial loss to disentangle the landmark-free feature from the landmark-related feature by treating the adversarial learning as a multi-player minimax game.
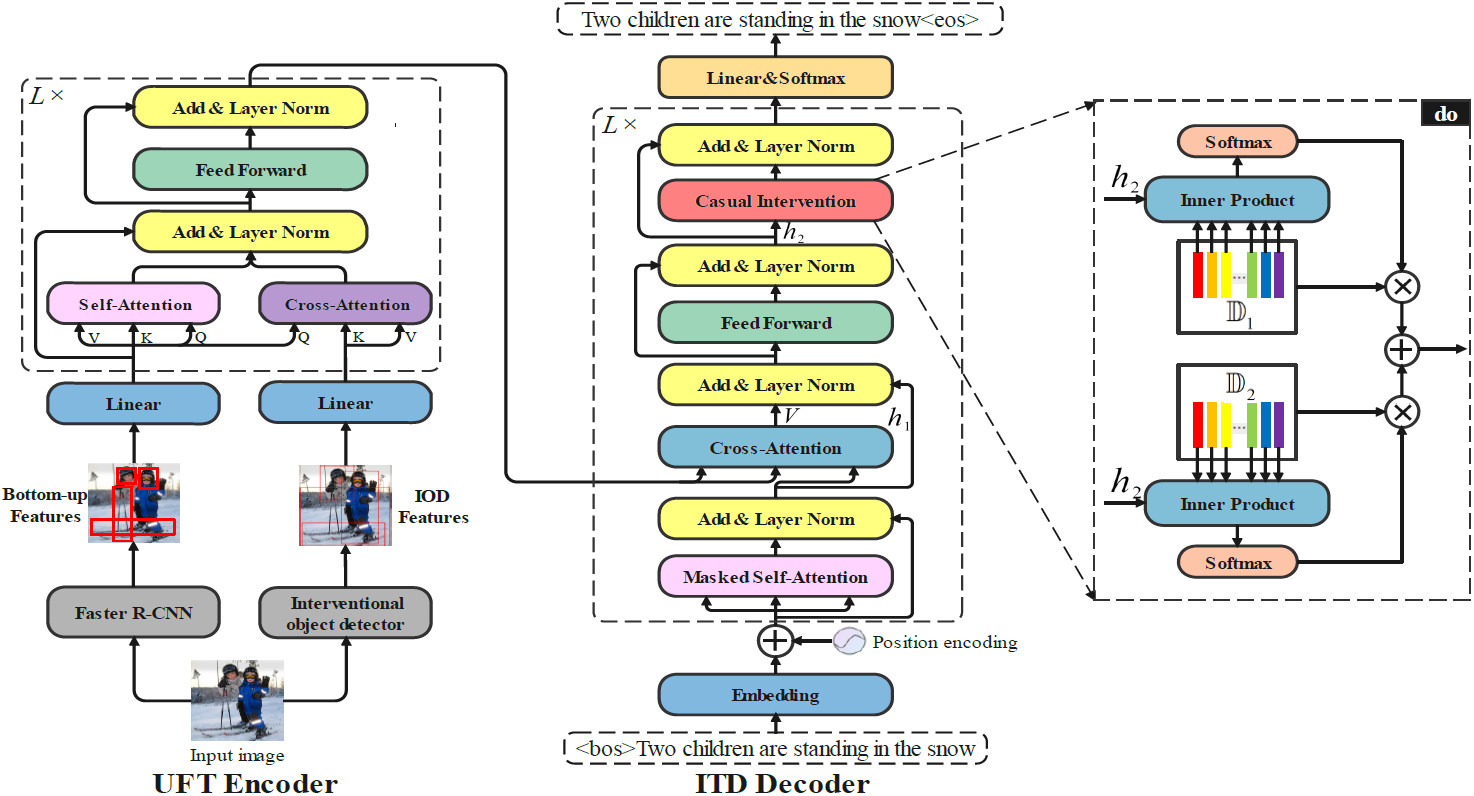
We first use Structural Causal Models (SCMs) to show how two confounders damage the image captioning. Then we apply the backdoor adjustment to propose a novel causal inference based image captioning (CIIC) framework, which consists of an interventional object detector (IOD) and an interventional transformer decoder (ITD) to jointly confront both confounders. In the encoding stage, the IOD is able to disentangle the region-based visual features by deconfounding the visual confounder. In the decoding stage, the ITD introduces causal intervention into the transform decoder and deconfounds the visual and linguistic confounders simultaneously. Two modules collaborate with each other to eliminate the spurious correlations caused by the unobserved confounders.
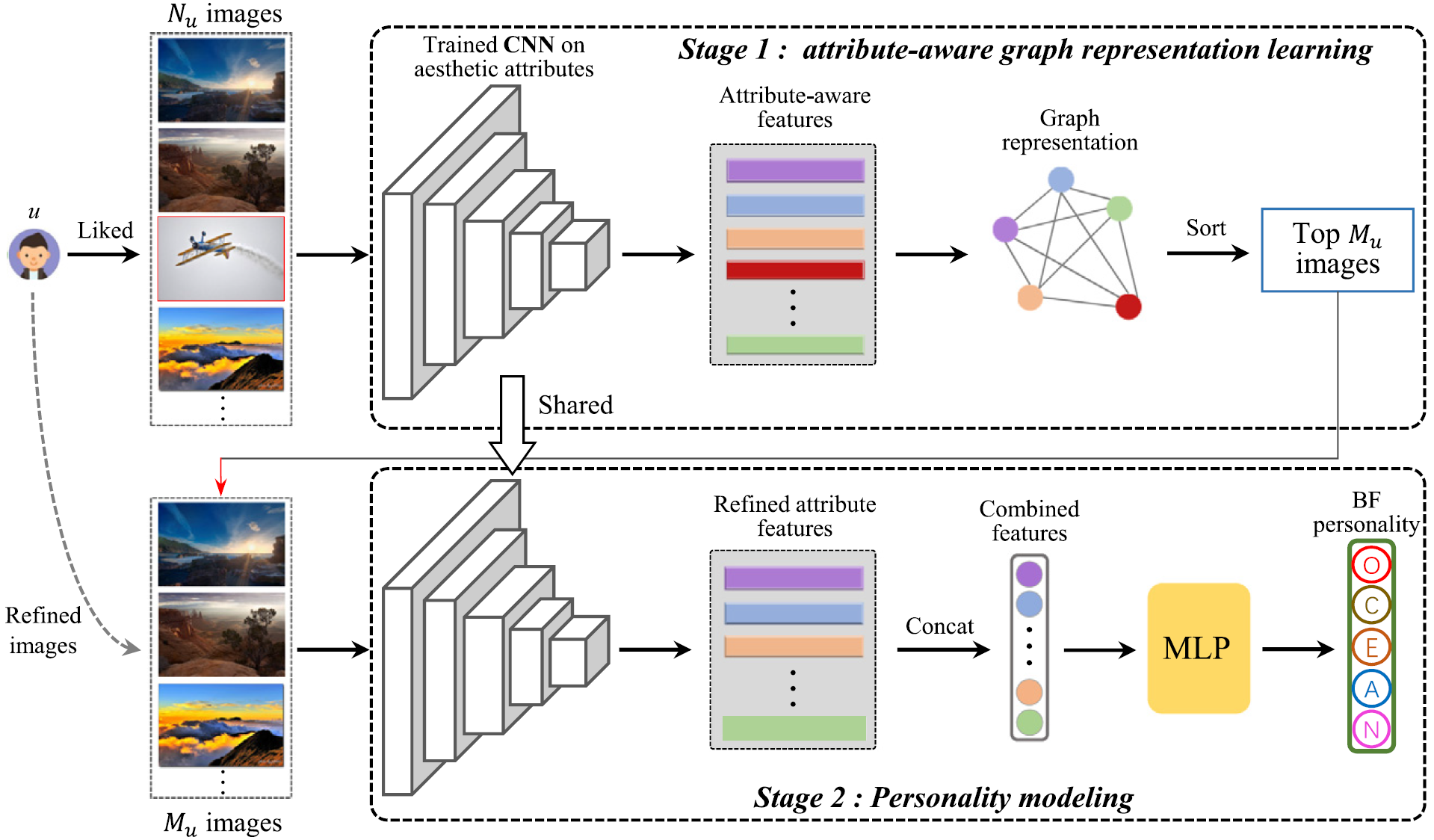
This paper proposes a personality modeling approach based on image aesthetic attribute-aware graph representation learning, which can leverage aesthetic attributes to refine the liked images that are consistent with users’ personality traits. Specifically, we first utilize a Convolutional Neural Network (CNN) to train an aesthetic attribute prediction module. Then, attribute-aware graph representation learning is introduced to refine the images with similar aesthetic attributes from users’ liked images. Finally, the aesthetic attributes of all refined images are combined to predict personality traits through a Multi-Layer Perceptron (MLP).
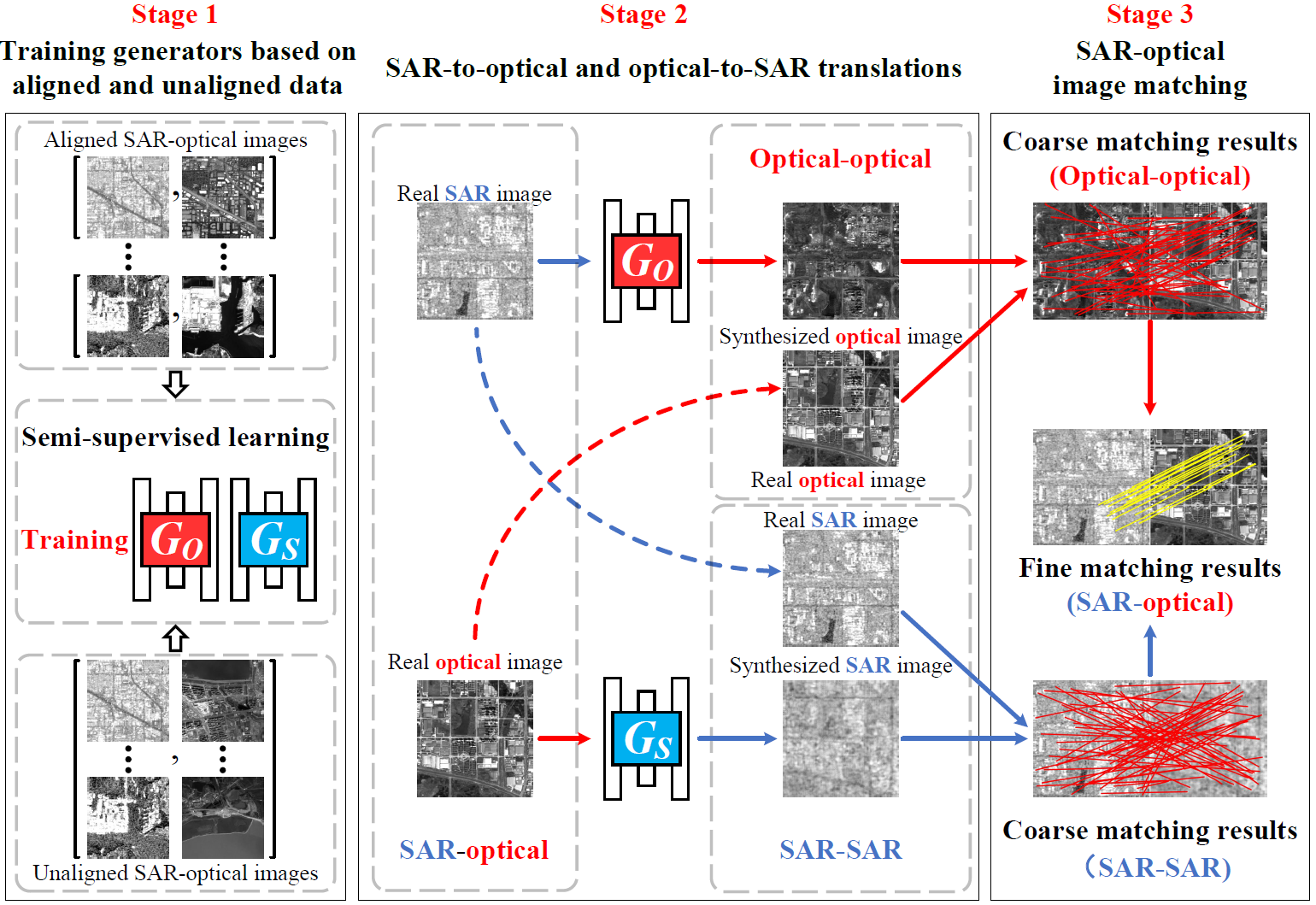
We investigate the applicability of semi-supervised image-to-image translation for SAR-optical image matching such that both aligned and unaligned SAR-optical images could be used. To this end, we combine the benefits of both supervised and unsupervised well-known image-to-image translation methods, i.e., Pix2pix and CycleGAN, and propose a simple yet effective semi-supervised image-to-image translation framework.
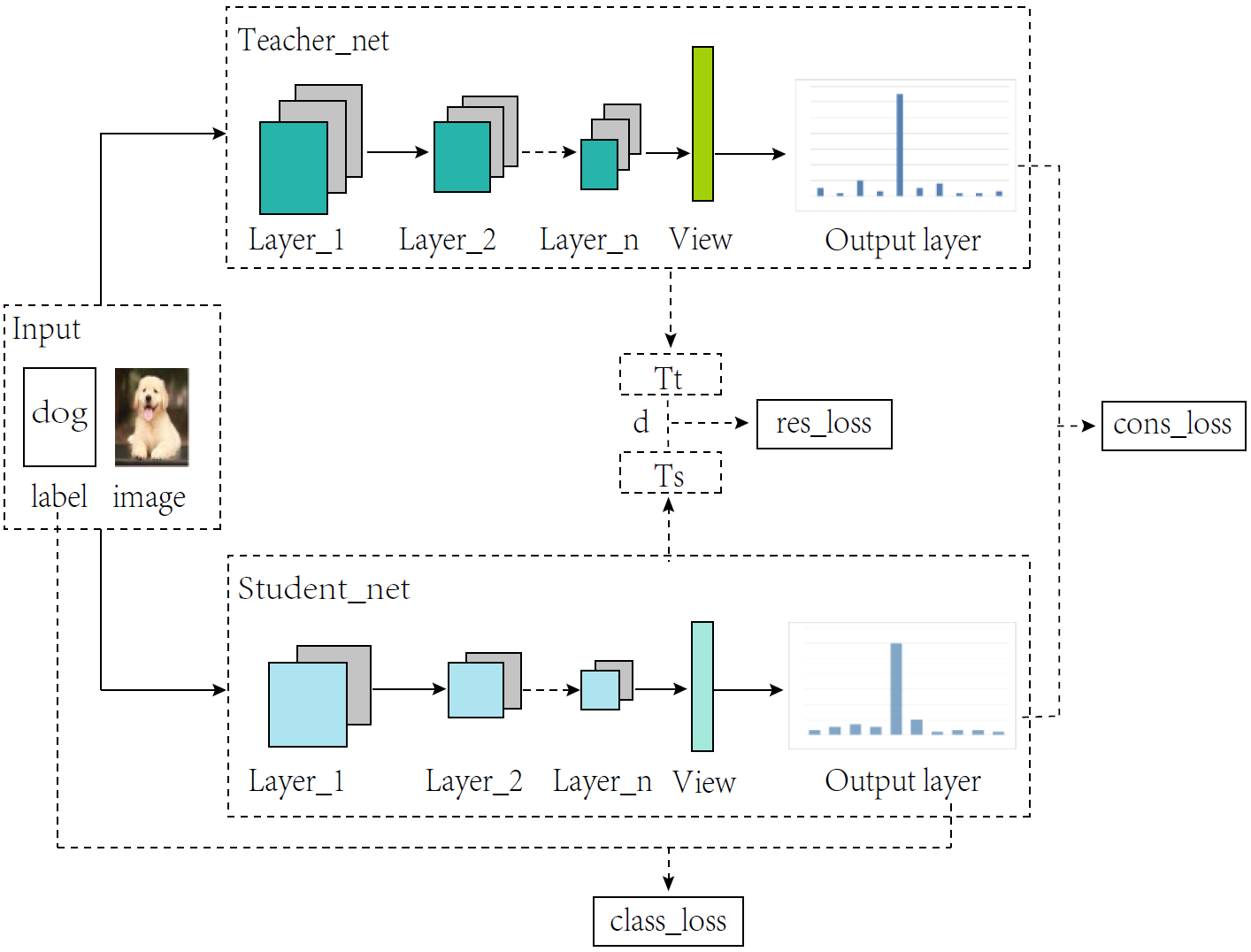
We explained how to use only a few of labels, design a more flexible network architecture and combine feature distillation method to improve model efficiency while ensuring high accuracy. Specifically, we integrate different network structures into independent individuals to make the use of network structures more flexible. Based on knowledge distillation, we extract the channel features and establish a feature distillation connection from the teacher network to the student network.
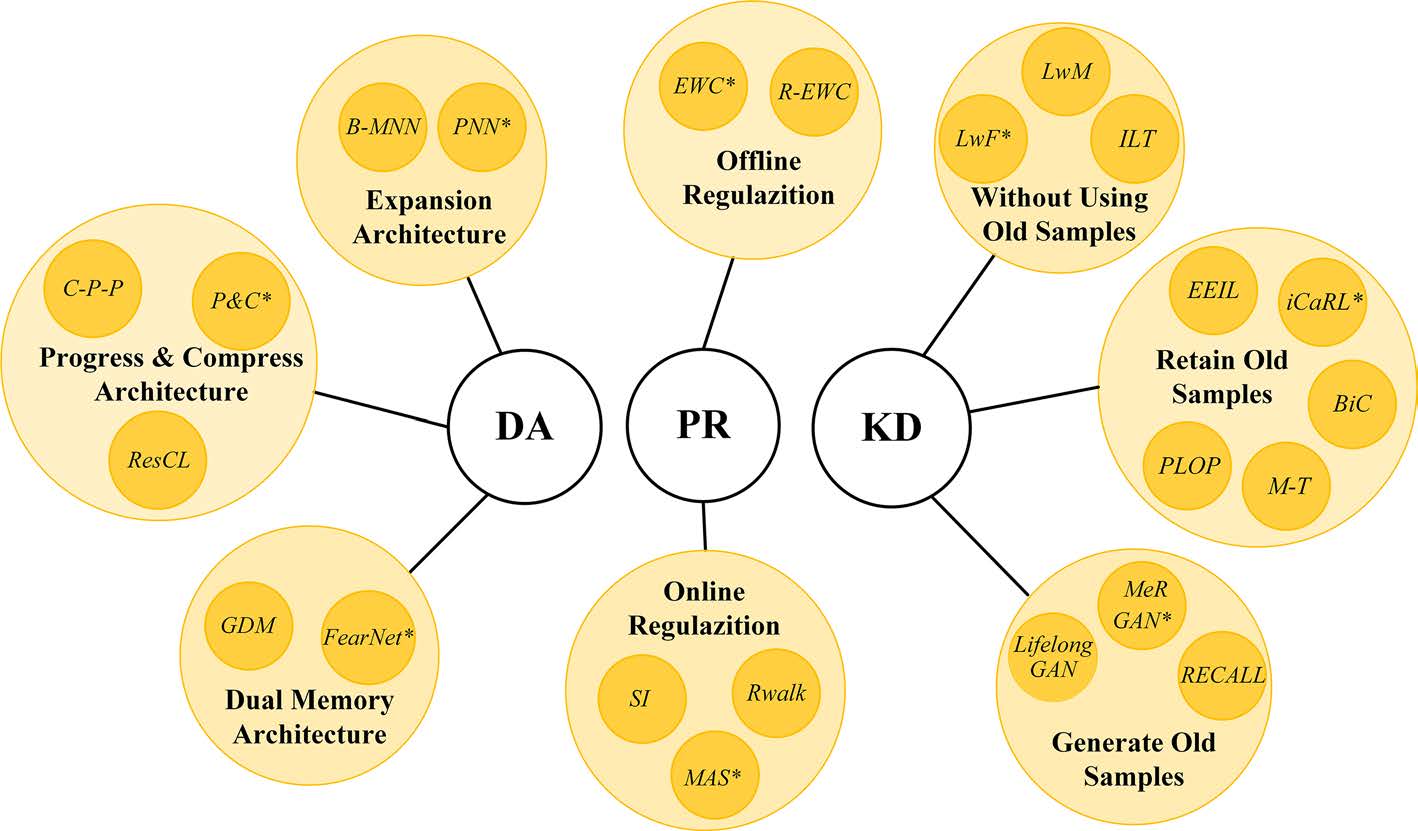
We systematically review the current development of incremental learning and give the overall taxonomy of the incremental learning methods. Specifically, three kinds of mainstream methods, i.e., parameter regularization-based approaches, knowledge distillation-based approaches, and dynamic architecture-based approaches, are surveyed, summarized, and discussed in detail. Furthermore, we comprehensively analyze the performance of data-permuted incremental learning, class-incremental learning, and multi-modal incremental learning on widely used datasets, covering a broad of incremental learning scenarios for image classification and semantic segmentation. Lastly, we point out some possible research directions and inspiring suggestions for incremental learning in the field of computer vision.
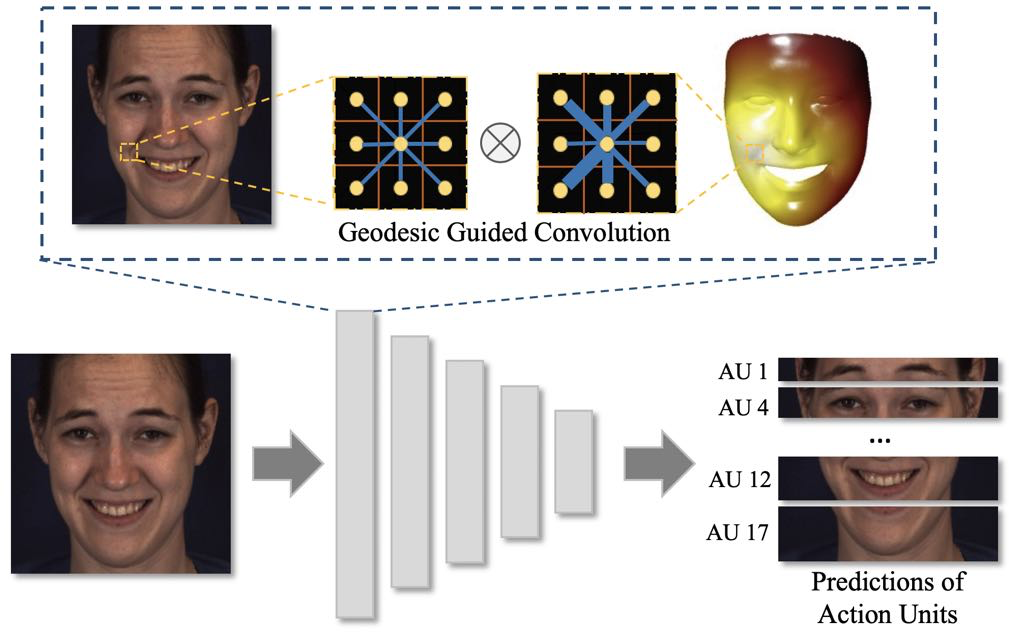
We propose a novel geodesic guided convolution (GeoConv) for AU recognition by embedding 3D manifold information into 2D convolutions. Specifically, the kernel of GeoConv is weighted by our introduced geodesic weights, which are negatively correlated to geodesic distances on a coarsely reconstructed 3D morphable face model. Moreover, based on GeoConv, we further develop an end-to-end trainable framework named GeoCNN for AU recognition.
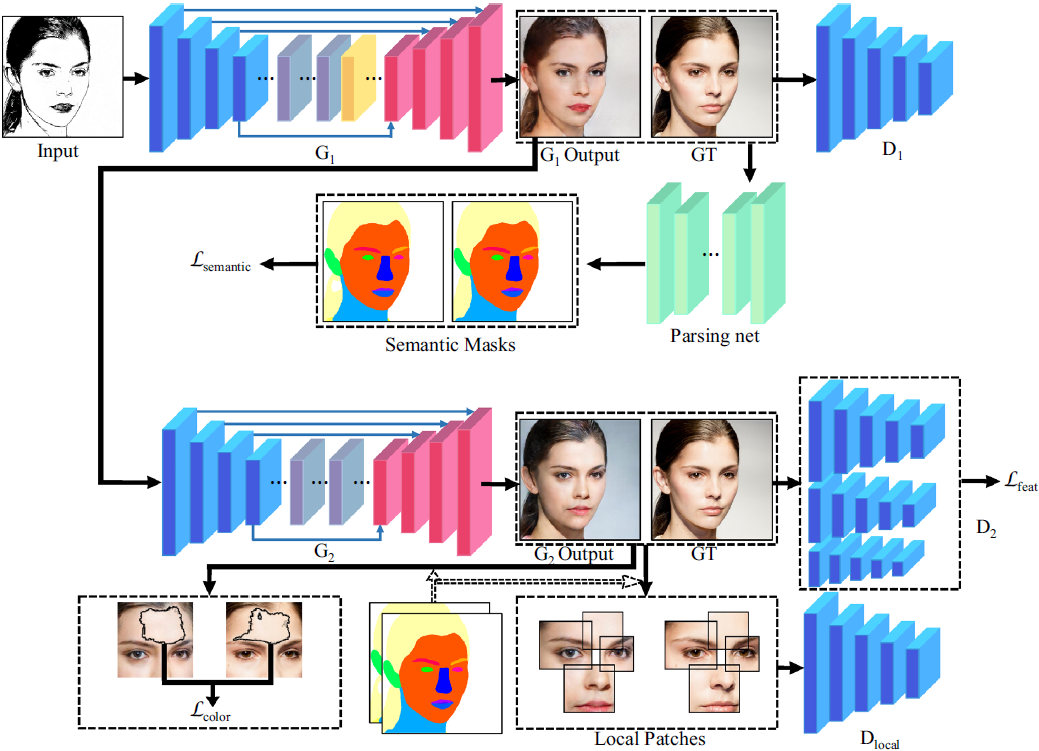
We propose a two-stage sketch-to-photo generative adversarial network for face generation. In the first stage, we propose a semantic loss to maintain semantic consistency. In the second stage, we define the similar connected component and propose a color refinement loss to generate fine-grained details. Moreover, we introduce a multi-scale discriminator and design a patch-level local discriminator. We also propose a texture loss to enhance the local fidelity of synthesized images.
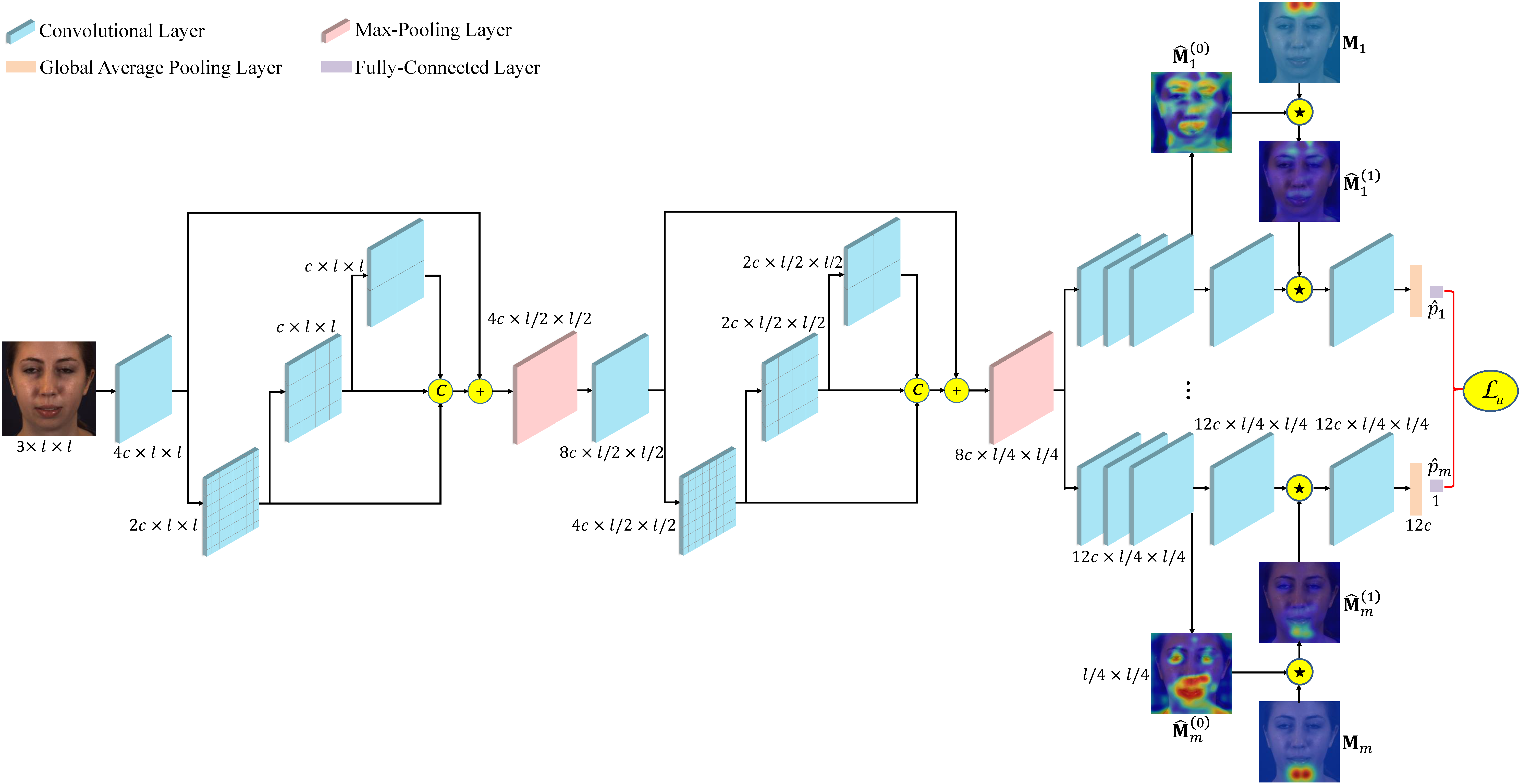
We propose a novel AU recognition method by prior and adaptive attention. Specifically, we predefine a mask for each AU, in which the locations farther away from the AU centers specified by prior knowledge have lower weights. A learnable parameter is adopted to control the importance of different locations. Then, we element-wise multiply the mask by a learnable attention map, and use the new attention map to extract the AU-related feature, in which AU recognition can supervise the adaptive learning of a new attention map.
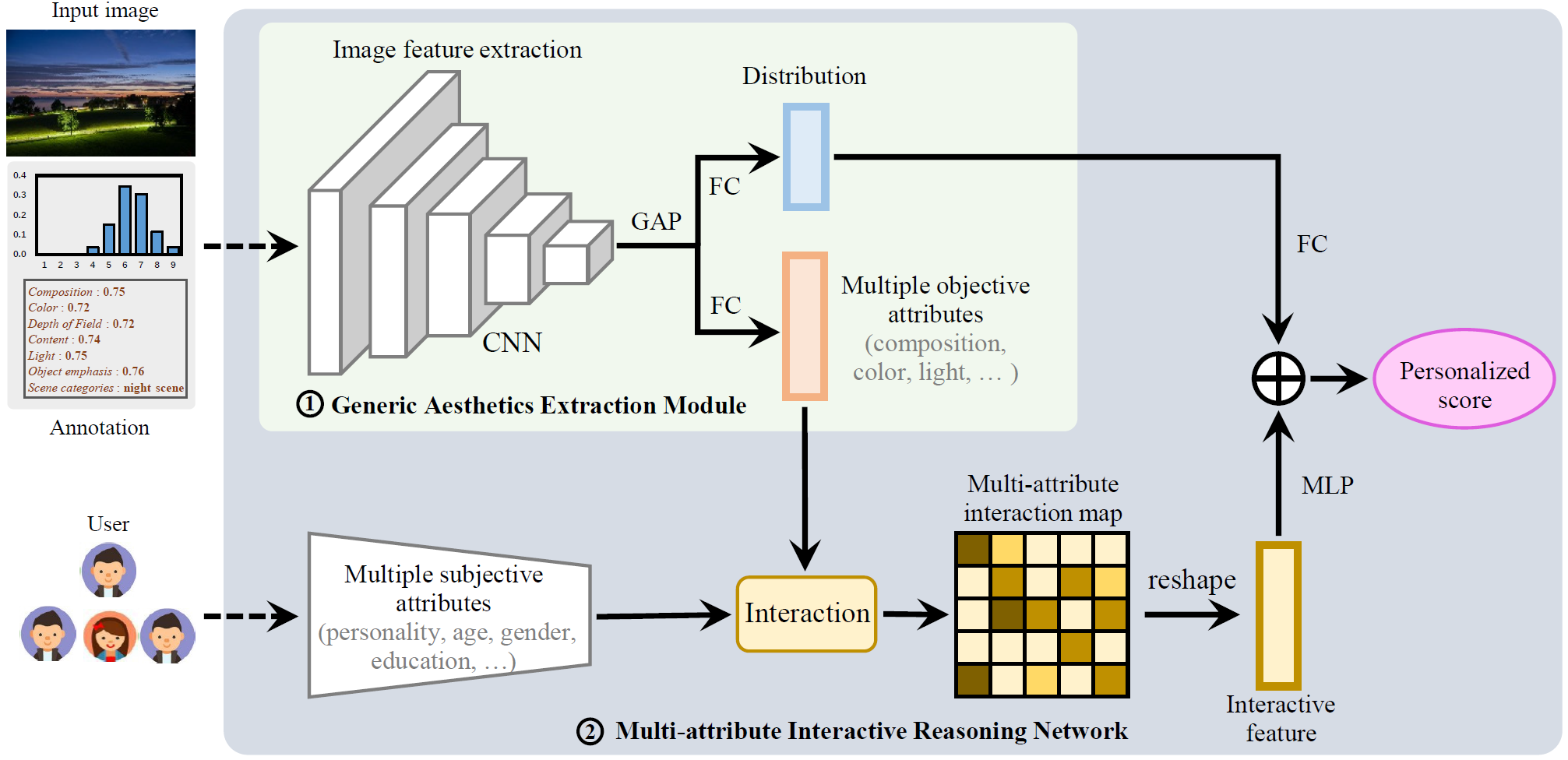
This paper proposes a personalized image aesthetics assessment method via multi-attribute interactive reasoning. Different from existing PIAA models, the multi-attribute interaction constructed from both images and users is used as more effective prior knowledge. First, we designed a generic aesthetics extraction module from the perspective of images to obtain the aesthetic score distribution and multiple objective attributes of images rated by most users. Then, we propose a multi-attribute interactive reasoning network from the perspective of users. By interacting multiple subjective attributes of users with multiple objective attributes of images, we fused the obtained multi-attribute interactive features and aesthetic score distribution to predict personalized aesthetic scores.
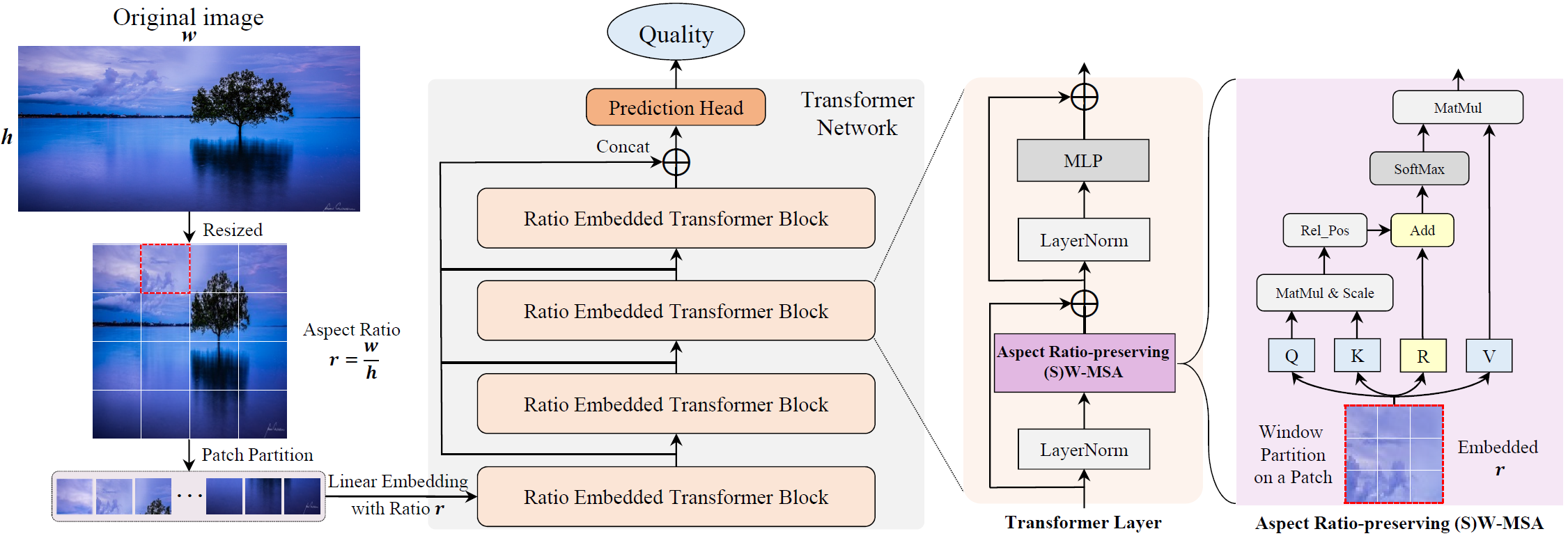
This paper proposes an aspect-ratio-embedded Transformer-based image quality assessment method, which can implant the adaptive aspect ratios of input images into the multihead self-attention module of the Swin Transformer. In this way, the proposed IQA model can not only relieve the variety of perceptual quality caused by size changes in input images but also leverage more global content correlations to infer image perceptual quality. Furthermore, to comprehensively capture the impact of low-level and high-level features on image quality, the proposed IQA model combines the output features of multistage Transformer blocks for jointly inferring image quality.
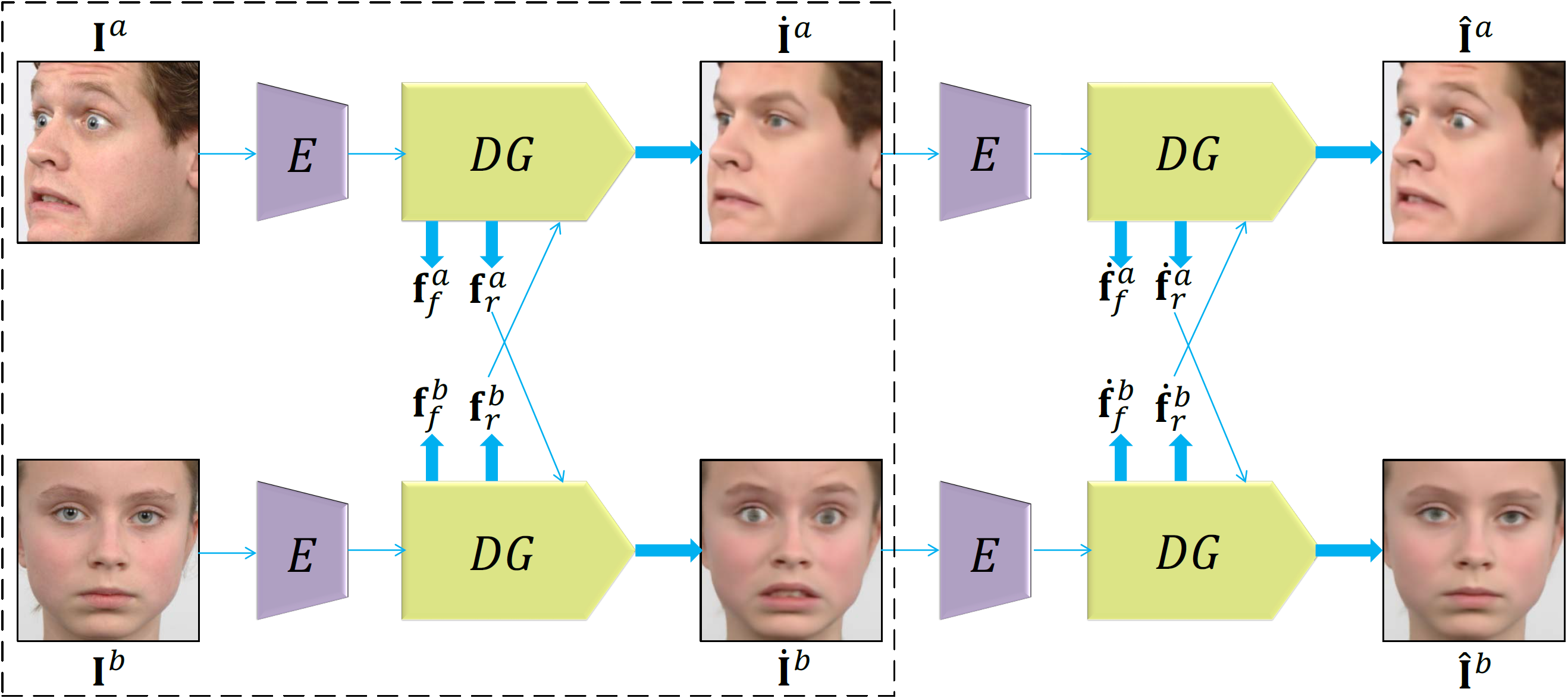
We propose to explicitly transfer facial expression by directly mapping two unpaired input images to two synthesized images with swapped expressions. Specifically, considering AUs semantically describe fine-grained expression details, we propose a novel multi-class adversarial training method to disentangle input images into two types of fine-grained representations: AU-related feature and AU-free feature. Then, we can synthesize new images with preserved identities and swapped expressions by combining AU-free features with swapped AU-related features. Moreover, to obtain reliable expression transfer results of the unpaired input, we introduce a swap consistency loss to make the synthesized images and self-reconstructed images indistinguishable.
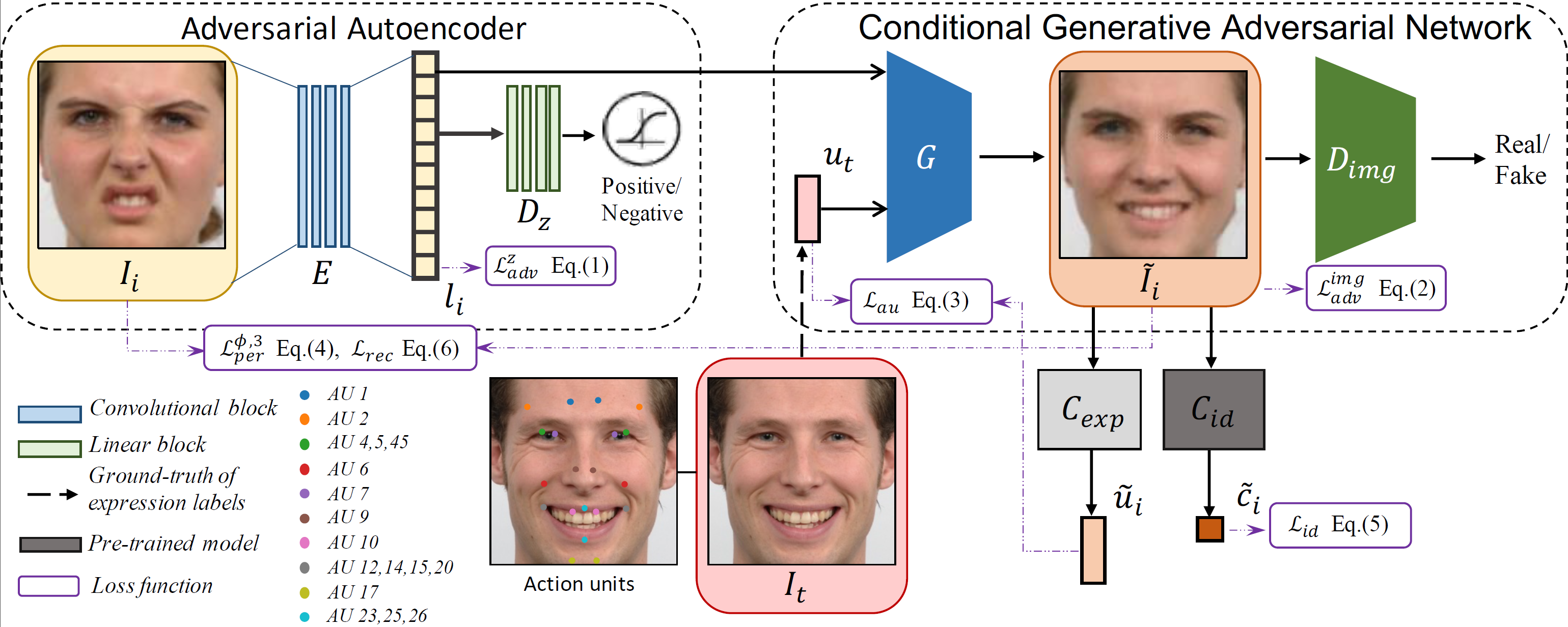
We propose an end-to-end expression-guided generative adversarial network (EGGAN), which synthesizes an image with expected expression given continuous expression label and structured latent code. In particular, an adversarial autoencoder is used to translate a source image into a structured latent space. The encoded latent code and the target expression label are input to a conditional GAN to synthesize an image with the target expression. Moreover, a perceptual loss and a multi-scale structural similarity loss are introduced to preserve facial identity and global shape during expression manipulation.
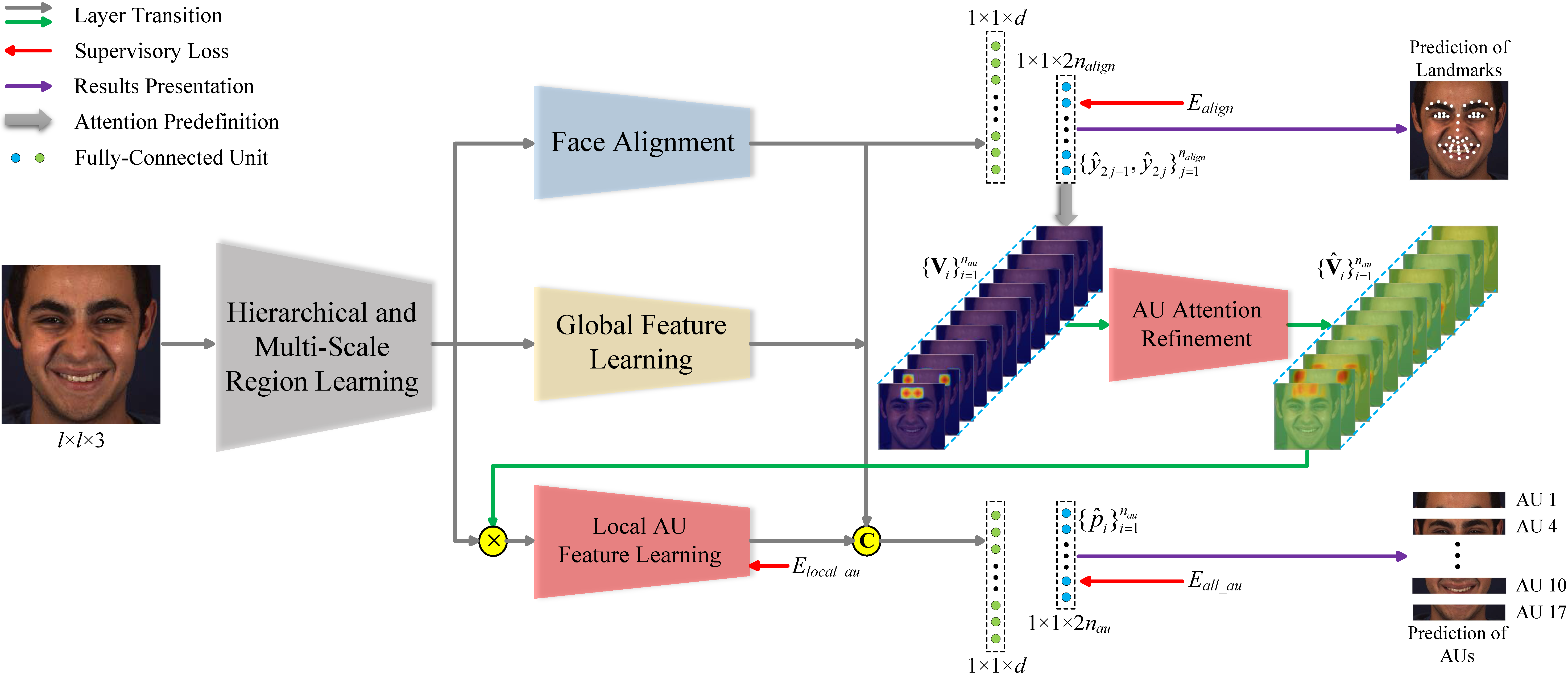
We propose a novel end-to-end deep learning framework for joint AU detection and face alignment, which has not been explored before. In particular, multi-scale shared feature is learned firstly, and high-level feature of face alignment is fed into AU detection. Moreover, to extract precise local features, we propose an adaptive attention learning module to refine the attention map of each AU adaptively. Finally, the assembled local features are integrated with face alignment feature and global feature for AU detection.
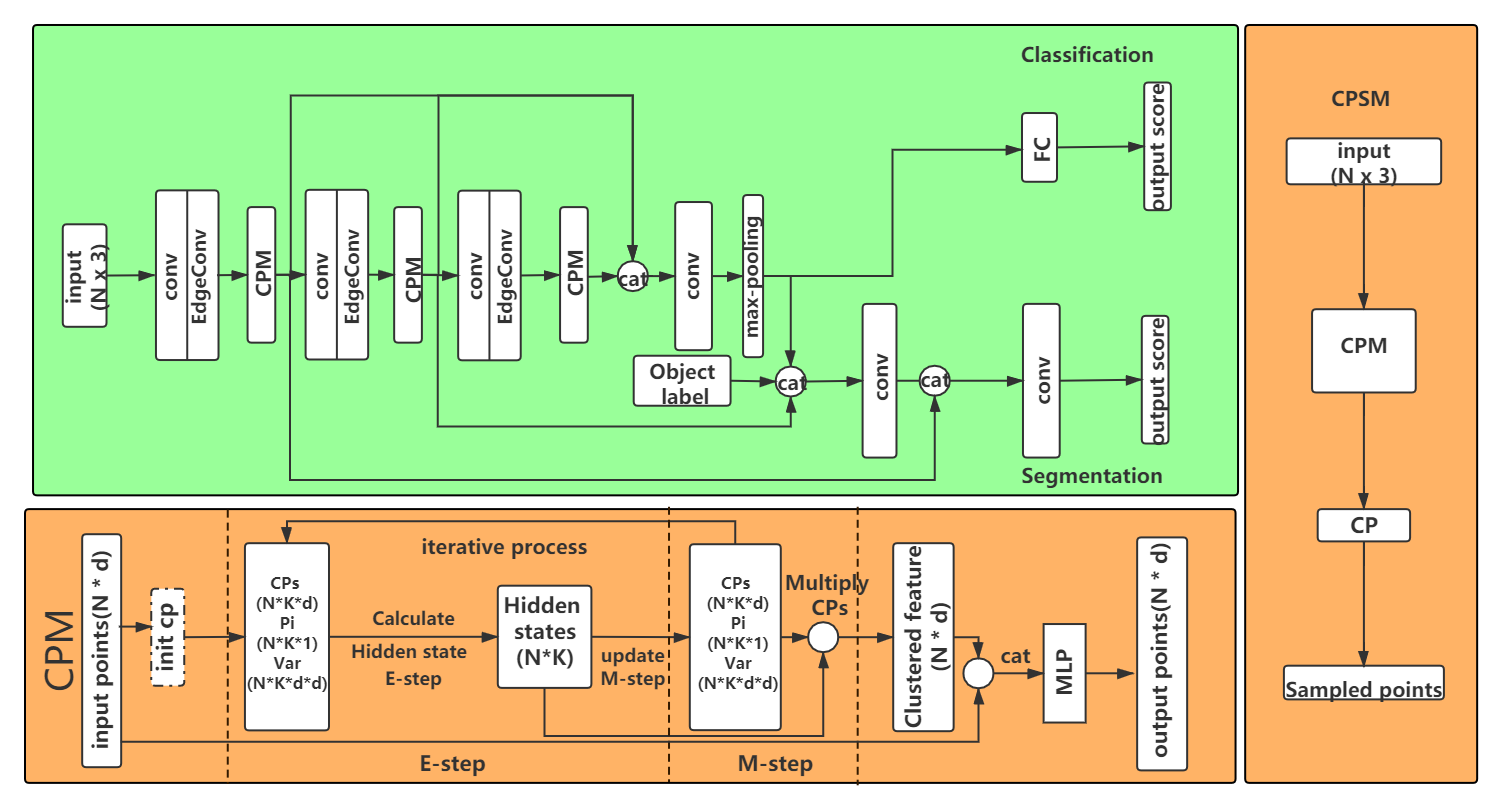
We introduce the Expectation-Maximization Attention module, to find the critical subset points and cluster the other points around them. Moreover, we explore a point cloud sampling strategy to sample points based on the critical subset.
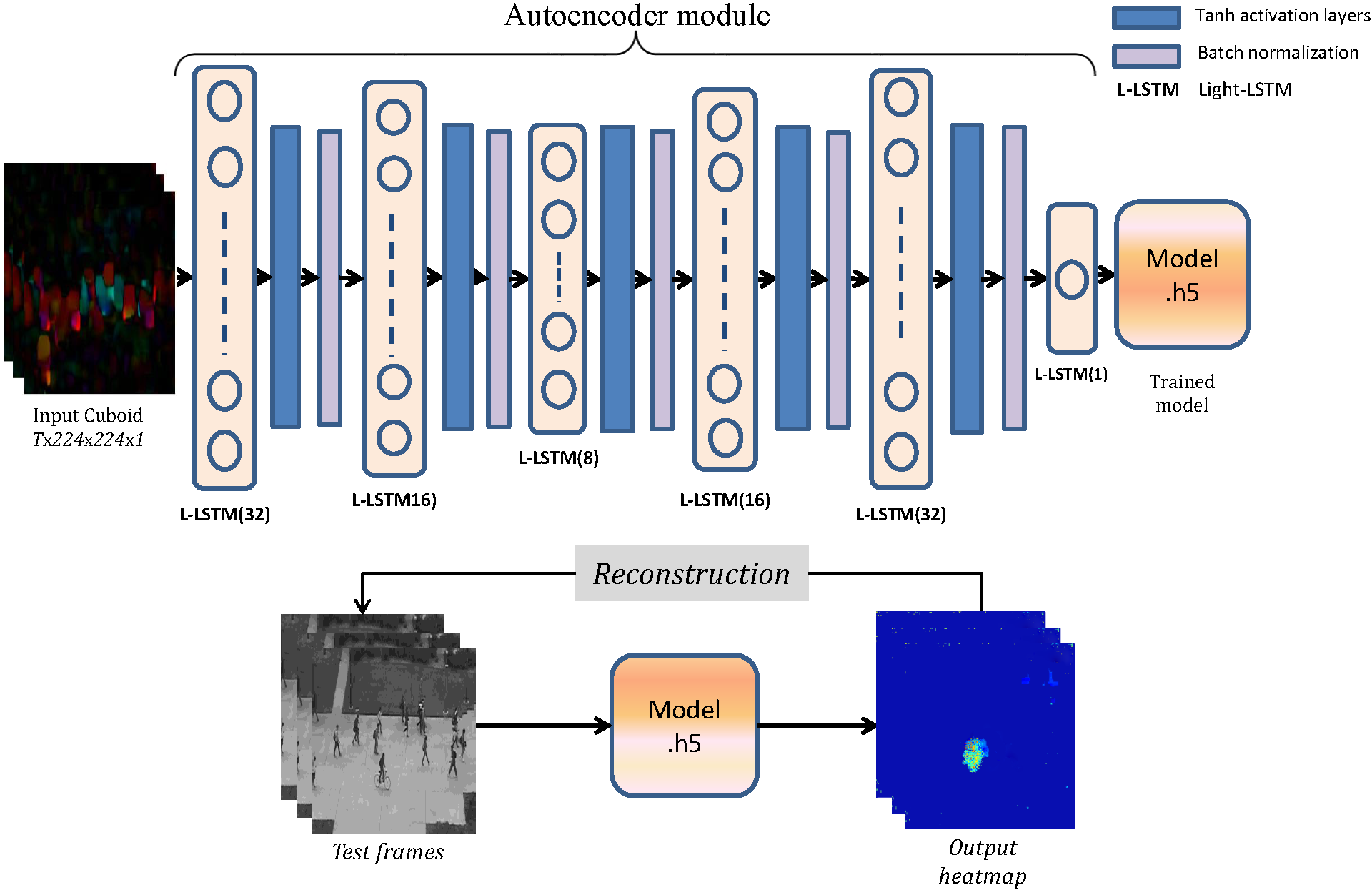
We introduce a bi-gated, light LSTM cell by discarding the forget gate and introducing sigmoid activation. Specifically, the proposed LSTM architecture fully sustains content from previous hidden state thereby enabling the trained model to be robust and make context-independent decision during evaluation. Removing the forget gate results in a simplified and undemanding LSTM cell with improved performance and computational efficiency.
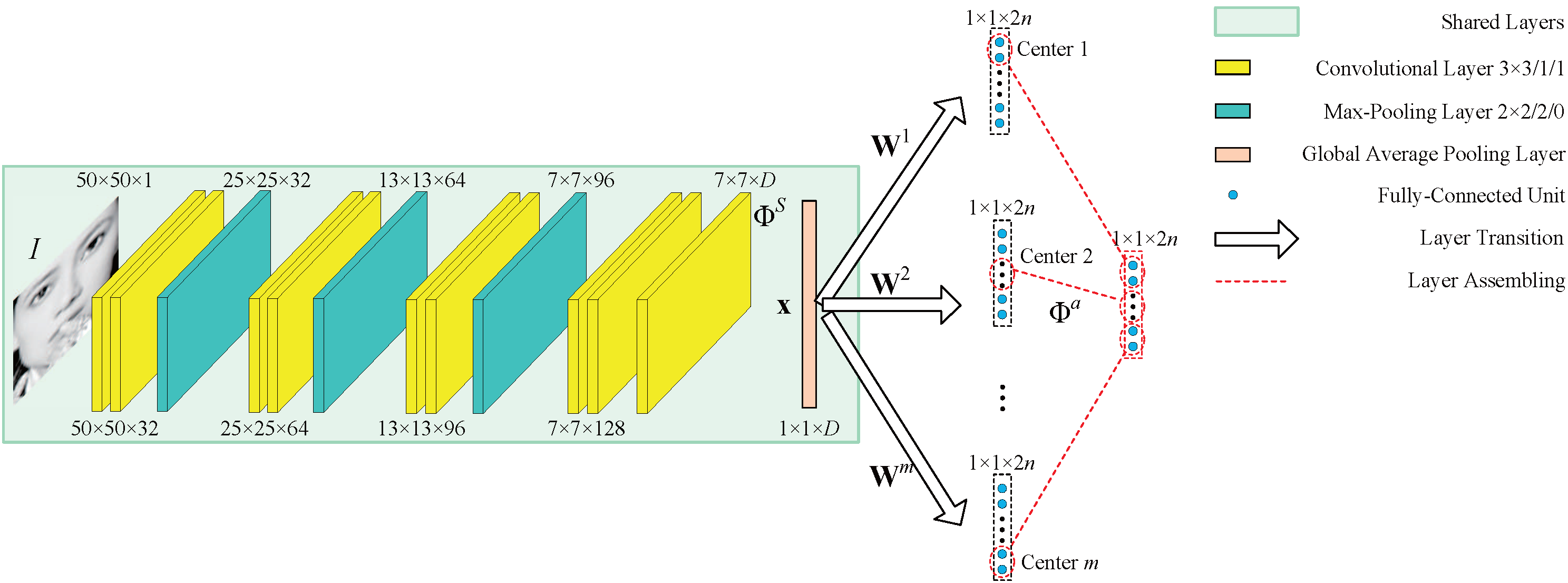
We propose a novel deep learning framework named Multi-Center Learning with multiple shape prediction layers for face alignment. In particular, each shape prediction layer emphasizes on the detection of a certain cluster of semantically relevant landmarks respectively. Challenging landmarks are focused firstly, and each cluster of landmarks is further optimized respectively. Moreover, to reduce the model complexity, we propose a model assembling method to integrate multiple shape prediction layers into one shape prediction layer.
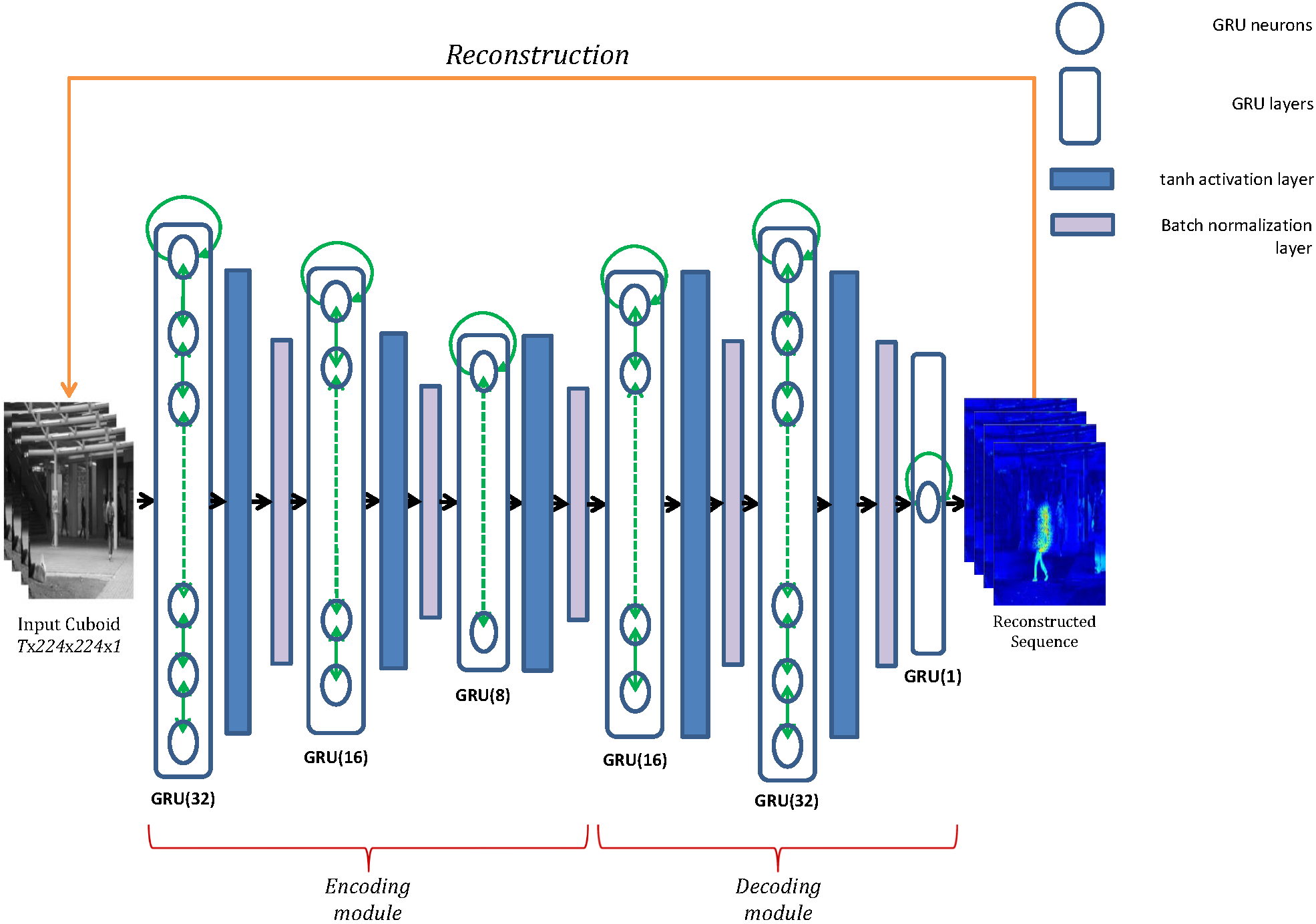
We propose a novel version of Gated Recurrent Unit (GRU), called Single-Tunnelled GRU for abnormality detection. Particularly, the Single-Tunnelled GRU discards the heavy-weighted reset gate from GRU cells that overlooks the importance of past content by only favouring current input to obtain an optimized single-gated-cell model. Moreover, we substitute the hyperbolic tangent activation in standard GRUs with sigmoid activation, as the former suffers from performance loss in deeper networks.
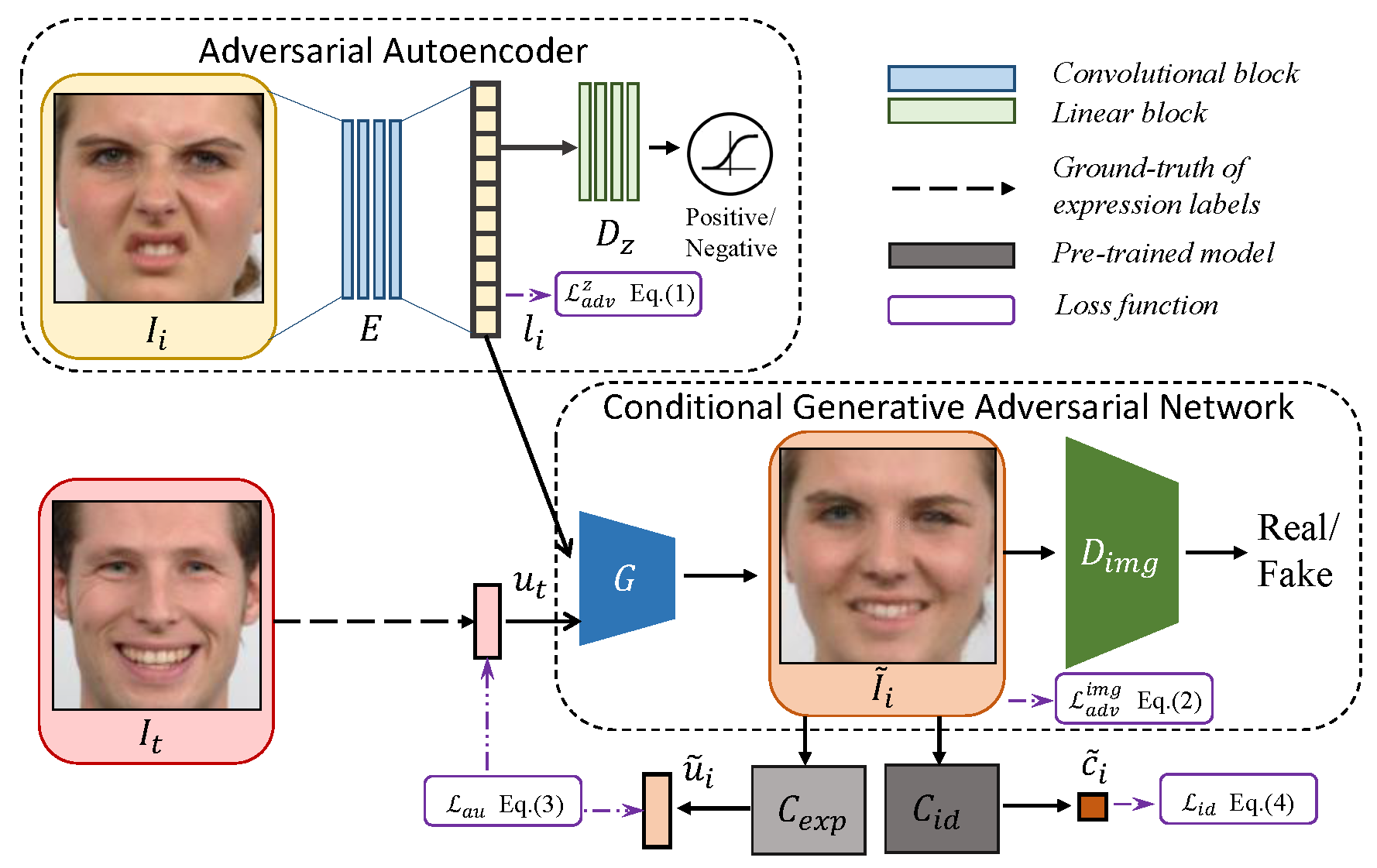
We propose an end-to-end expression-guided generative adversarial network (EGGAN), which utilizes structured latent codes and continuous expression labels as input to generate images with expected expressions. Specifically, we adopt an adversarial autoencoder to map a source image into a structured latent space. Then, given the source latent code and the target expression label, we employ a conditional GAN to generate a new image with the target expression. Moreover, we introduce a perceptual loss and a multi-scale structural similarity loss to preserve identity and global shape during generation.
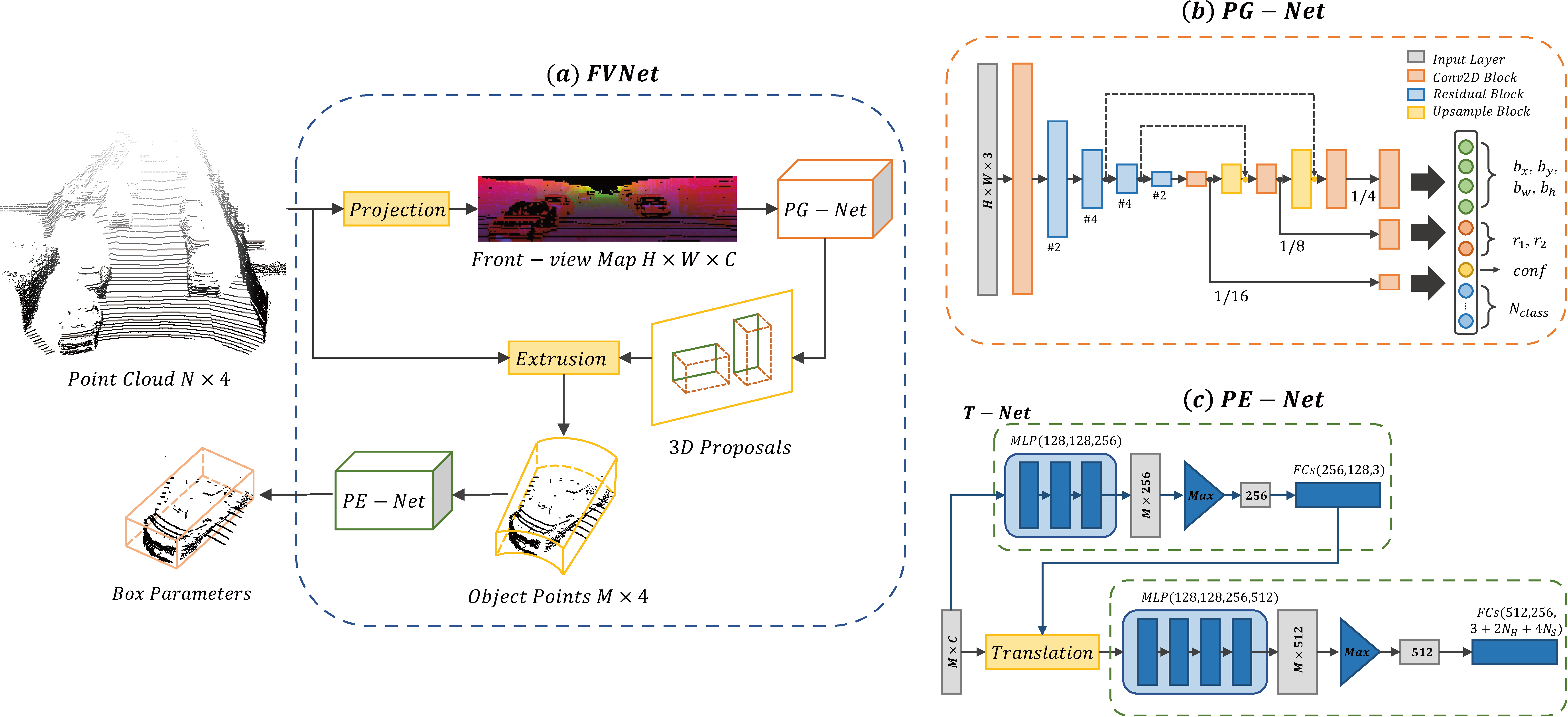
We propose a novel framework called FVNet for 3D front-view proposal generation and object detection from point clouds. It consists of two stages: generation of front-view proposals and estimation of 3D bounding box parameters. We first project point clouds onto a cylindrical surface to generate front-view feature maps which retains rich information. We then introduce a proposal generation network to predict 3D region proposals from the generated maps and further extrude objects of interest from the whole point cloud. Finally, we present another network to extract the point-wise features from the extruded object points and regress the final 3D bounding box parameters in the canonical coordinates.
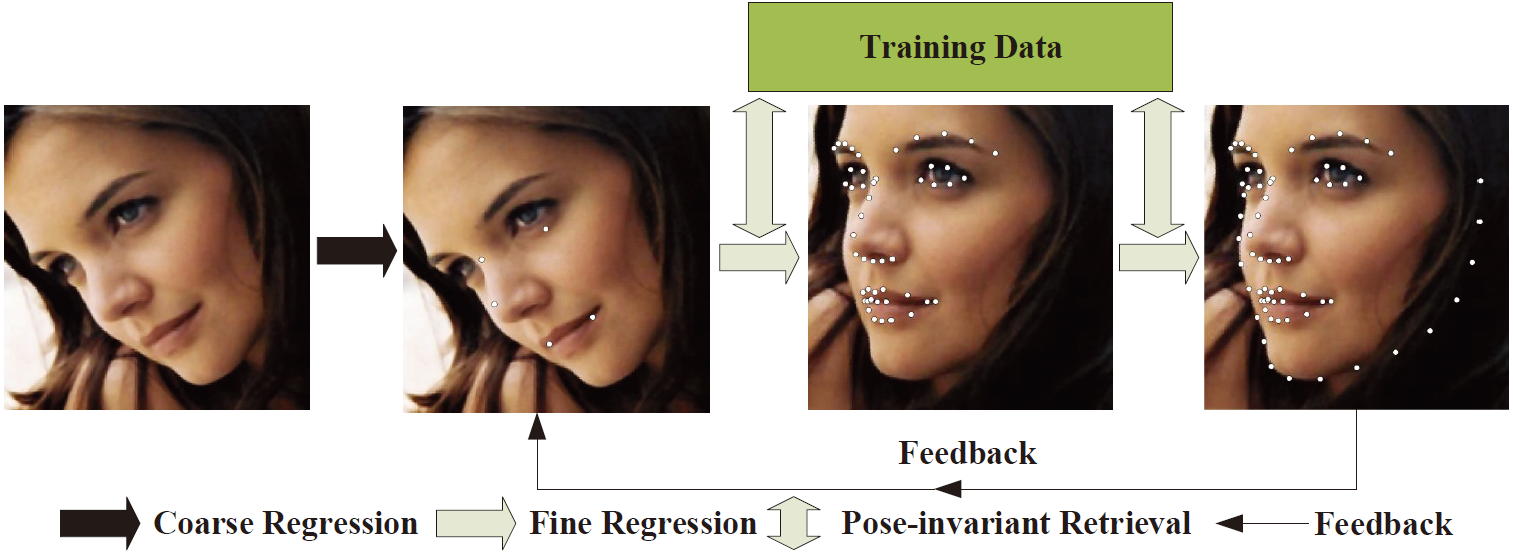
We propose a new pipeline of salient-to-inner-to-all to progressively compute the locations of landmarks. Additionally, a feedback process is utilised to improve the robustness of regression. They bring out a pose-invariant shape retrieval method to generate the discriminative initialisation.
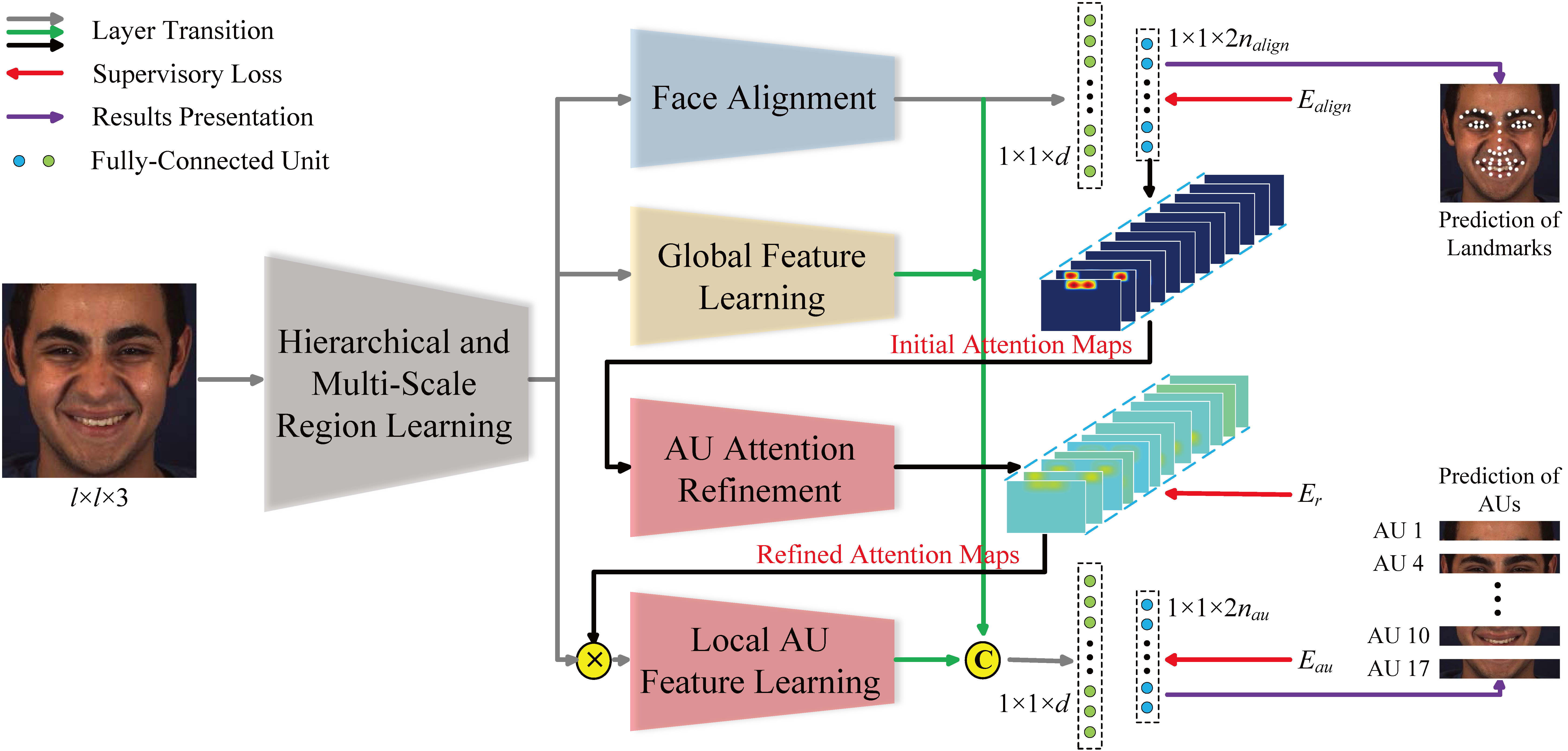
We propose a novel end-to-end deep learning framework for joint AU detection and face alignment, which has not been explored before. In particular, multi-scale shared features are learned firstly, and high-level features of face alignment are fed into AU detection. Moreover, to extract precise local features, we propose an adaptive attention learning module to refine the attention map of each AU adaptively. Finally, the assembled local features are integrated with face alignment features and global features for AU detection.
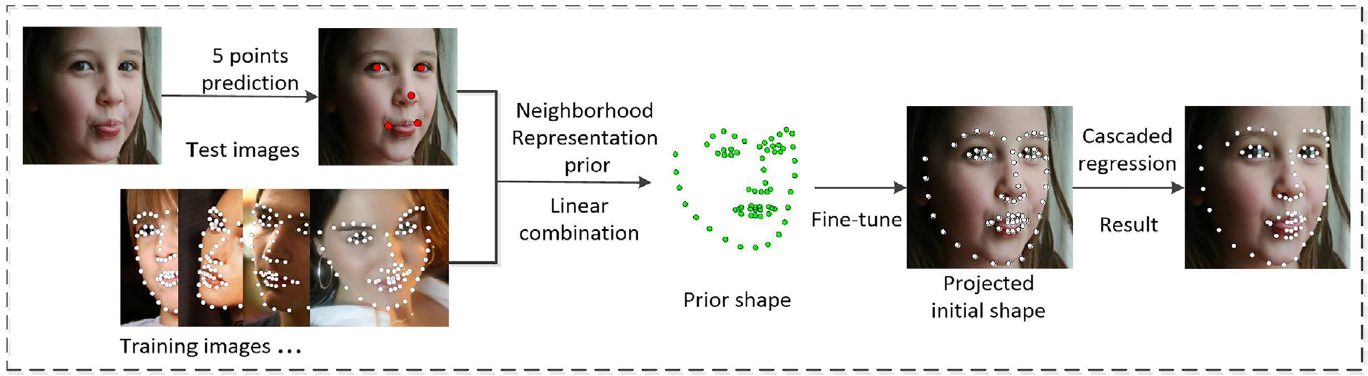
We discuss how to improve initialization by studying a neighborhood representation prior, leveraging neighboring faces to obtain a high-quality initial shape. In order to further improve the estimation precision of each facial landmark, we propose a face-like landmark adjustment algorithm to refine the face shape.
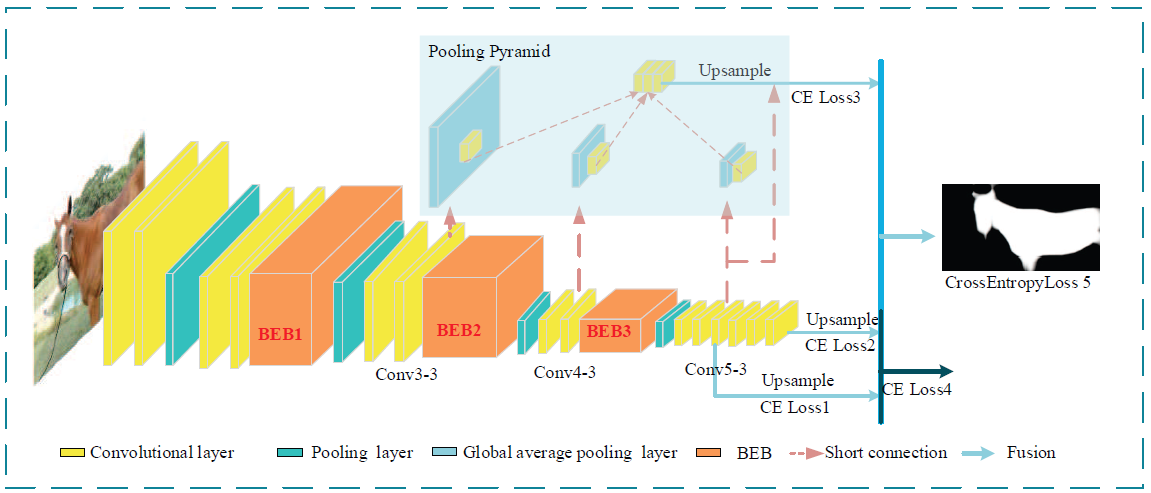
We propose to embed the boundary enhancement block (BEB) into the network to refine edge. It keeps the details by the mutual-coupling convolutional layers. Besides, we employ a pooling pyramid that utilizes the multi-level feature informations to search global context, and it also contributes as an auxiliary supervision. The final saliency map is obtained by fusing the edge refinement with global context extraction.
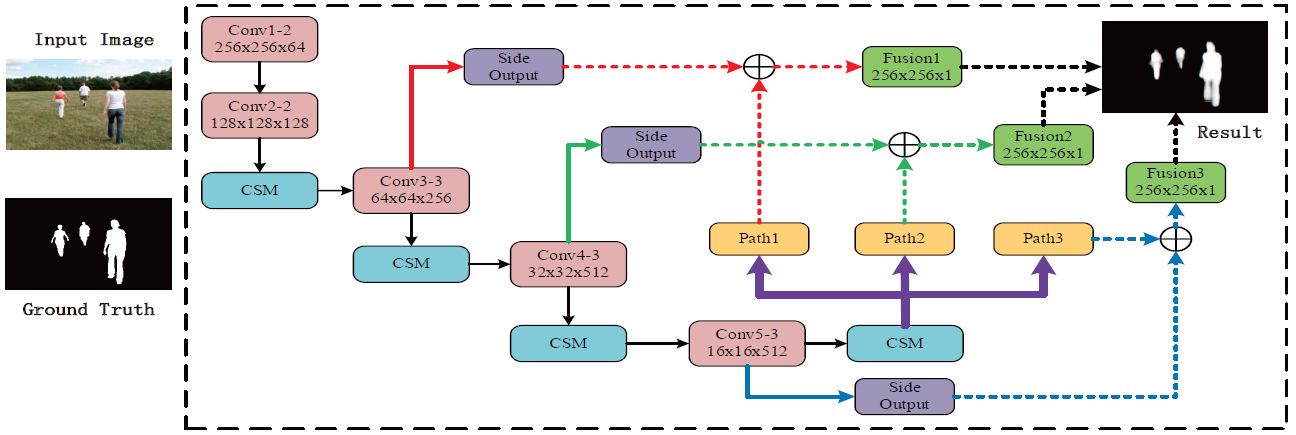
We exploit a multi-path feature fusion model for saliency detection. The proposed model is a fully convolutional network with raw images as input and saliency maps as output. In particular, we propose a multi-path fusion strategy for deriving the intrinsic features of salient objects. The structure has the ability of capturing the low-level visual features and generating the boundary-preserving saliency maps. Moreover, a coupled structure module is proposed in our model, which helps to explore the high-level semantic properties of salient objects.
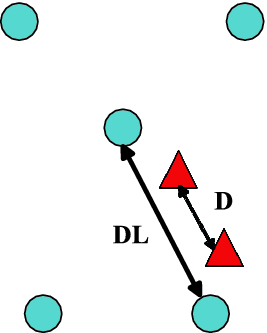
We propose a two-stage cascade regression framework using patch-difference features to overcome the above problem. In the first stage, by applying the patch-difference feature and augmenting the large pose samples to the classical shape regression model, salient landmarks (eye centers, nose, mouth corners) can be located precisely. In the second stage, by applying enhanced feature section constraint to the patch-difference feature, multi-landmark detection is achieved.
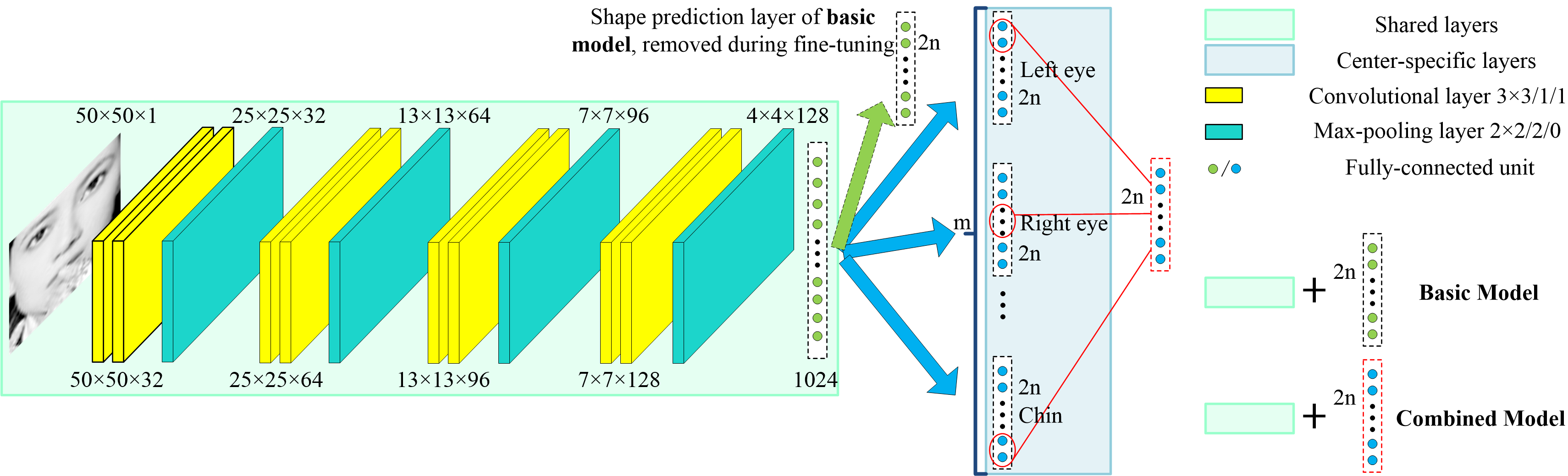
We propose a novel multi-center convolutional neural network for unconstrained face alignment. To utilize structural correlations among different facial landmarks, we determine several clusters based on their spatial position. We pre-train our network to learn generic feature representations. We further fine-tune the pre-trained model to emphasize on locating a certain cluster of landmarks respectively. Fine-tuning contributes to searching an optimal solution smoothly without deviating from the pre-trained model excessively. We obtain an excellent solution by combining multiple fine-tuned models.
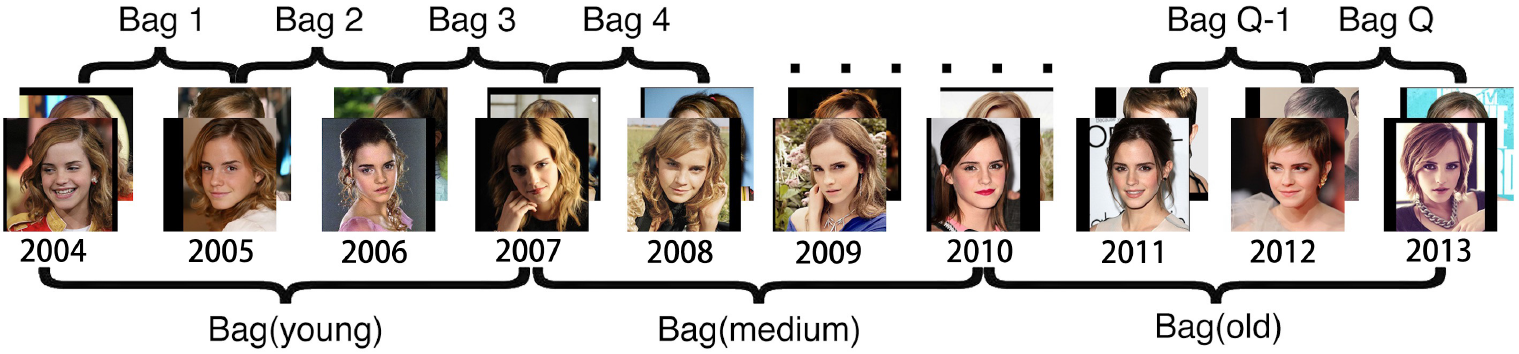
We propose a deep feature based framework for face retrieval problem. Our framework uses deep CNNs feature descriptor and two well designed post-processing methods to achieve age-invariance. To the best of our knowledge, this is the first deep feature based method in cross-age face retrieval problem.
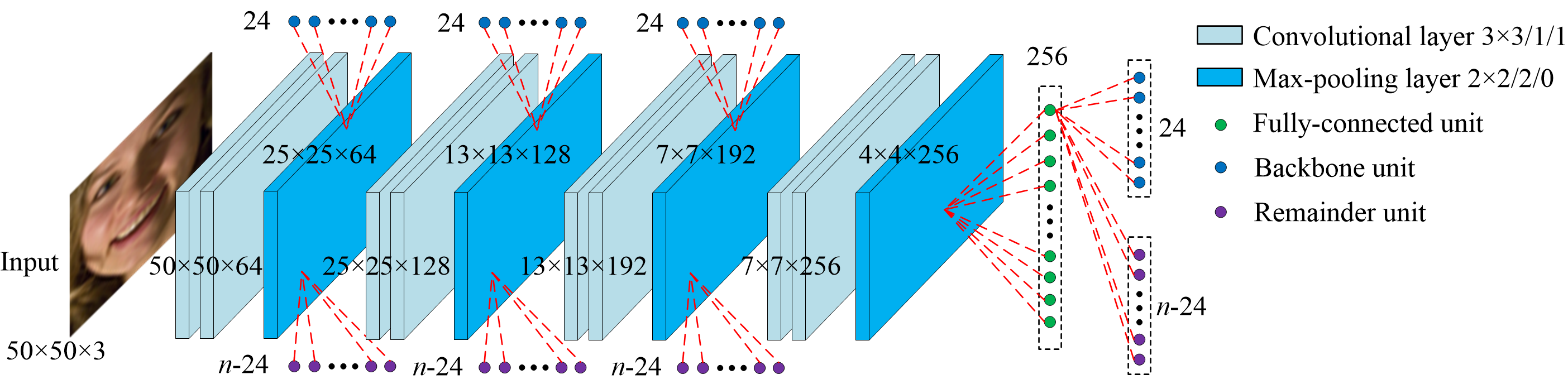
We propose a novel face alignment method that trains deep convolutional network from coarse to fine. It divides given landmarks into principal subset and elaborate subset. We firstly keep a large weight for principal subset to make our network primarily predict their locations while slightly take elaborate subset into account. Next the weight of principal subset is gradually decreased until two subsets have equivalent weights. This process contributes to learn a good initial model and search the optimal model smoothly to avoid missing fairly good intermediate models in subsequent procedures.
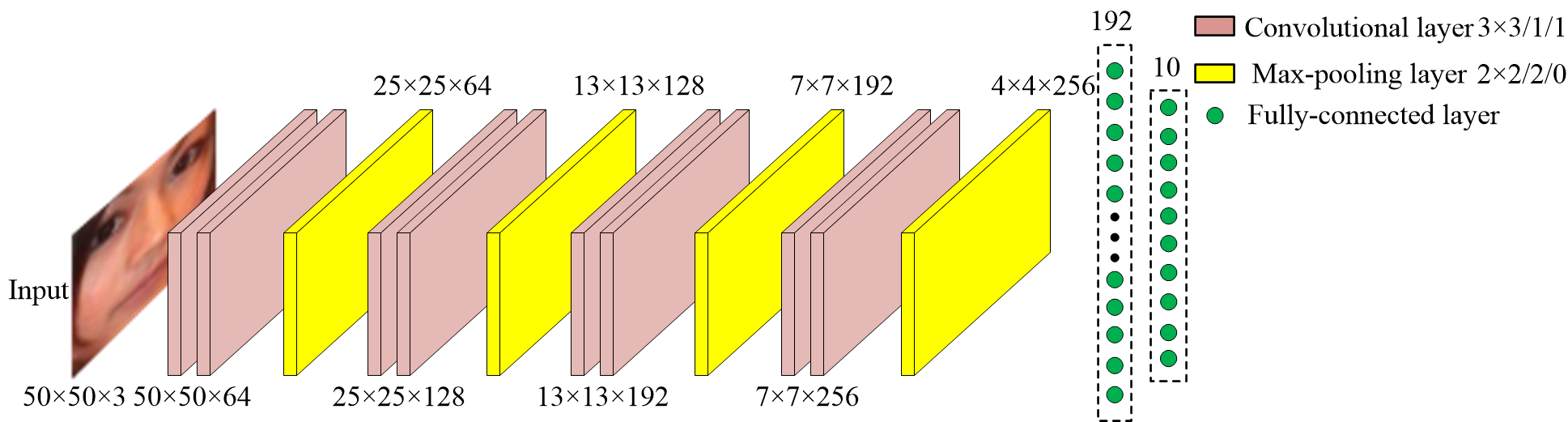
We propose a novel data augmentation strategy. And we design an innovative training algorithm with adaptive learning rate for two iterative procedures, which helps the network to search an optimal solution. Our convolutional network can learn global high-level features and directly predict the coordinates of facial landmarks.
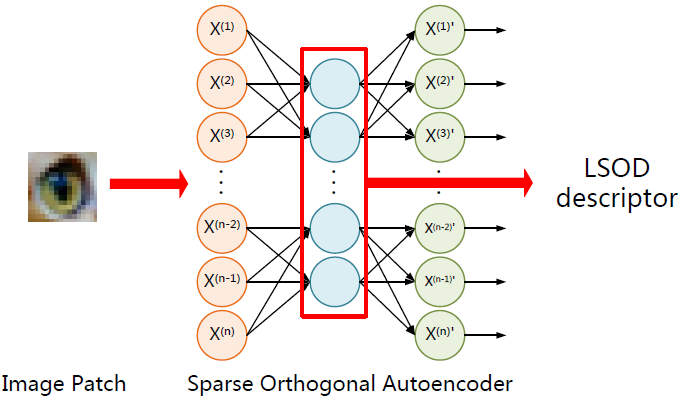
We propose a novel method for feature description used for image matching in this paper. Our method is inspired by the autoencoder, an artificial neural network designed for learning efficient codings. Sparse and orthogonal constraints are imposed on the autoencoder and make it a highly discriminative descriptor. It is shown that the proposed descriptor is not only invariant to geometric and photometric transformations (such as viewpoint change, intensity change, noise, image blur and JPEG compression), but also highly efficient.