Biography
He is now an Associate Professor and a Graduate Advisor at the School of Computer Science and Technology, China University of Mining and Technology (CUMT), as well as a Postdoctoral Fellow at the Department of Computer Science and Engineering, Hong Kong University of Science and Technology (HKUST) and the Department of Computer Science and Engineering, Shanghai Jiao Tong University (SJTU). He received the Ph.D. degree in Computer Science and Technology from the SJTU in Aug. 2020, and works as a postdoctoral fellow at the SJTU since Dec. 2022, both with Prof. Lizhuang Ma as the advisor. Also, he works as a postdoctoral fellow at the HKUST since Jan. 2024, advised by Prof. Dit-Yan Yeung. From Nov. 2017 to Nov. 2018, he was a joint Ph.D. student at the Multimedia and Interactive Computing Lab, Nanyang Technological University (NTU), advised by Prof. Jianfei Cai. Before that, he received the B.Eng. degree in Computer Science and Technology from the Northwestern Polytechnical University (NPU) in Jul. 2015. He has been sponsored with fundings such as Hong Kong Scholars Program, Young Scientists Fund of the National Natural Science Foundation of China, High-Level Talent Program for Innovation and Entrepreneurship (ShuangChuang Doctor) of Jiangsu Province, and Talent Program for Deputy General Manager of Science and Technology of Jiangsu Province. He has published more than 40 academic papers in popular journals and conferences. He has been serving as an area chair for ACM MM 2024, a publication chair for CGI 2023, as well as a program committee member or a reviewer in top journals and conferences such as IEEE TPAMI / TIP, IJCV, CVPR, ICCV, IJCAI, AAAI, and ACM MM. His research interests lie in the fields of Computer Vision and Deep Learning. The official faculty websites can be found here and here. [ Résumé ]
News
Jan. 2024: I serve as an Area Chair in ACM International Conference on Multimedia (MM) 2024.
Jan. 2024: I am now studying at Hong Kong University of Science and Technology (HKUST) as a postdoctoral fellow, advised by Prof. Dit-Yan Yeung.
Aug. 2023: I am a recipient of Hong Kong Scholars Program.
July 2023: Our paper CT-Net: Arbitrary-Shaped Text Detection via Contour Transformer is accepted by IEEE TCSVT.
June 2023: I am a recipient of General Project of China Postdoctoral Science Foundation.
May 2023: Our paper Facial Action Unit Detection via Adaptive Attention and Relation is accepted by IEEE TIP.
Jan. 2023: I am a recipient of Outstanding Young Teacher Program of China University of Mining and Technology.
Jan. 2023: I serve as a Publication Chair in Computer Graphics International (CGI) 2023.
Dec. 2022: Our paper Facial Action Unit Detection Using Attention and Relation Learning becomes an ESI highly cited paper.
July 2022: I am a recipient of Talent Program for Deputy General Manager of Science and Technology of Jiangsu Province.
June 2022: Our paper TextDCT: Arbitrary-Shaped Text Detection via Discrete Cosine Transform Mask is accepted by IEEE TMM.
Aug. 2021: I am a recipient of Young Scientists Fund of the National Natural Science Foundation of China.
July 2021: I am a recipient of High-Level Talent Program for Innovation and Entrepreneurship (ShuangChuang Doctor) of Jiangsu Province.
June 2021: Our paper Unconstrained Facial Action Unit Detection via Latent Feature Domain is accepted by IEEE TAFFC.
Apr. 2021: Our paper Explicit Facial Expression Transfer via Fine-Grained Representations is accepted by IEEE TIP.
Sept. 2020: I serve as a PC member in AAAI 2021 and IJCAI 2021.
Aug. 2020: Our paper JÂA-Net: Joint Facial Action Unit Detection and Face Alignment via Adaptive Attention is accepted by IJCV.
Oct. 2019: Our paper Facial Action Unit Detection Using Attention and Relation Learning is accepted by IEEE TAFFC.
July 2018: Our paper Deep Adaptive Attention for Joint Facial Action Unit Detection and Face Alignment is accepted by ECCV 2018.
Nov. 2017: I am now studying at Multimedia and Interactive Computing Lab of Nanyang Technological University (NTU), Singapore as a research assistant, advised by Prof. Jianfei Cai.
Timeline
Sponsored Projects
2023: Hong Kong Scholars Program, Principal Investigator
2023: Opening Fund of Key Laboratory of Image Processing and Intelligent Control (Huazhong University of Science and Technology), Ministry of Education, Principal Investigator
2023: General Project of China Postdoctoral Science Foundation, Principal Investigator
2023: Outstanding Young Teacher Program of China University of Mining and Technology, Principal Investigator
2022: Participation in Computer Graphics International (CGI) 2022 Supported by K.C.Wong Education Foundation, Principal Investigator
2022: Patent License Project for Method and Device of Facial Action Unit Recognition Based on Joint Learning and Optical Flow Estimation, Principal Investigator
2022: Talent Program for Deputy General Manager of Science and Technology of Jiangsu Province, Principal Investigator
2021: Young Scientists Fund of the National Natural Science Foundation of China, Principal Investigator
2021: High-Level Talent Program for Innovation and Entrepreneurship (ShuangChuang Doctor) of Jiangsu Province, Principal Investigator
2021: Young Scientists Fund of the Fundamental Research Funds for the Central Universities, Principal Investigator
2020: Start-Up Grant of China University of Mining and Technology, Principal Investigator
Selected Publications
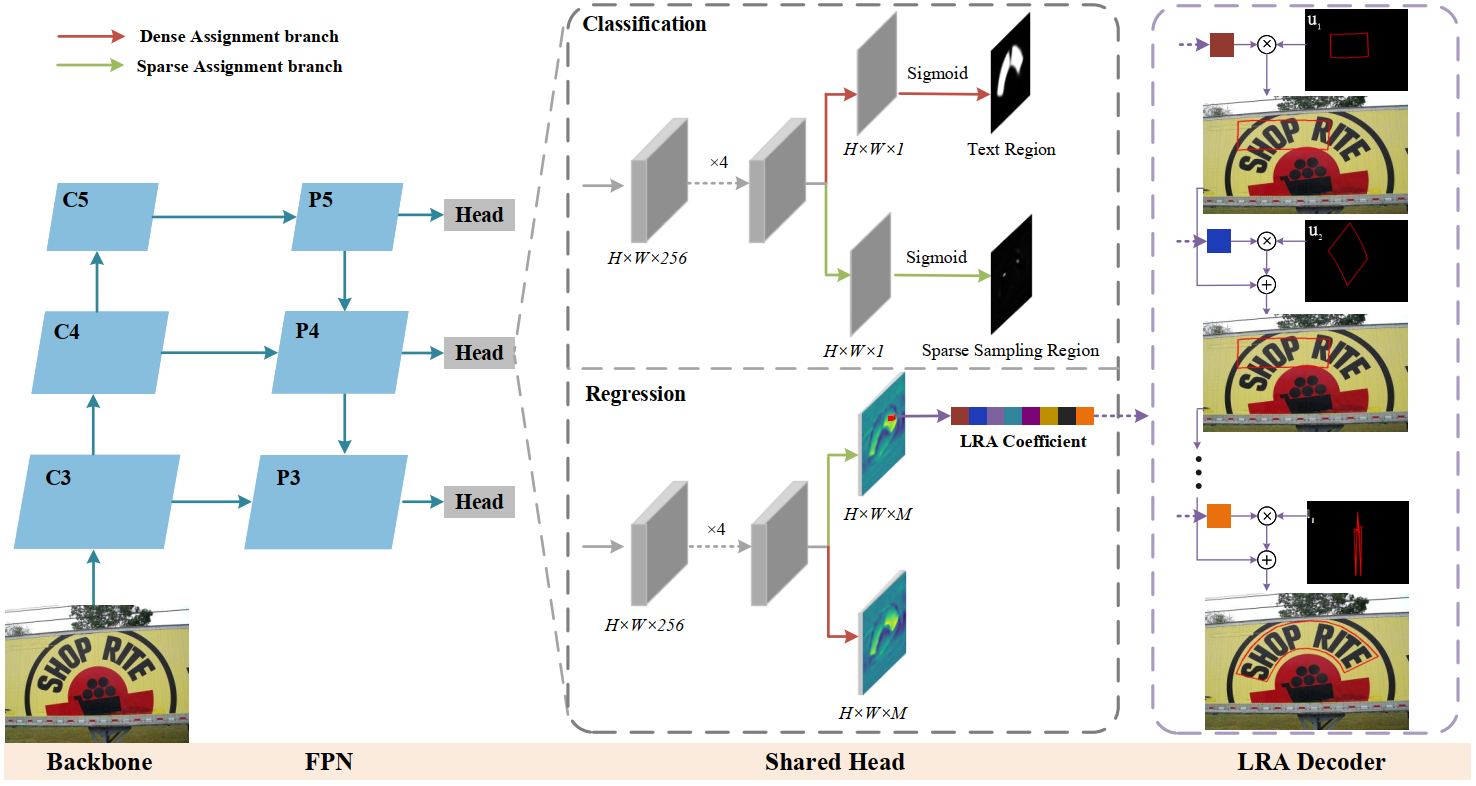
We first propose a novel parameterized text shape method based on low-rank approximation. Unlike other shape representation methods that employ data-irrelevant parameterization, our approach utilizes singular value decomposition and reconstructs the text shape using a few eigenvectors learned from labeled text contours. By exploring the shape correlation among different text contours, our method achieves consistency, compactness, simplicity, and robustness in shape representation. Next, we propose a dual assignment scheme for speed acceleration. It adopts a sparse assignment branch to accelerate the inference speed, and meanwhile, provides ample supervised signals for training through a dense assignment branch. Building upon these designs, we implement an accurate and efficient arbitrary-shaped text detector named LRANet.
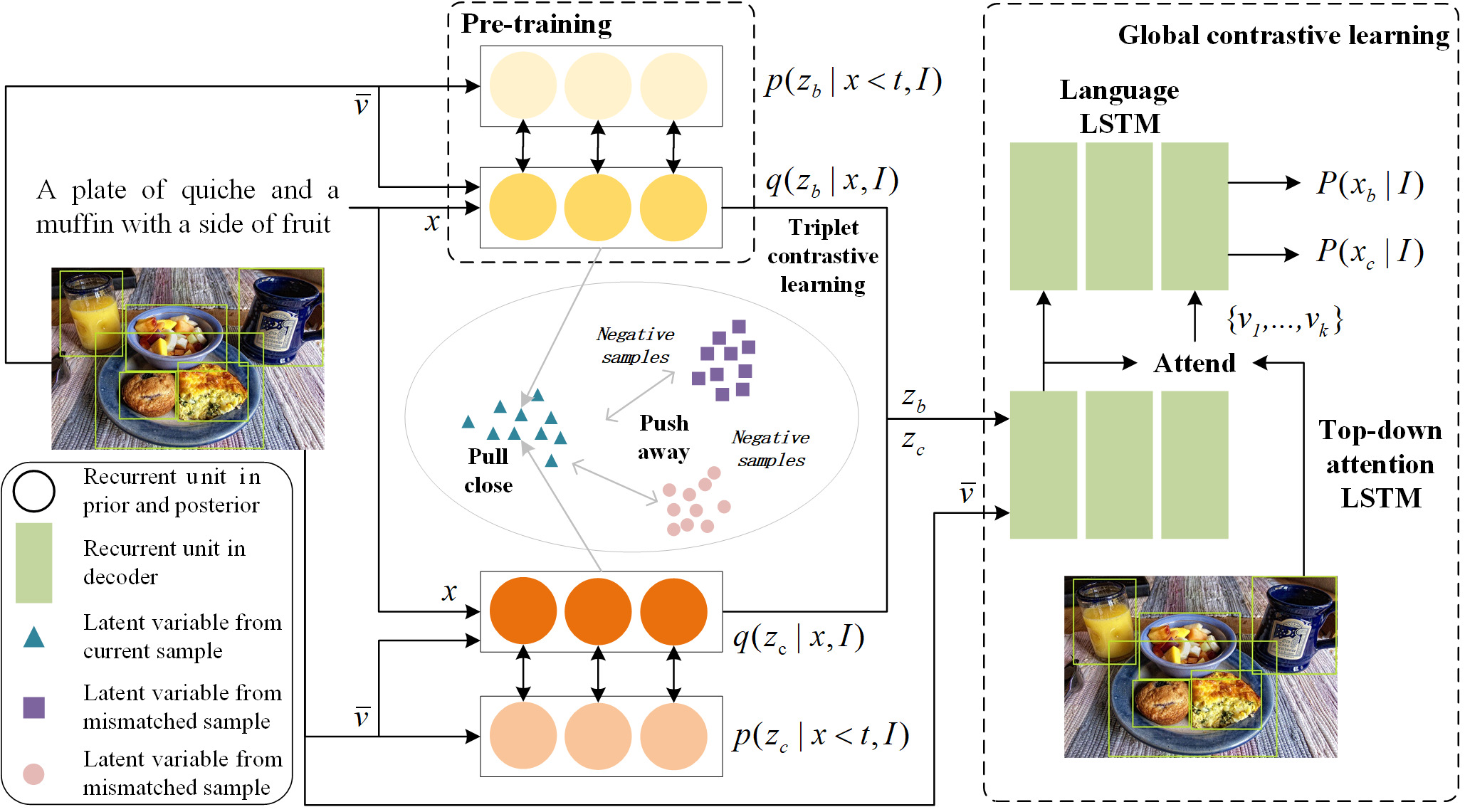
We propose a novel Conditional Variational Autoencoder (DCL-CVAE) framework for diverse image captioning by seamlessly integrating sequential variational autoencoder with contrastive learning. In the encoding stage, we first build conditional variational autoencoders to separately learn the sequential latent spaces for a pair of captions. Then, we introduce contrastive learning in the sequential latent spaces to enhance the discriminability of latent representations for both image-caption pairs and mismatched pairs. In the decoding stage, we leverage the captions sampled from the pre-trained Long Short-Term Memory (LSTM), LSTM decoder as the negative examples and perform contrastive learning with the greedily sampled positive examples, which can restrain the generation of common words and phrases induced by the cross entropy loss. By virtue of dual constrastive learning, DCL-CVAE is capable of encouraging the discriminability and facilitating the diversity, while promoting the accuracy of the generated captions.
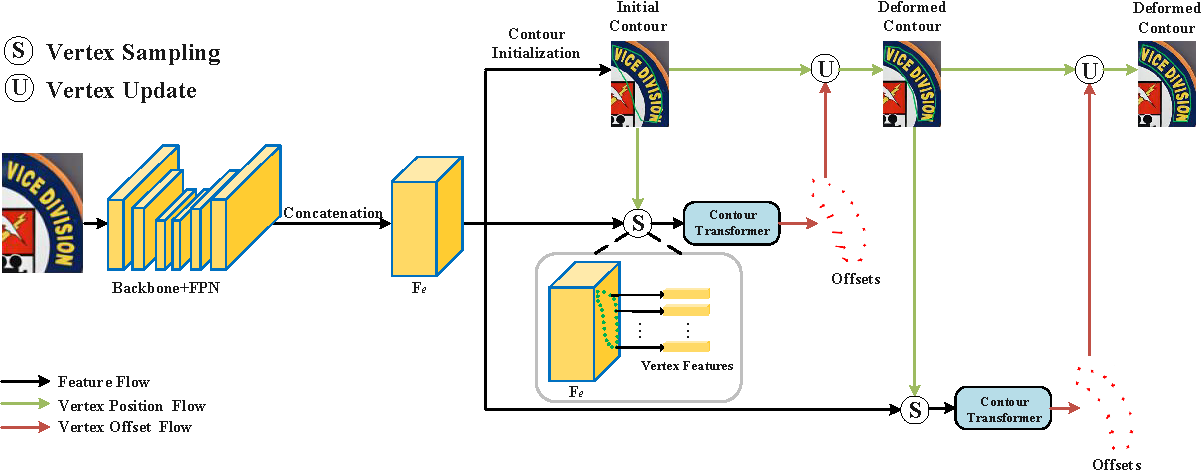
We propose a novel arbitrary-shaped scene text detection framework named CT-Net by progressive contour regression with contour transformers. Specifically, we first employ a contour initialization module that generates coarse text contours without any post-processing. Then, we adopt contour refinement modules to adaptively refine text contours in an iterative manner, which are beneficial for context information capturing and progressive global contour deformation. Besides, we propose an adaptive training strategy to enable the contour transformers to learn more potential deformation paths, and introduce a re-score mechanism that can effectively suppress false positives.
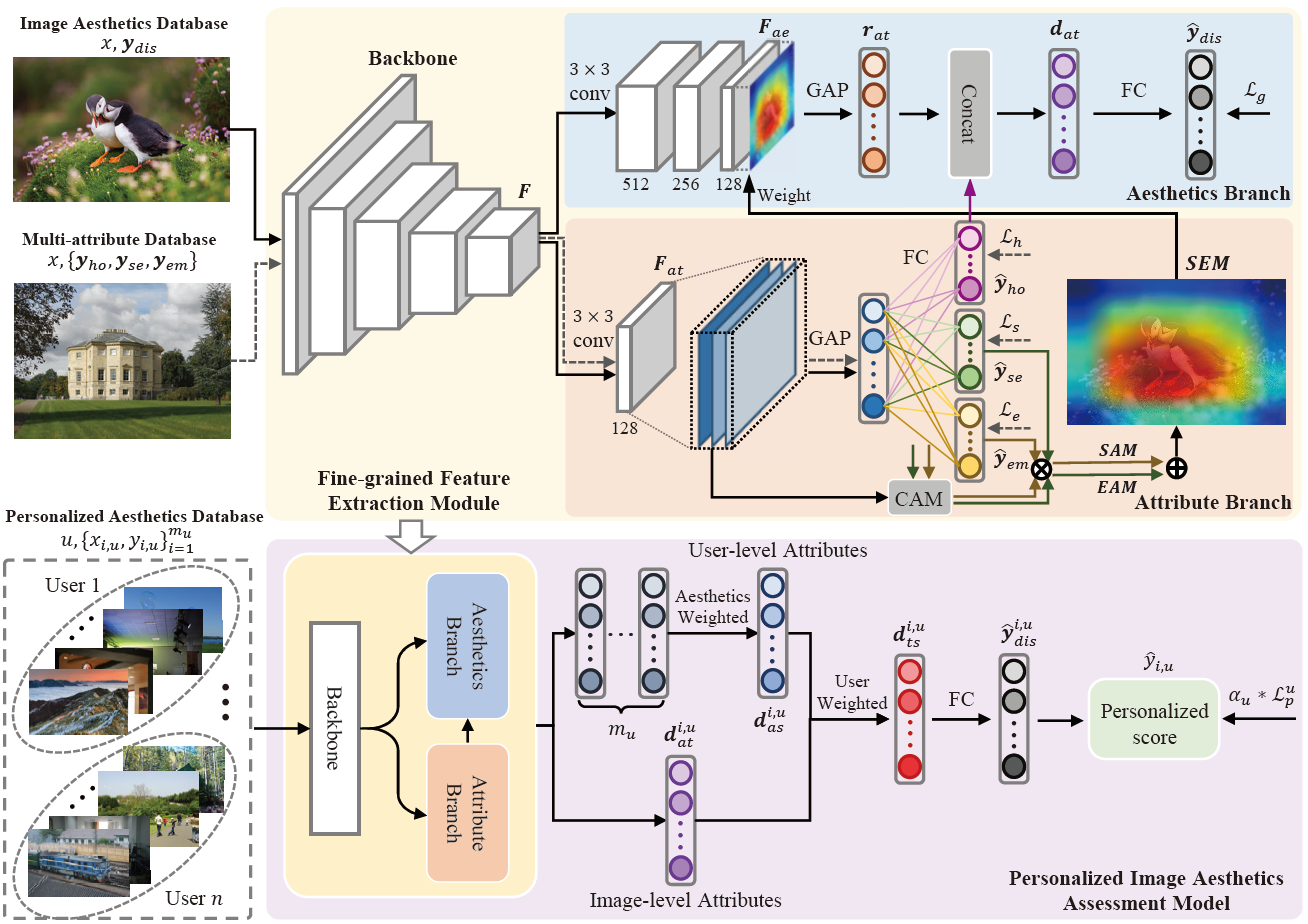
We first build a fine-grained feature extraction (FFE) module to obtain the refined local features of image attributes to compensate for holistic features. The FFE module is then used to generate user-level features, which are combined with the image-level features to obtain user-preferred fine-grained feature representations. By training extensive PIAA tasks, the aesthetic distribution of most users can be transferred to the personalized scores of individual users. To enable our proposed model to learn more generalizable aesthetics among individual users, we incorporate the degree of dispersion between personalized scores and image aesthetic distribution as a coefficient in the loss function during model training.
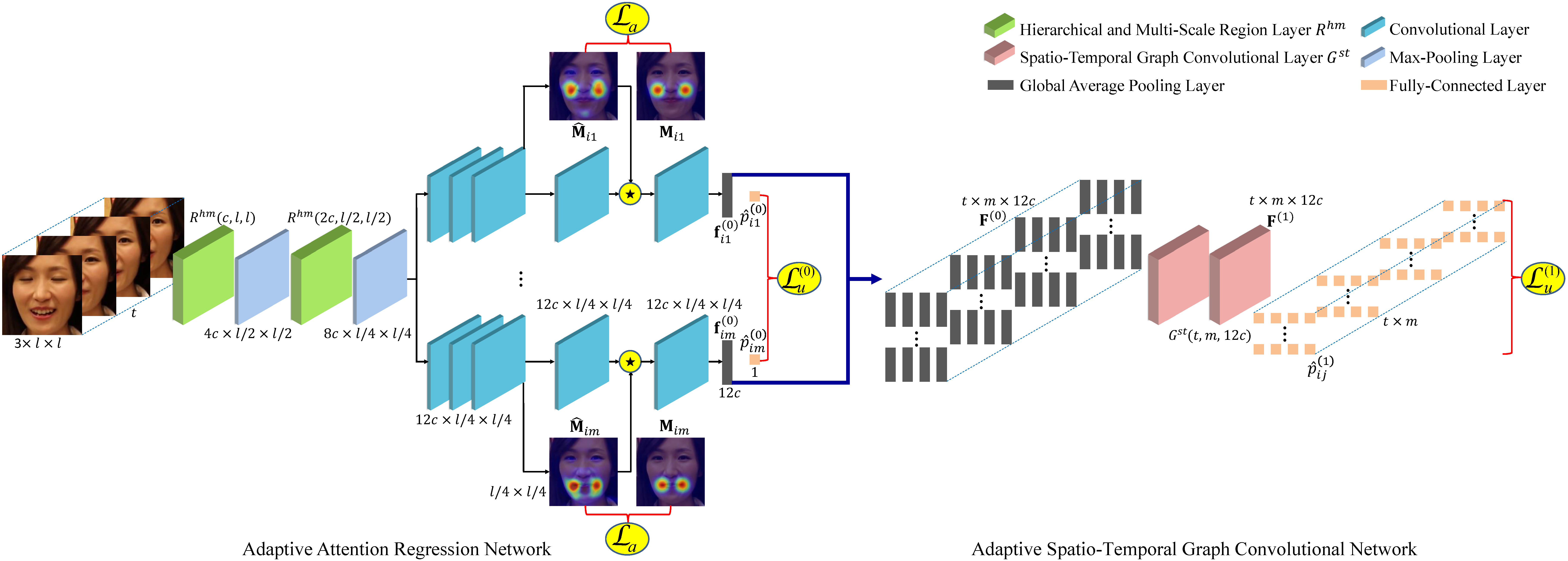
We propose a novel adaptive attention and relation (AAR) framework for facial AU detection. Specifically, we propose an adaptive attention regression network to regress the global attention map of each AU under the constraint of attention predefinition and the guidance of AU detection, which is beneficial for capturing both specified dependencies by landmarks in strongly correlated regions and facial globally distributed dependencies in weakly correlated regions. Moreover, considering the diversity and dynamics of AUs, we propose an adaptive spatio-temporal graph convolutional network to simultaneously reason the independent pattern of each AU, the inter-dependencies among AUs, as well as the temporal dependencies.
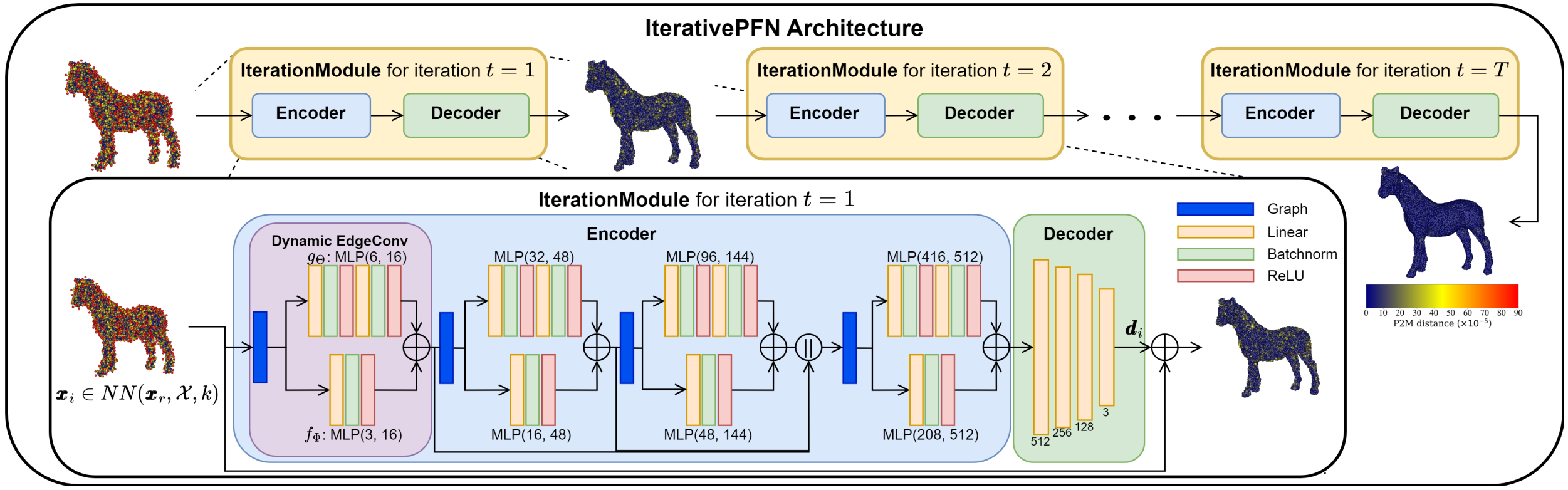
We propose IterativePFN (iterative point cloud filtering network), which consists of multiple IterationModules that model the true iterative filtering process internally, within a single network. We train our IterativePFN network using a novel loss function that utilizes an adaptive ground truth target at each iteration to capture the relationship between intermediate filtering results during training. This ensures filtered results converge faster to the clean surfaces.
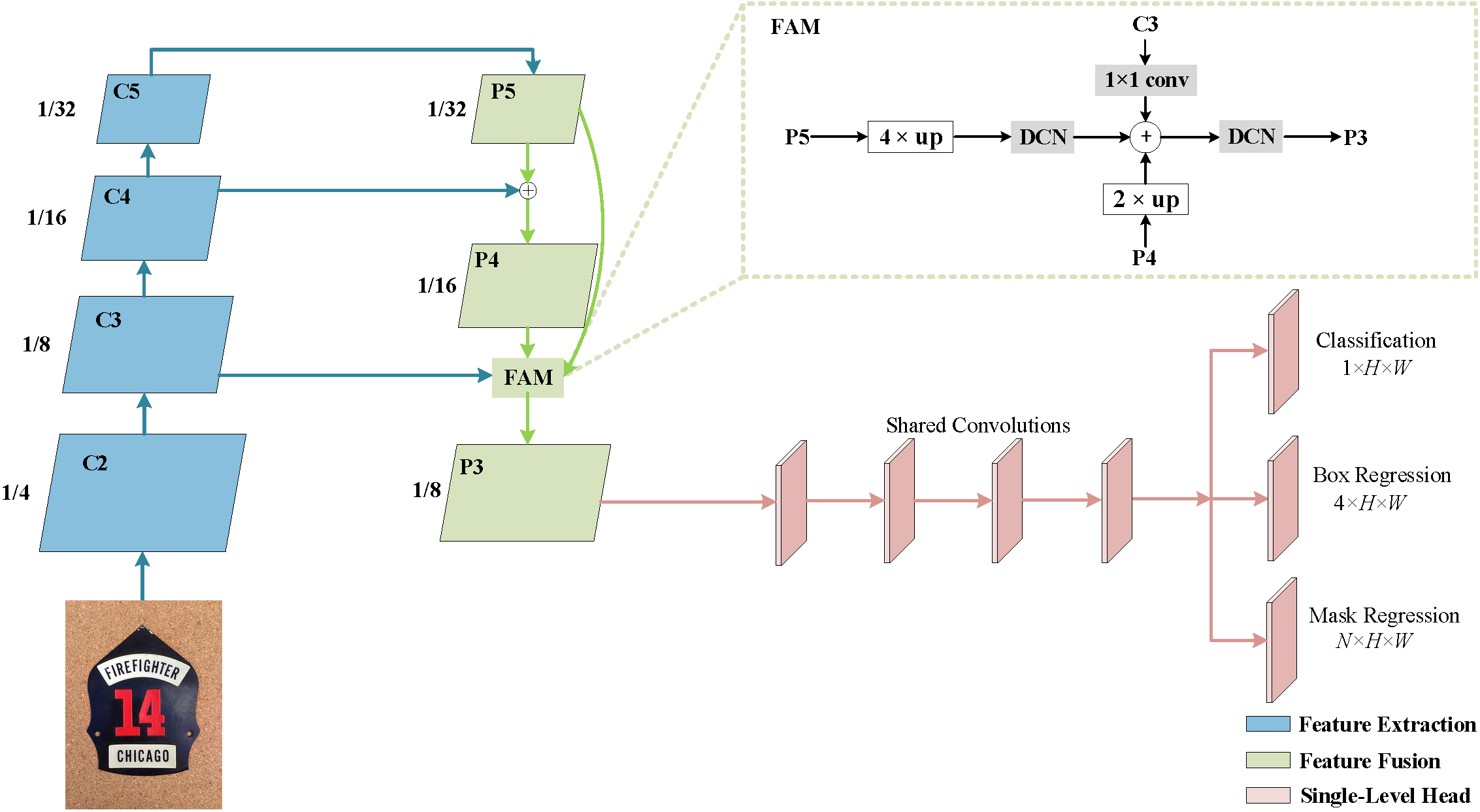
We propose a novel light-weight anchor-free text detection framework called TextDCT, which adopts the discrete cosine transform (DCT) to encode the text masks as compact vectors. Further, considering the imbalanced number of training samples among pyramid layers, we only employ a single-level head for top-down prediction. To model the multi-scale texts in a single-level head, we introduce a novel positive sampling strategy by treating the shrunk text region as positive samples, and design a feature awareness module (FAM) for spatial-awareness and scale-awareness by fusing rich contextual information and focusing on more significant features. Moreover, we propose a segmented non-maximum suppression (S-NMS) method that can filter low-quality mask regressions.
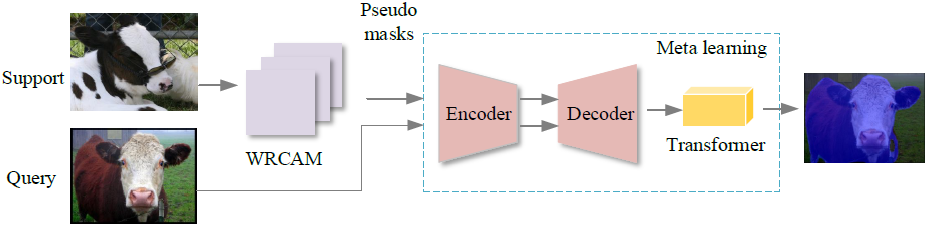
We propose a weakly supervised few-shot semantic segmentation model based on the meta learning framework, which utilizes prior knowledge and adjusts itself according to new tasks. Thereupon then, the proposed network is capable of both high efficiency and generalization ability to new tasks. In the pseudo mask generation stage, we develop a WRCAM method with the channel-spatial attention mechanism to refine the coverage size of targets in pseudo masks. In the few-shot semantic segmentation stage, the optimization based meta learning method is used to realize few-shot semantic segmentation by virtue of the refined pseudo masks.
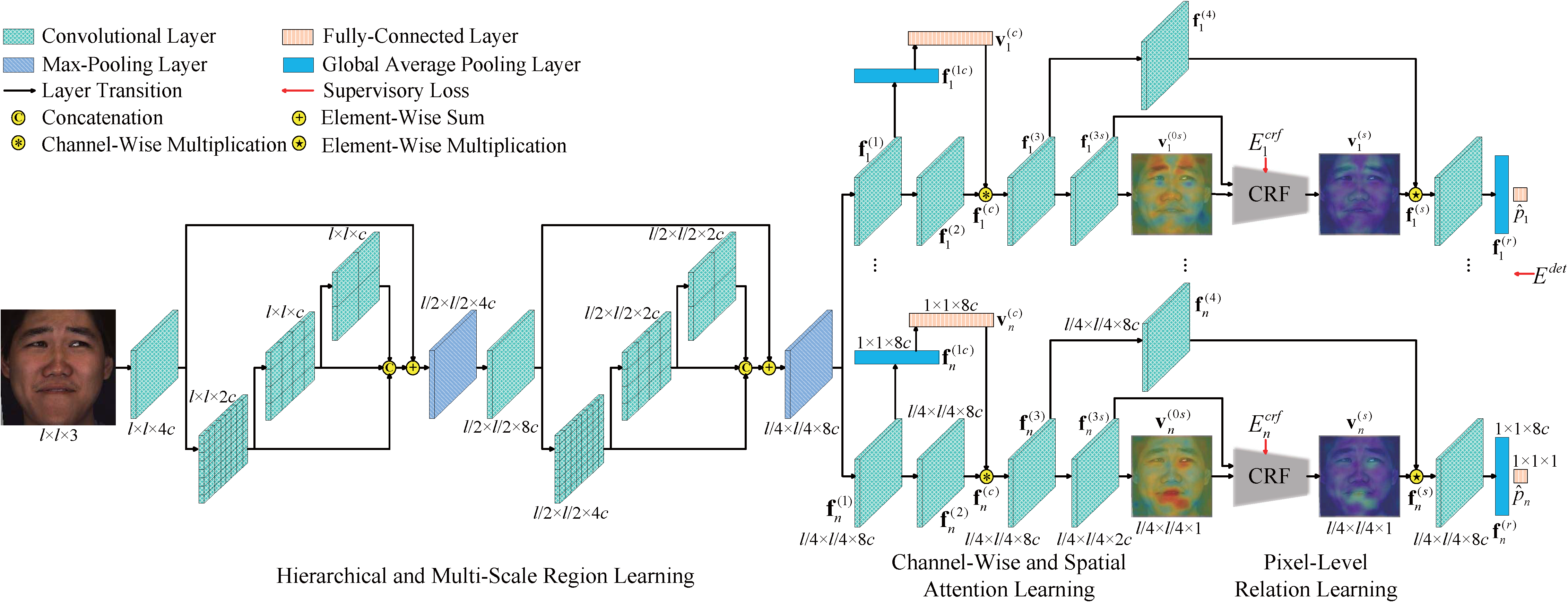
We propose an end-to-end deep learning based attention and relation learning framework for AU detection with only AU labels, which has not been explored before. In particular, multi-scale features shared by each AU are learned firstly, and then both channel-wise and spatial attentions are adaptively learned to select and extract AU-related local features. Moreover, pixel-level relations for AUs are further captured to refine spatial attentions so as to extract more relevant local features. Without changing the network architecture, our framework can be easily extended for AU intensity estimation.
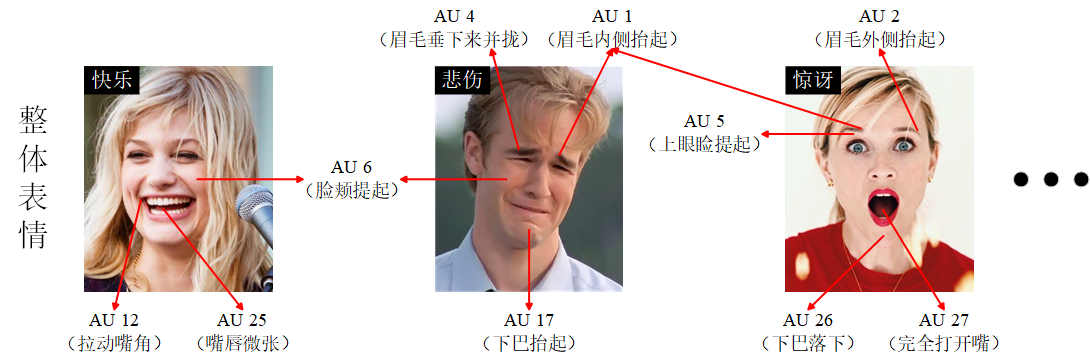
Expression action unit (AU) recognition based on deep learning is a hot topic in the fields of computer vision and affective computing. Each AU describes a facial local expression action, and the combinations of AUs can quantitatively represent any expression. Current AU recognition mainly faces three challenging factors: scarcity of labels, difficulty of feature capture, and imbalance of labels. On the basis of this, this paper categorizes the existing researches into transfer learning based, region learning based, and relation learning based methods, and comments and summarizes each category of representative methods. Finally, this paper compares and analyzes different methods, and further discusses the future research directions of AU recognition.
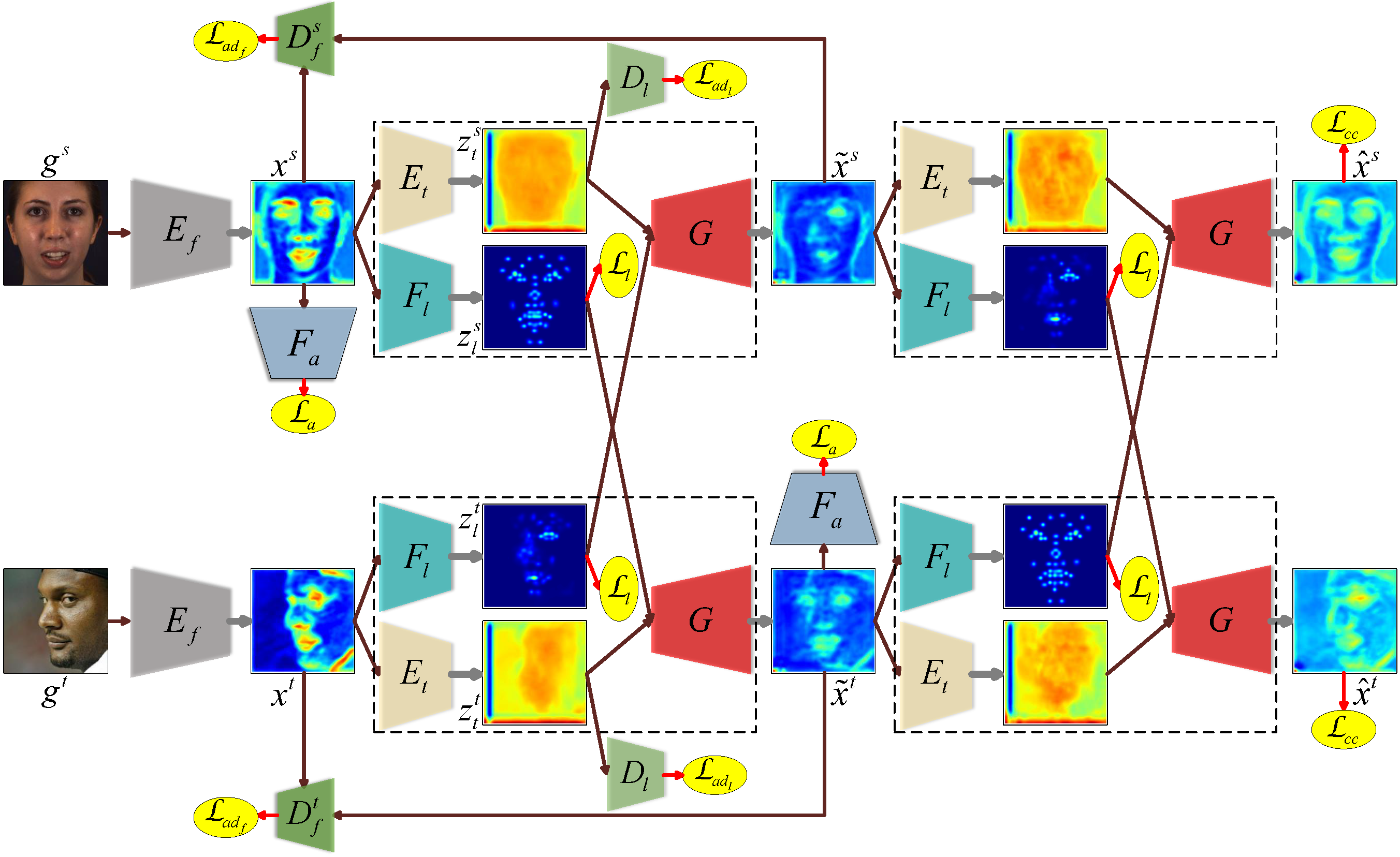
We propose an end-to-end unconstrained facial AU detection framework based on domain adaptation, which transfers accurate AU labels from a constrained source domain to an unconstrained target domain by exploiting labels of AU-related facial landmarks. Specifically, we map a source image with label and a target image without label into a latent feature domain by combining source landmark-related feature with target landmark-free feature. Due to the combination of source AU-related information and target AU-free information, the latent feature domain with transferred source label can be learned by maximizing the target-domain AU detection performance. Moreover, we introduce a novel landmark adversarial loss to disentangle the landmark-free feature from the landmark-related feature by treating the adversarial learning as a multi-player minimax game.
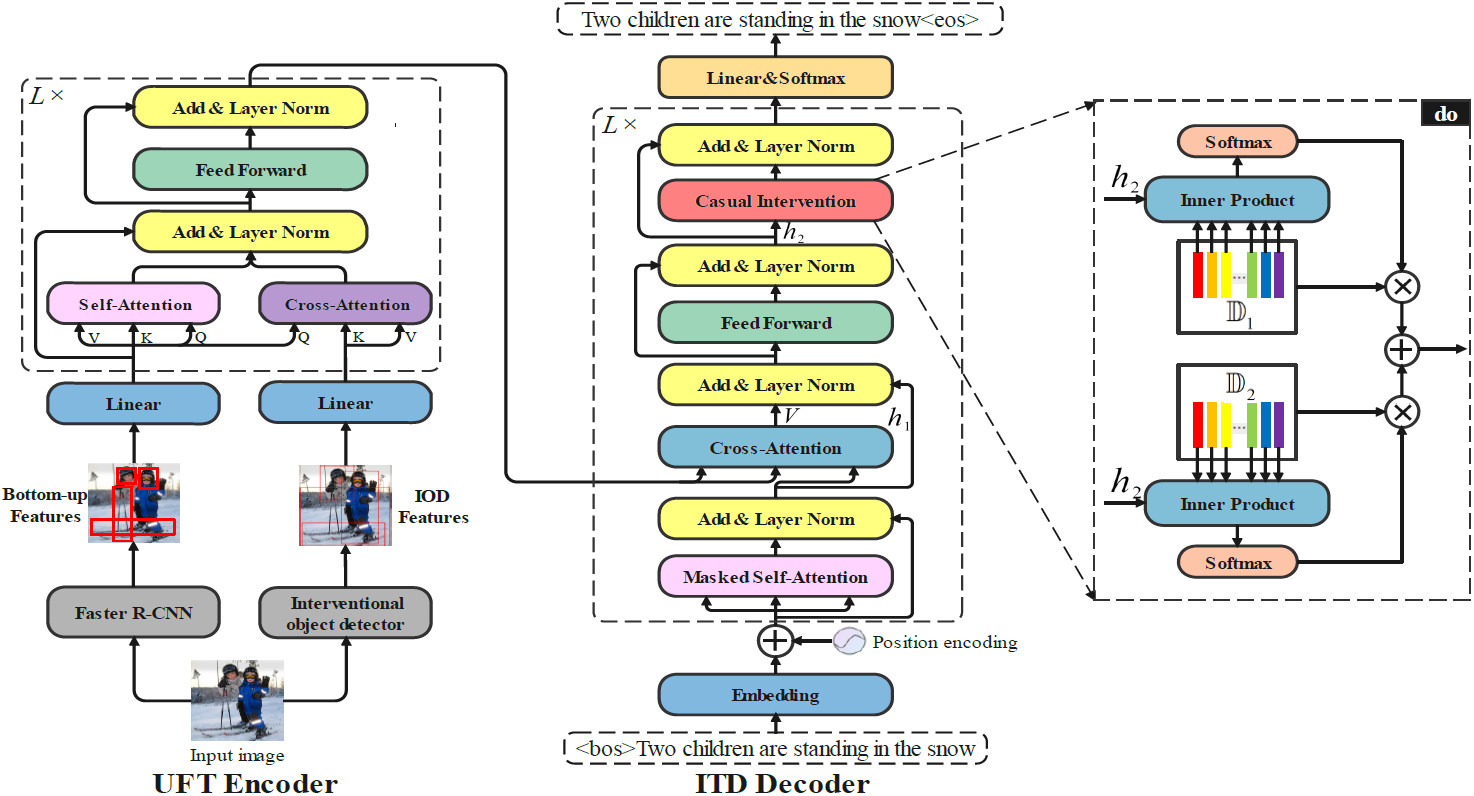
We first use Structural Causal Models (SCMs) to show how two confounders damage the image captioning. Then we apply the backdoor adjustment to propose a novel causal inference based image captioning (CIIC) framework, which consists of an interventional object detector (IOD) and an interventional transformer decoder (ITD) to jointly confront both confounders. In the encoding stage, the IOD is able to disentangle the region-based visual features by deconfounding the visual confounder. In the decoding stage, the ITD introduces causal intervention into the transform decoder and deconfounds the visual and linguistic confounders simultaneously. Two modules collaborate with each other to eliminate the spurious correlations caused by the unobserved confounders.
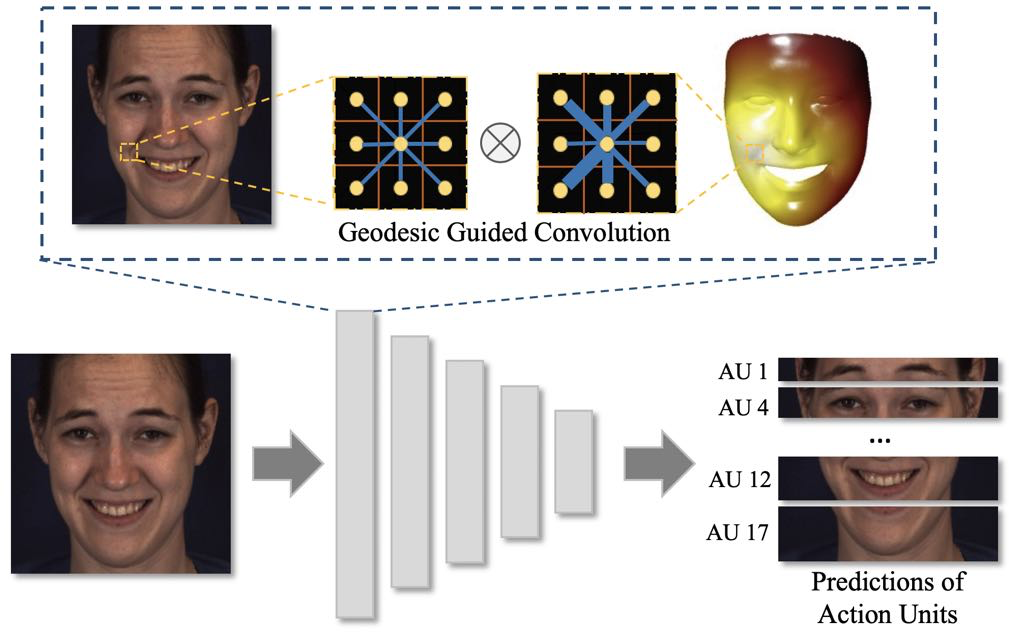
We propose a novel geodesic guided convolution (GeoConv) for AU recognition by embedding 3D manifold information into 2D convolutions. Specifically, the kernel of GeoConv is weighted by our introduced geodesic weights, which are negatively correlated to geodesic distances on a coarsely reconstructed 3D morphable face model. Moreover, based on GeoConv, we further develop an end-to-end trainable framework named GeoCNN for AU recognition.
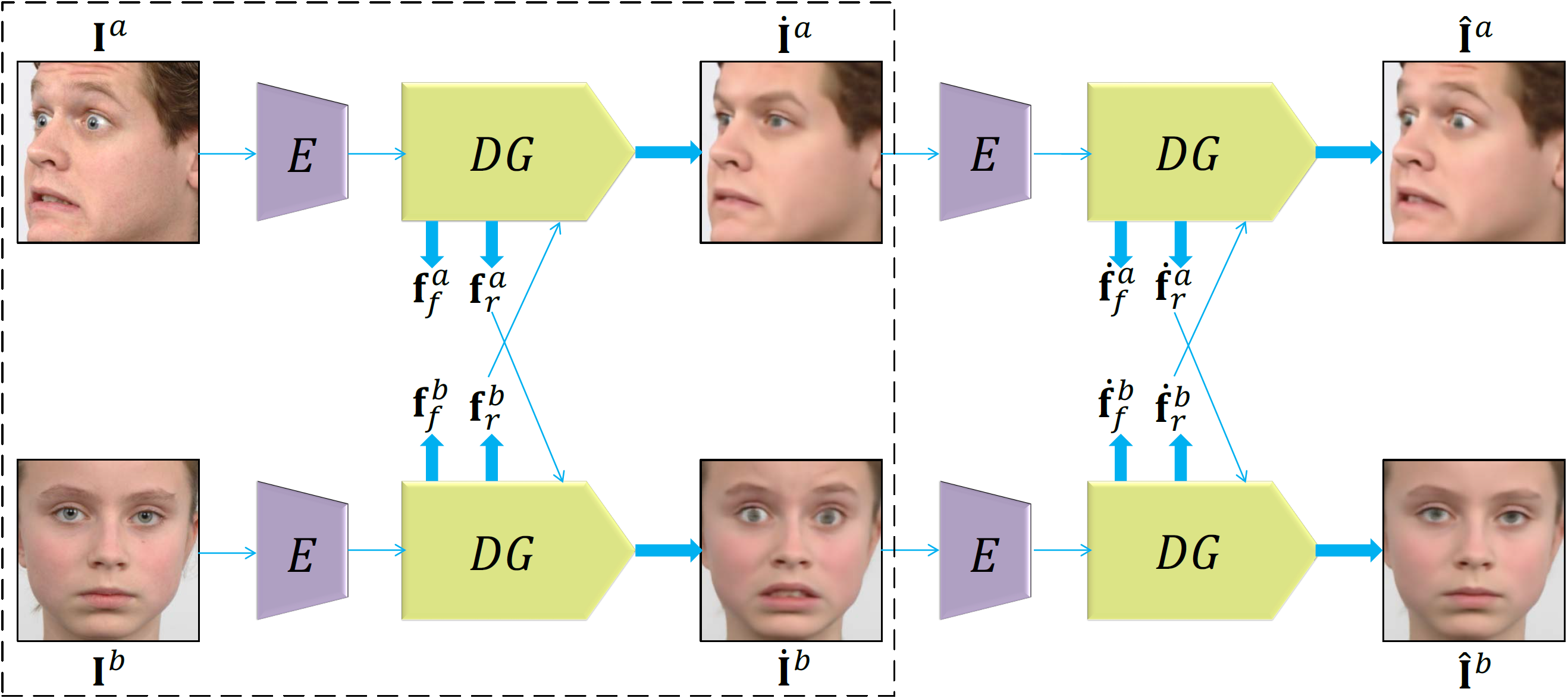
We propose to explicitly transfer facial expression by directly mapping two unpaired input images to two synthesized images with swapped expressions. Specifically, considering AUs semantically describe fine-grained expression details, we propose a novel multi-class adversarial training method to disentangle input images into two types of fine-grained representations: AU-related feature and AU-free feature. Then, we can synthesize new images with preserved identities and swapped expressions by combining AU-free features with swapped AU-related features. Moreover, to obtain reliable expression transfer results of the unpaired input, we introduce a swap consistency loss to make the synthesized images and self-reconstructed images indistinguishable.
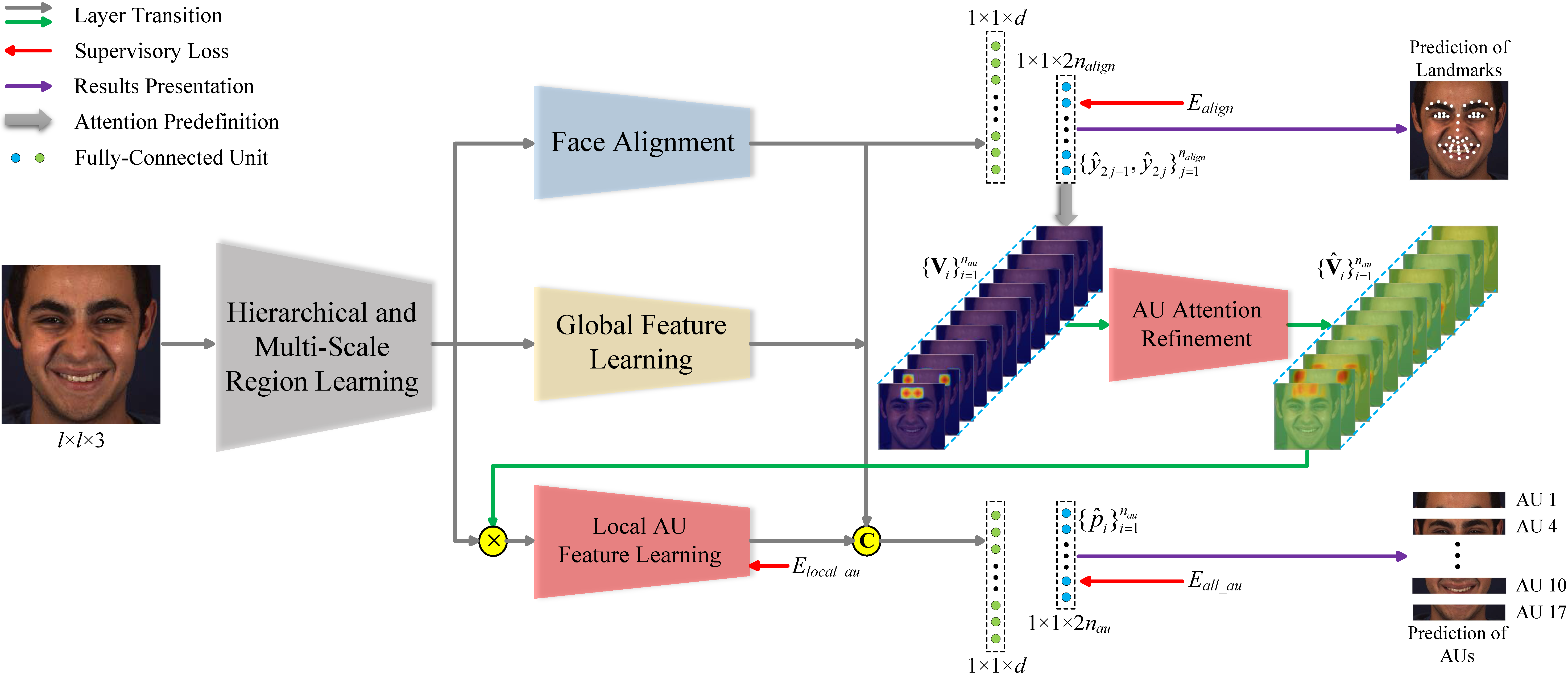
We propose a novel end-to-end deep learning framework for joint AU detection and face alignment, which has not been explored before. In particular, multi-scale shared feature is learned firstly, and high-level feature of face alignment is fed into AU detection. Moreover, to extract precise local features, we propose an adaptive attention learning module to refine the attention map of each AU adaptively. Finally, the assembled local features are integrated with face alignment feature and global feature for AU detection.
Granted Patents
2023: A Method and Device of Facial Action Unit Recognition Based on Adaptive Attention and Spatio-Temporal Correlation, The First Inventor, ZL202210606040.5
2022: A Method and Device of User Personality Characteristic Prediction Based on Multi-Modal Information Fusion, The Third Inventor, ZL202111079044.4
2021: A Method and Device of Facial Action Unit Recognition Based on Joint Learning and Optical Flow Estimation, The First Inventor, ZL202110360938.4
2018: Identity Verification System V1.0 Based on Face Recognition, Software Copyright, The Second Inventor, 2018SR160441
Awards
2023: Excellent Thesis Advisor of the China University of Mining and Technology
2022: Honorable Mention for Teaching Competition at the School of Computer Science and Technology, China University of Mining and Technology
2021: Excellent Headteacher of the China University of Mining and Technology
2020: Outstanding Prize for Scientific and Technological Progress of Shanghai Municipality, 11/18
2020: One of the Top 10 Scientific Advances in the Shanghai Jiao Tong University, 8/9
2019: Super AI Leader (SAIL) TOP 30 project at World Artificial Intelligence Conference, 6/13
2016-2019: KoGuan Endeavor Scholarship, Suzhou Yucai Scholarship
2015: Outstanding Graduate of the Northwestern Polytechnical University
2012-2015: Outstanding Student of the Northwestern Polytechnical University
2012-2015: National Endeavor Scholarship, Samsung China Scholarship, Wu Yajun Scholarship
Teaching Experiences
2023 - Present: Introduction to Information Science, Lecturer
2022 - Present: Image Processing and Computer Vision, Lecturer (Principal of Course)
2022 - Present: Practice for Python Programming, Lecturer
2021: Computational Thinking and Artificial Intelligence Foundation, Teaching Assistant
2020: Practice for Computational Thinking and Artificial Intelligence Foundation, Lecturer
2020: Technology of Cloud Computing and Big Data, Teaching Assistant
2020: Introduction to Information Science, Teaching Assistant
Academic Services
Area Chair: ACM International Conference on Multimedia (MM) 2024
Publication Chair: Computer Graphics International (CGI) 2023
Session Chair: Computer Graphics International (CGI) 2022, Shanghai Cross-Media Intelligence and Computer Vision Forum 2019
Member in Chinese Association for Artificial Intelligence (CAAI): Professional Committee of Pattern Recognition, Professional Committee of Knowledge Engineering and Distributed Intelligence, Professional Committee of Machine Learning
Member in China Society of Image and Graphics (CSIG): Professional Committee of Machine Vision, Professional Committee of Animation and Digital Entertainment
Member in JiangSu association of Artificial Intelligence (JSAI): Professional Committee of Pattern Recognition, Professional Committee of Uncertain Artificial Intelligence
Program Committee Member/Conference Reviewer: CVPR, ICCV, IJCAI, AAAI, ACM MM, CGI, ICONIP, ICIG, NCIIP
Journal Reviewer: IEEE TPAMI, IJCV, IEEE TIP, IEEE TAFFC, IEEE TMM, Signal Processing, SPIC, IET IP, TVC, Computers & Graphics, IEEE Sensors, Journal of Electronic Imaging, Frontiers in Computer Science